Multi-view Clustering for the Integration Analysis of Gene Expression and Methylation Data
BIBM(2021)
摘要
The accumulated gene expression and DNA methylation data provide a great opportunity to exploit the mechanisms of biological systems. Current algorithms for the integration of gene expression and methylation data are characterized for undesirable performance because they fail to address the latent relations in the heterogeneous data. To solve this problem, we propose a novel multi-view clustering with self-representation learning and low-rank tensor constraint (MCSL-LTC), where the gene expression and DNA methylation data are treated as complementary views, and MCSL-LTC obtains a consensus partitioning reflecting the structure and features of various views. Specifically, self-representation learning is employed to explore the low-dimensional subspace structures embedded in different views, where the tensor norm is adopted to smooth different views, therefore improving the quality of features. Experimental results demonstrate that the proposed approach outperforms state-of-the-art baselines in terms of accuracy on both the social and cancer data, provides an effective and efficient method for the integration of heterogeneous genomic data.
更多查看译文
关键词
Multi-view clustering,Self-representation learning,Low-rank tensor constraint,Gene clustering,Integrative analysis
AI 理解论文
溯源树
样例
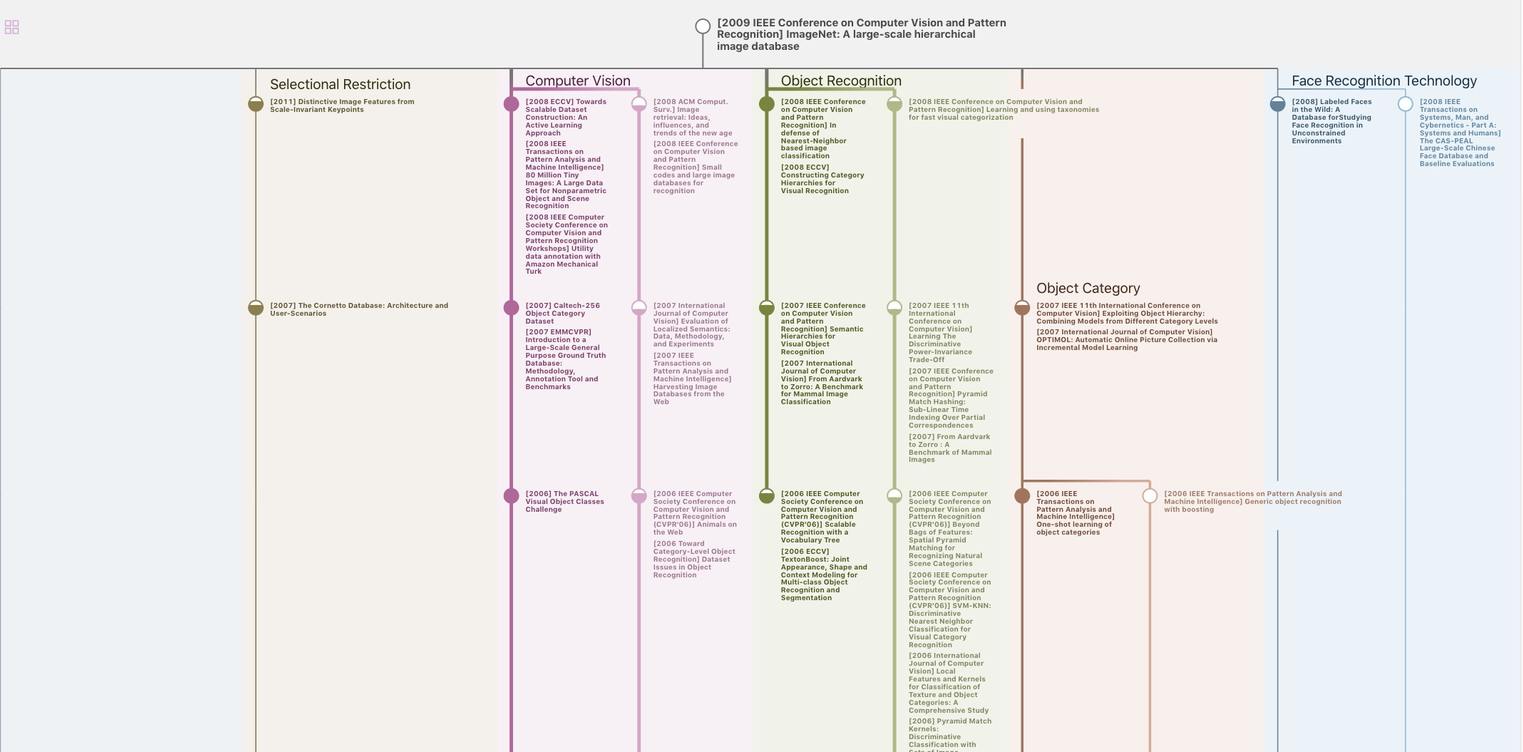
生成溯源树,研究论文发展脉络
Chat Paper
正在生成论文摘要