Identifying and Characterizing Opioid Addiction States Using Social Media Posts.
BIBM(2021)
摘要
Opioid addiction constitutes a significant contemporary health crisis that is multifarious in its complexity. Modeling the epidemiology of any addiction is challenging in its own right. For opioid addiction, the challenge is exacerbated due to the difficulties in collecting real-time data and the circumscribed nature of information opioid users may disclose owing to stigma associated with prescription misuse. Given this context, identifying the progression of individuals through the stages of (opioid) addiction is one of the more acute problems in epidemiological modeling whose solution is crucial for designing specific interventions at both personal and population levels. We describe a computational approach for determining and characterizing addiction stages of opioid users from their social media posts. The proposed approach combines recurrent neural network learning with information-theoretic analysis of word-associations and context-based word embedding to determine addiction stage-specific language usage. Users who have a high likelihood for relapsing back to drug-use are identified and characterized using propensity score matching and logistic regression. Experimental evaluations indicate that the proposed approach can distinguish between various addiction stages and identify users prone to relapse with high accuracy as evidenced by F1 scores of 0.88 and 0.79 respectively.
更多查看译文
关键词
Computational epidemiology,opioid addiction,addiction state analysis,social media
AI 理解论文
溯源树
样例
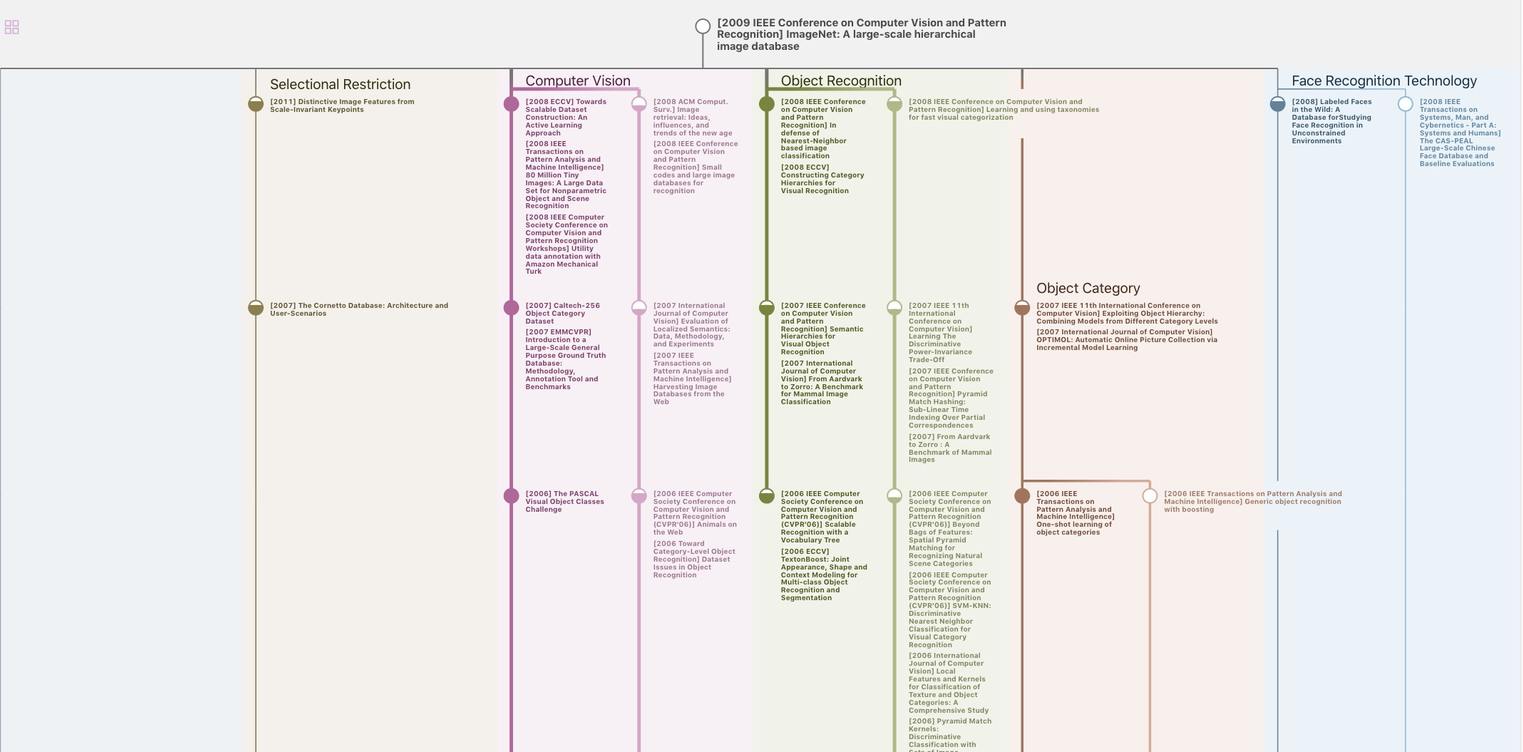
生成溯源树,研究论文发展脉络
Chat Paper
正在生成论文摘要