Controlled Breathing Effect on Respiration Quality Assessment Using Machine Learning Approaches
CinC(2021)
摘要
Thoracic bio-impedance (BioZ) measurements have been proposed as an alternative for respiratory monitoring. Given the ambulatory nature of this modality, it is more prone to noise sources. In this study, two pre-trained machine learning models were used to classify BioZ signals into clean and noisy classes. The models were trained on data from patients suffering from chronic obstructive pulmonary disease, and their performance was evaluated on data from patients undergoing bariatric surgery. Additionally, transfer learning (TL) was used to optimize the models for the new patient cohort. Lastly, the effect of different breathing patterns on the performance of the machine learning models was studied. Results showed that the models performed accurately when applying them to another patient population and their performance was improved by TL. However, different imposed respiratory frequencies were found to affect the performance of the models.
更多查看译文
关键词
noise sources,pre-trained machine learning models,BioZ signals,chronic obstructive pulmonary disease,bariatric surgery,transfer learning,patient cohort,patient population,controlled breathing effect,respiration quality assessment,machine learning approaches,thoracic bio-impedance measurements,respiratory monitoring,ambulatory nature,imposed respiratory frequencies
AI 理解论文
溯源树
样例
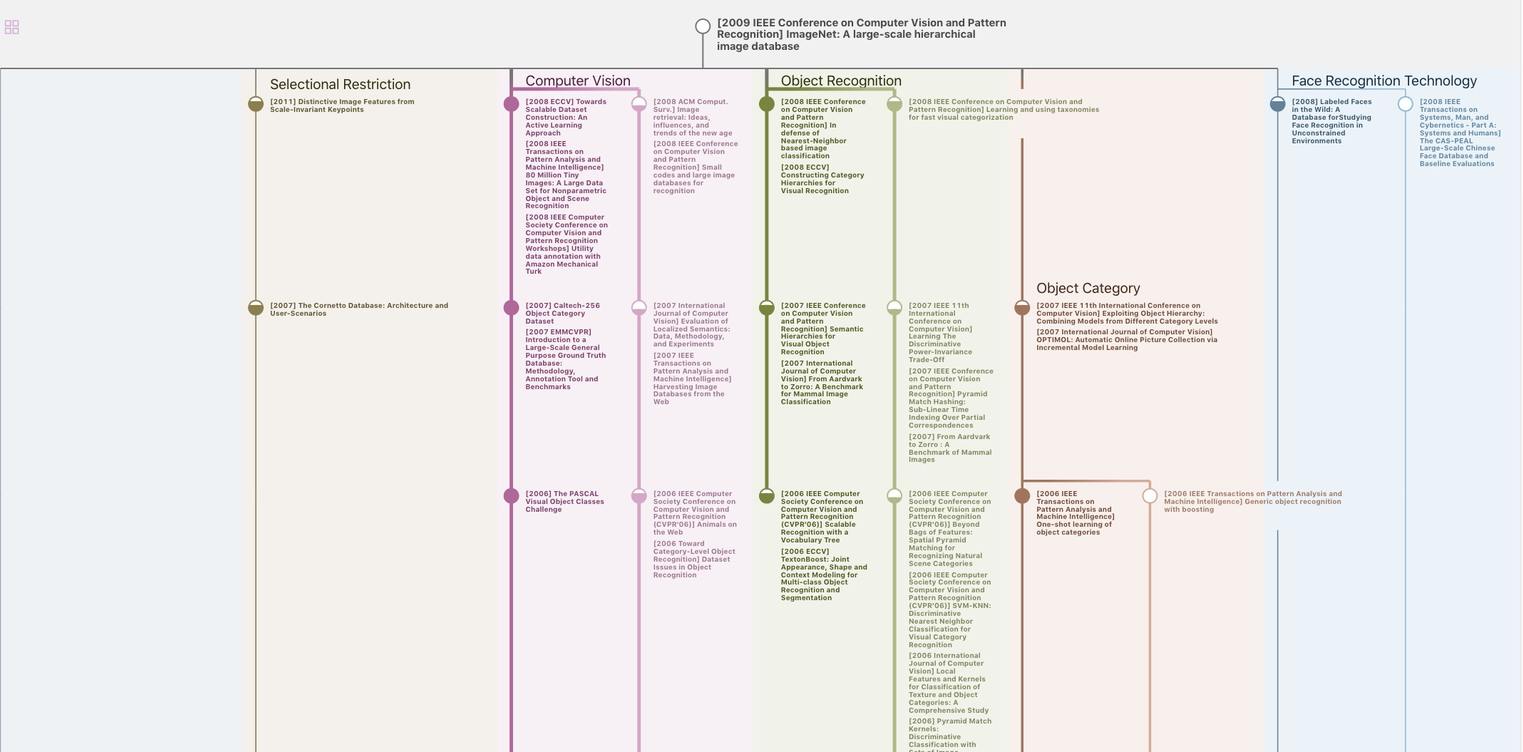
生成溯源树,研究论文发展脉络
Chat Paper
正在生成论文摘要