Enhancing Detection of SSVEPs for High-Speed Brain-Computer Interface with a Siamese Architecture
BIBM(2021)
摘要
Brain-Computer Interface (BCI) is a direct communication medium between brain and outside world. This study focuses on a Steady-State Visually Evoked Potential (SSVEP)based BCI due to its large number of instruction set. However, it is still challenging to decode multi-class SSVEPs. To improve target identification accuracy, we propose a Siamese Correlation Analysis model (SiamCA), which involves two feature extractors with tied parameters and a top decision network. We consider two datasets for benchmarking the performance of the proposed model and compare it with FBCCA, TRCA, ensemble-TRCA and a deep learning method named ConvCA. The proposed method realize a significantly higher average classification accuracy than the compared method at different data length (0.2–1.0s) on two datasets. This suggests that the proposed SiamCA model is a promising methodology for target identification of SSVEPs and could further improve the performance of SSVEP-based BCI system.
更多查看译文
关键词
Electroencephalo-gram (EEG),brain-computer interface (BCI),steady-state visual evoked potential (SSVEP),deep learning
AI 理解论文
溯源树
样例
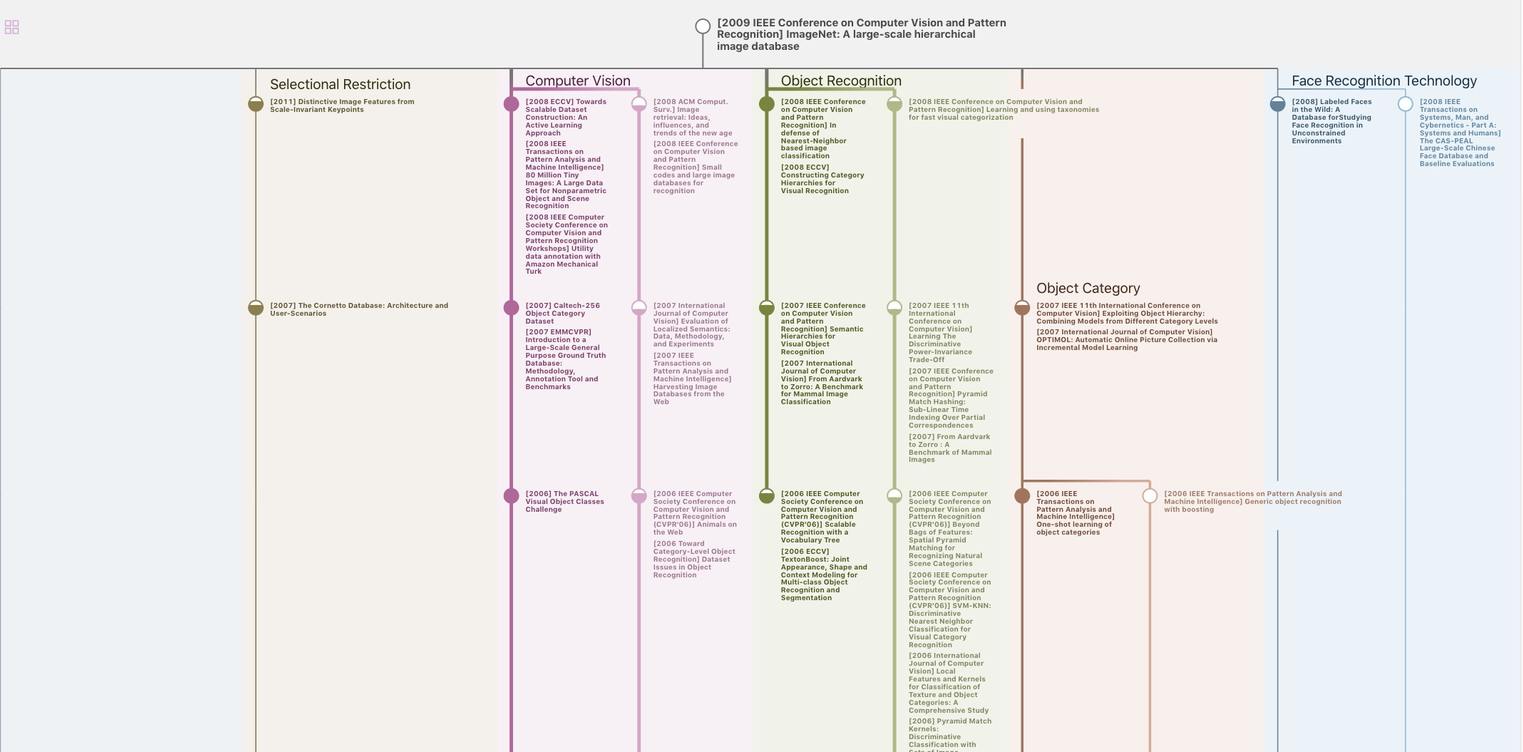
生成溯源树,研究论文发展脉络
Chat Paper
正在生成论文摘要