Hierarchical Clustering Split for Low-Bias Evaluation of Drug-Target Interaction Prediction.
BIBM(2021)
摘要
Drug-target interaction (DTI) prediction is important in drug discovery and chemogenomics studies. Machine learning, particularly deep learning, has advanced this area significantly over the past few years. However, a significant gap between the performance reported in academic papers and that in practical drug discovery settings, e.g. the random-split-based evaluation strategy tends to be too optimistic in estimating the prediction performance in real-world settings. Such performance gap is largely due to hidden data bias in experimental datasets and inappropriate data split. In this paper, we construct a low-bias DTI dataset and study more challenging data split strategies to improve performance evaluation for real-world settings. Specifically, we study the data bias in a popular DTI dataset, BindingDB, and re-evaluate the prediction performance of three state-of-the-art deep learning models using five different data split strategies: random split, cold drug split, scaffold split, and two hierarchical-clustering-based splits. In addition, we comprehensively examine six performance metrics. Our experimental results confirm the overoptimism of the popular random split and show that hierarchical-clustering-based splits are far more challenging and can provide potentially more useful assessment of model generalizability in real-world DTI prediction settings.
更多查看译文
关键词
Drug-target interaction,data bias,data splitting strategy,performance evaluation
AI 理解论文
溯源树
样例
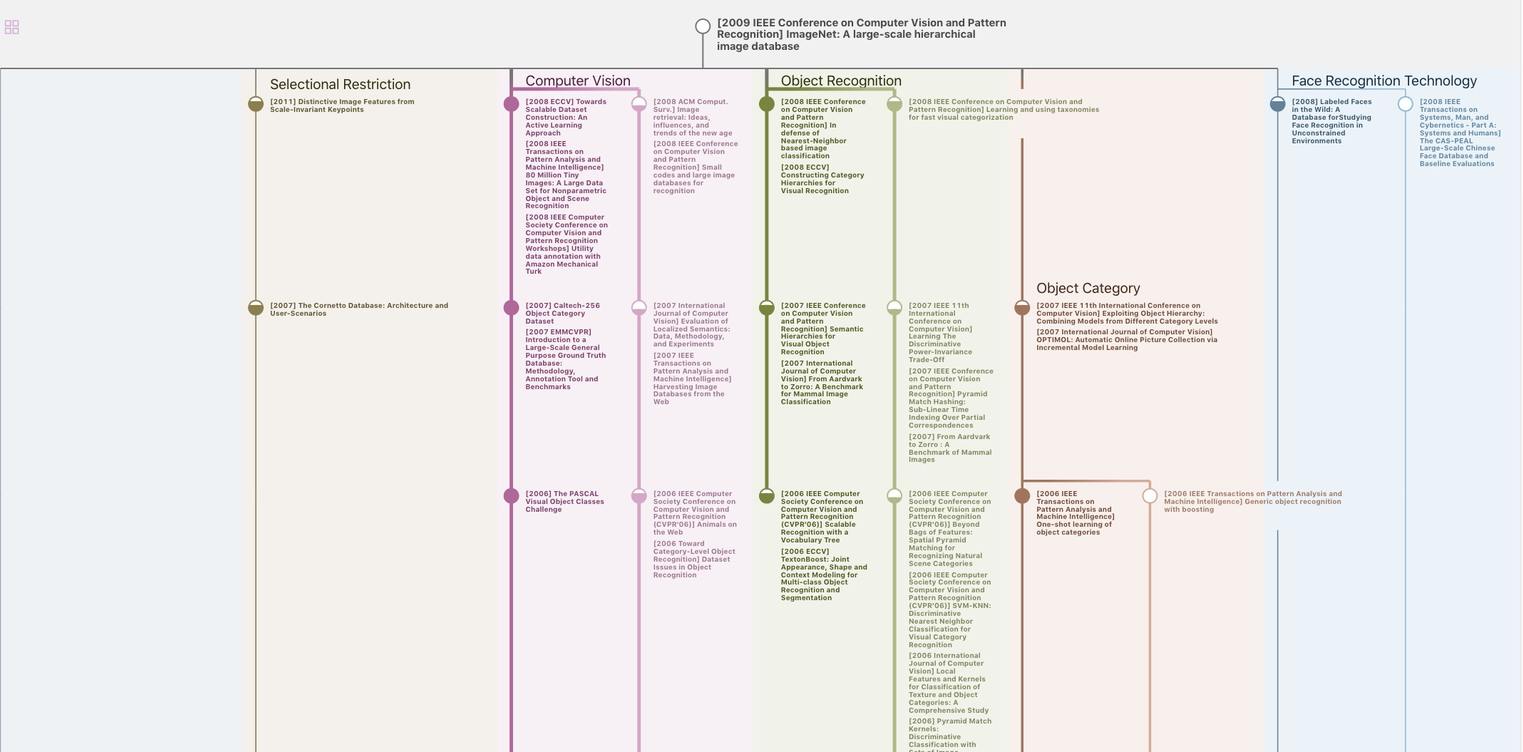
生成溯源树,研究论文发展脉络
Chat Paper
正在生成论文摘要