Dynamic Curriculum Learning with Co-training for Medical Dialogue Generation
BIBM(2021)
摘要
The purpose of medical dialogue generation is to provide automatic and accurate responses to help doctors provide diagnosis and treatment advice in an efficient w ay. However, due to the subjectivity and open-ended nature of human conversations, the complexity of training dataset varies greatly. Some methods try to solve this problem by using curriculum learning that organizes training data from easy to hard and optimizes the model with an “easy-to-difficult” scheme, but they ignore the intrinsic nature of conversation dataset that has different categories. To solve this problem, in this article, we learn a Dynamic Curriculum with Co-training (DCC) for generative dialogue systems considering both the quality and category of the data. Under our framework, we first t rain two models (query generation model and reply generation model) from dual view by leveraging end-to-end deep Gaussian Mixture Variational Autoencoders (GMVAE) architectures that combine generative and unsupervised clustering tasks together. Then we promote the clustering performance via an ensemble method by combining the clustering distribution of the two networks. Finally, we let the two models determine the training order for each other in each category according to their loss and category confidence dynamically through single-task and multitask curriculum learning. Evaluation results on the widely used medical dialogue generation dataset indicate that our proposed learning approach makes significant improvements compared to strong baselines. varies greatly.
更多查看译文
关键词
Medical dialogue generation,Curriculum learning,Co-training,GMVAE
AI 理解论文
溯源树
样例
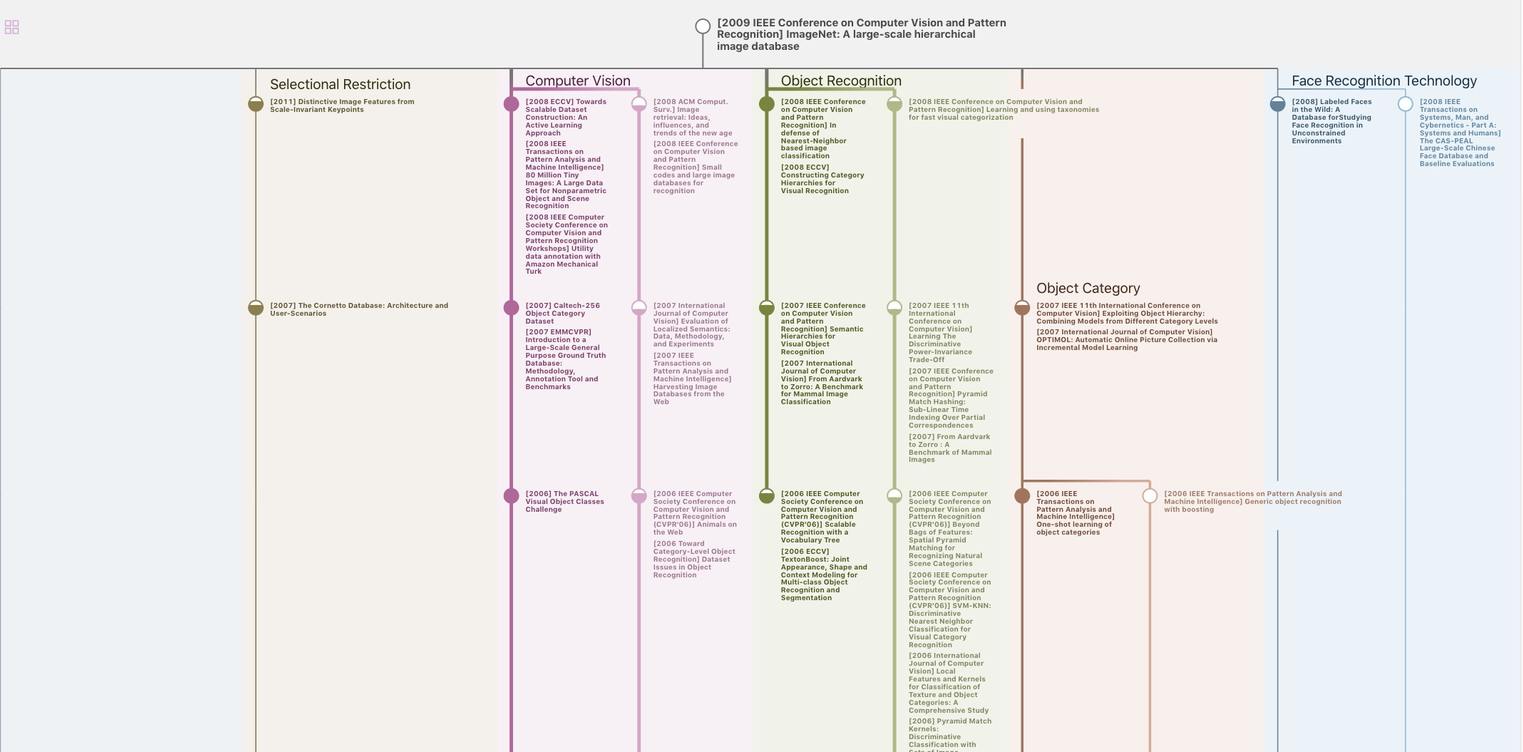
生成溯源树,研究论文发展脉络
Chat Paper
正在生成论文摘要