An Integrated Multi-Omics Approach for AMR Phenotype Prediction of Gut Microbiota.
BIBM(2021)
摘要
The gut microbiota is crucial for human physiology and susceptibility to diseases. Knowing the AMR phenotype canfacilitate the understanding of the impact of antibiotics administration on the gut microbiota. Nowadays, whole-genome sequencing for antibiotic susceptibility testing (WGS-AST) is widely used in clinical microbiology to predict the AMR phenotype. To release the limitations of the genomic information and improve the WGS-AST prediction, we propose an integrated multi-omics approach, employing a deep generative neural network (VAE: variational auto-encoder). We evaluate the proposed approach by two machine learning techniques (i.e., K-means for clustering and Random Forest for classification). Our evaluation results show that the integrated multi-omics approach achieves relatively better performance than the conventional WGS-AST. Moreover, the integrated multi-omics approach is able to visually reveal AMR phenotype of the gutmicrobiota via antibacterial spectrum. Our work provides evidence that multi-omics information is useful to enhance the WGS-AST prediction.
更多查看译文
关键词
gut microbiota,antibiotic resistance,genomics,metabolomics,machine learning
AI 理解论文
溯源树
样例
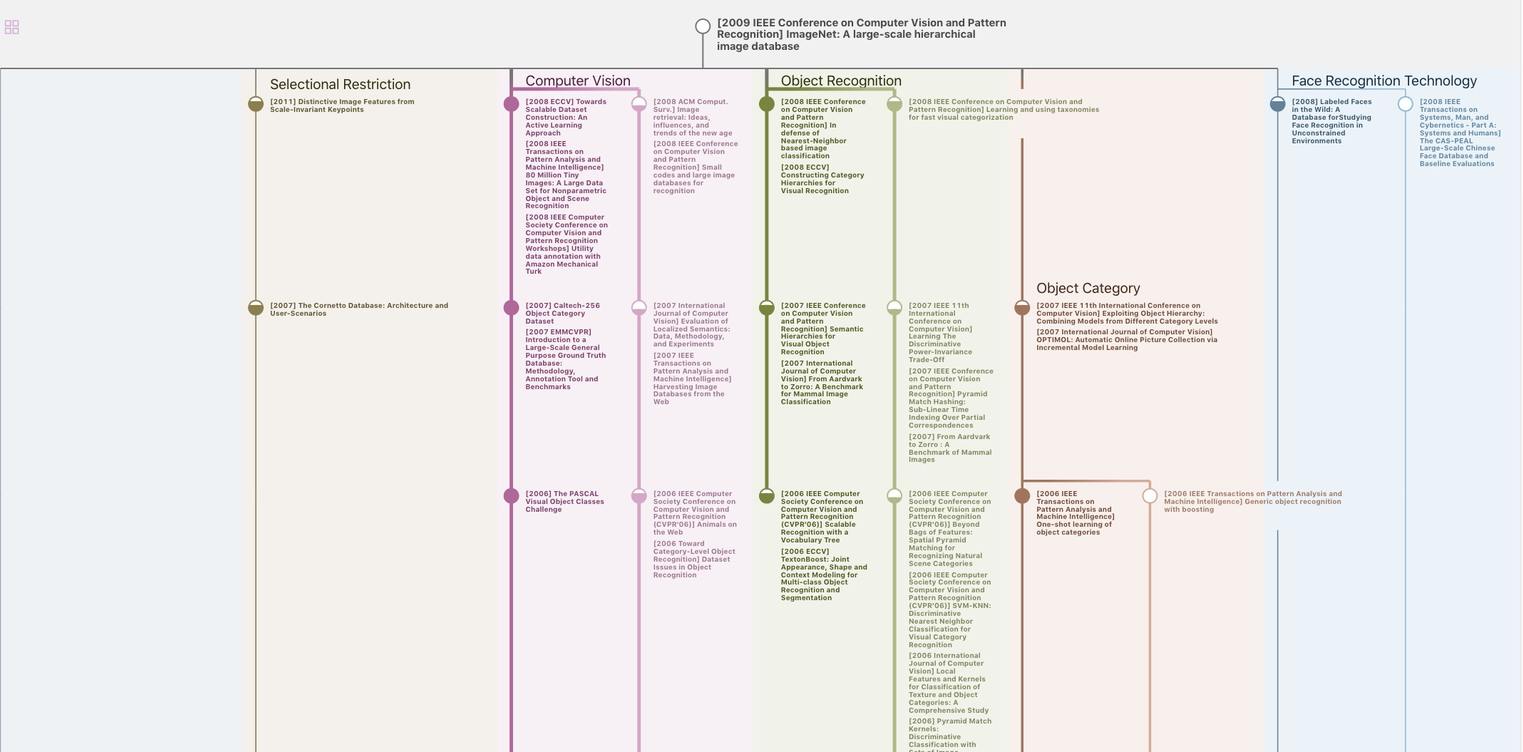
生成溯源树,研究论文发展脉络
Chat Paper
正在生成论文摘要