Multi-scale Hierarchical Transformer structure for 3D medical image segmentation.
BIBM(2021)
摘要
Transformers have demonstrated great potential in computer vision tasks which is benefited from its ability of long-range dependency modeling. However, its core material multi-head self-attention (MHSA) has high computational and spacial complexity. Besides, tokens embedded with 1D position sequence are unable to represent inductive bias of locality and neighbor contextual information, which are essential for high-resolution downstream vision tasks. Thus, transformer is hard to use in image segmentation tasks, especially in 3D medical images. In this paper, we propose a novel multi-scale hierarchical framework (MSHT) that efficiently incorporates the local modeling ability of CNN and long-range modeling ability of transformer for accurate 3D medical image segmentation. Unlike many prior Transformer-based solutions, the proposed MSHT first adopts parallel CNN and transformer as encoder block to extract the global and local feature representations. As the core component for our MSHT, a position embedding method for 3D medical image (3D IPE) is proposed to generate relational and absolute position encoding for tokens fed in transformer block. We conduct an extensive evaluation on both Lits2017 dataset and Kits2019 dataset. The results indicate that our MSHT leads to a substantial performance over other CNN-based methods on 3D image segmentation.
更多查看译文
关键词
3D medical image,image segmentation,transformer,CNN,deep learning
AI 理解论文
溯源树
样例
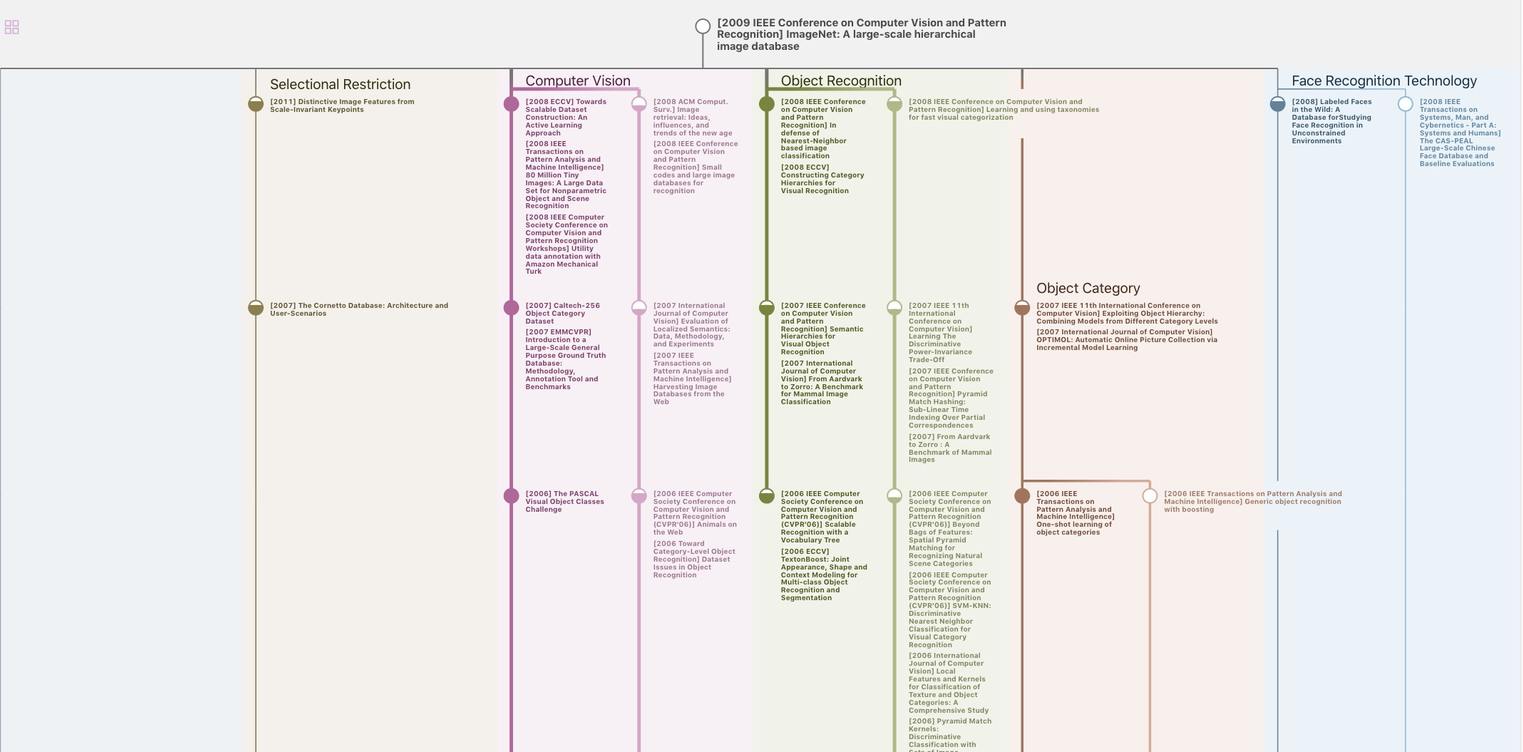
生成溯源树,研究论文发展脉络
Chat Paper
正在生成论文摘要