Quantum density estimation with density matrices: Application to quantum anomaly detection
PHYSICAL REVIEW A(2024)
摘要
Density estimation is a central task in statistics and machine learning. This problem aims to determine the underlying probability density function that best aligns with an observed dataset. Some of its applications include statistical inference, unsupervised learning, and anomaly detection. Despite its relevance, few works have explored the application of quantum computing to density estimation. In this article, we present a quantumclassical density-matrix density estimation model, called Q-DEMDE, based on the expected values of density matrices and a quantum embedding called quantum Fourier features. The method uses quantum hardware to build probability distributions of training data via mixed quantum states. As a core subroutine, we propose an algorithm to estimate the expected value of a mixed density matrix from its spectral decomposition on a quantum computer. In addition, we present an application of the method for quantum-classical anomaly detection. We evaluated the density estimation model with quantum random and quantum adaptive Fourier features on different datasets on a quantum simulator and a real quantum computer. An important result of this work is to show that it is possible to perform density estimation and anomaly detection with high performance on present-day quantum computers.
更多查看译文
AI 理解论文
溯源树
样例
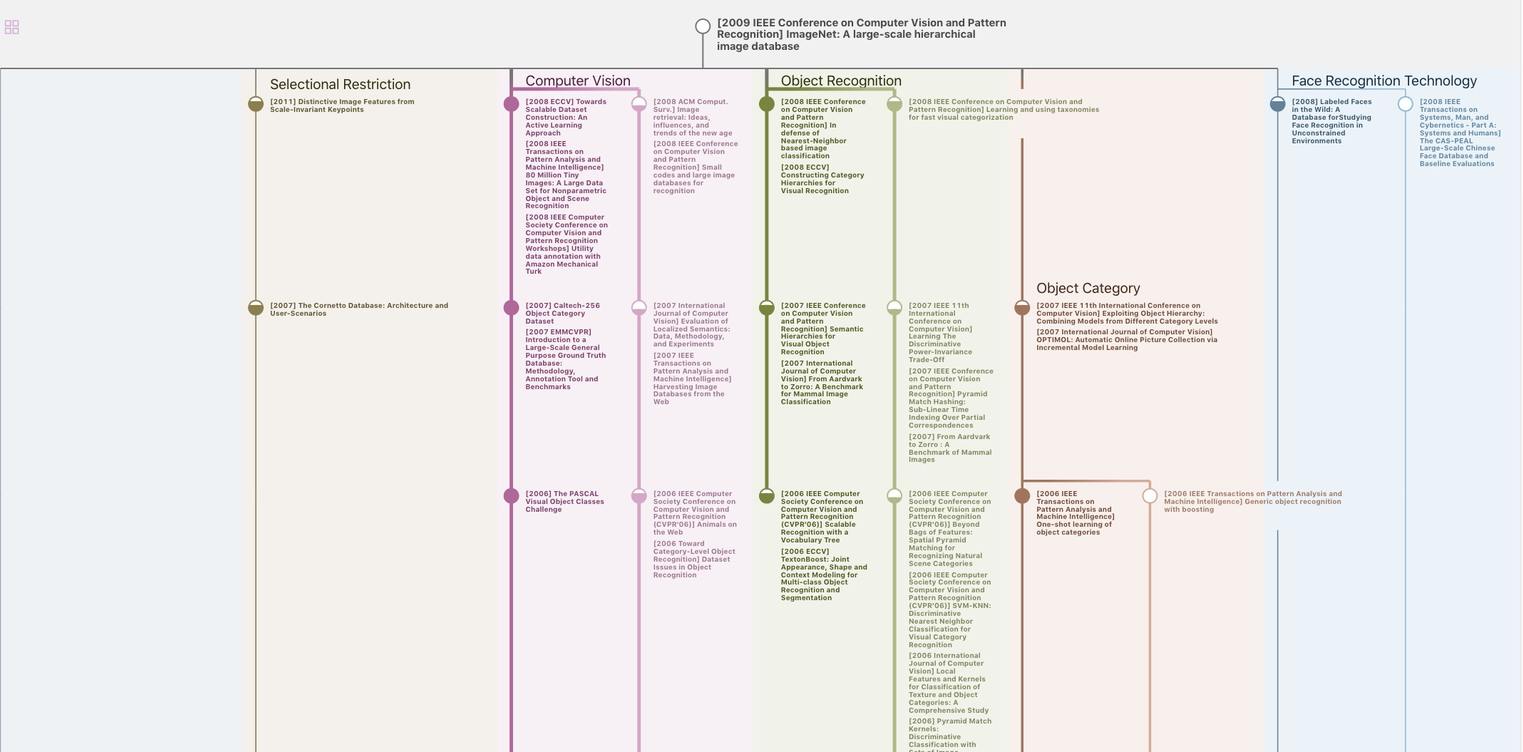
生成溯源树,研究论文发展脉络
Chat Paper
正在生成论文摘要