Classification of Single Cell Types using Small Sets of Expressed Genes - Comparative Analysis of Supervised Machine Learning Methods.
BIBM(2021)
摘要
Single cell transcriptomics measures gene expression data of large number of genes, concurrently, from tens of thousands of cells present in a studied biological sample. It is difficult to obtain good classification results due to high data dimensionality and variability of biological states. We performed a preliminary study to assess the feasibility of using supervised machine learning methods to classify peripheral blood mononuclear cell (PBMC) types from single cell gene expression data. We analyzed a large PBMC data set $(\sim 120,000$ PBMC cells), selected 47 genes (from 30698 features) suitable as SML classification features, and performed classification using 20 machine learning algorithms. Data sets represented three sample processing strategies: PBMC separation (two data sets), and experimental cell sorting by (two data sets). The accuracy in 5-class classification among 20 methods was 91-97% (PBMC separation), 97-100% (magnetic-activated cell sorting), and 82-99% (fluorescence-activated cell sorting). Our results indicate the feasibility of supervised machine learning for classification of cells into major PBMC cell types using a small number of classification features from single cell gene expression data.
更多查看译文
关键词
PBMC,10x SCT,gene expression,dimensionality reduction,transcriptome,classification,data mining,machine learning
AI 理解论文
溯源树
样例
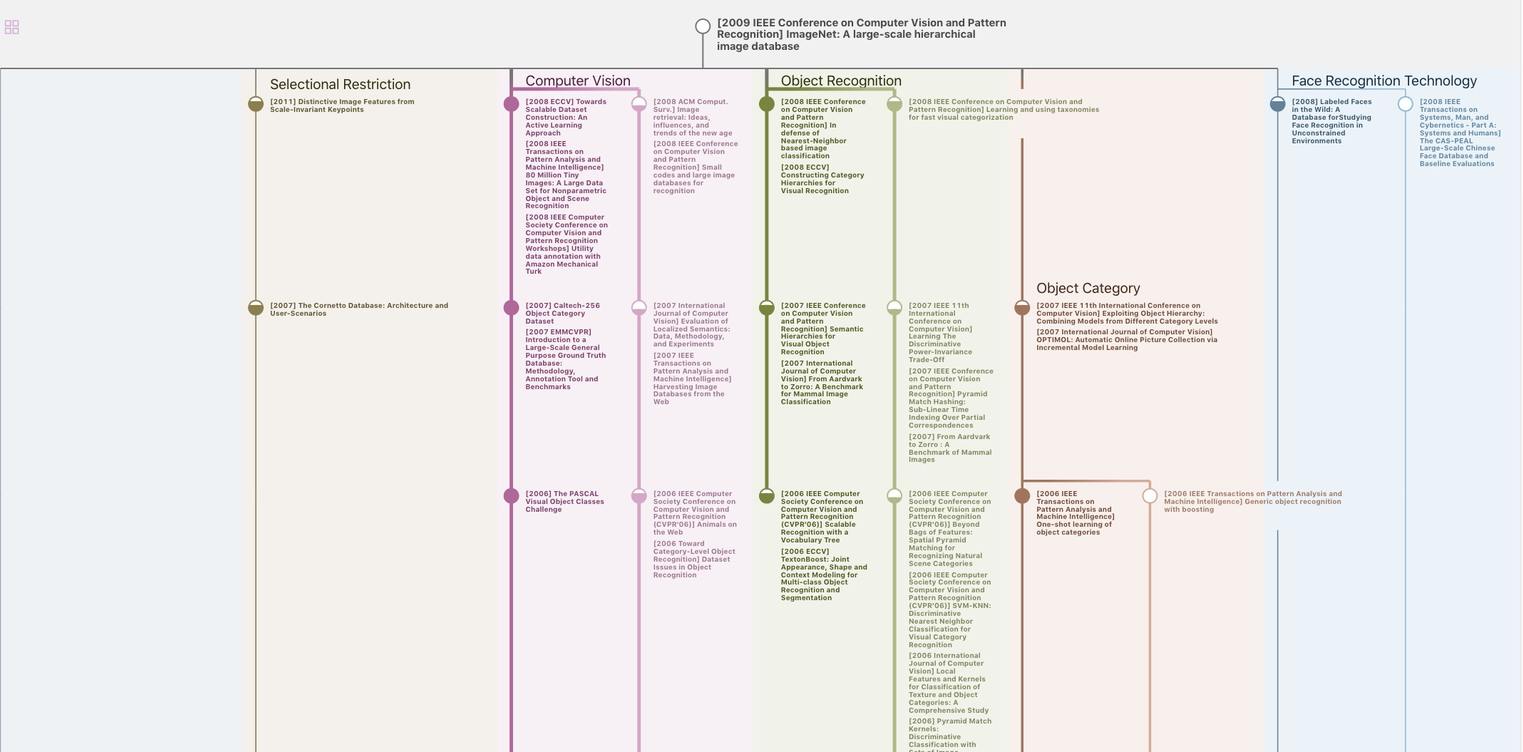
生成溯源树,研究论文发展脉络
Chat Paper
正在生成论文摘要