HMM-LSTM for Proactive Traffic Prediction in 6G Wireless Networks.
ICCT(2021)
摘要
Network traffic prediction can aid network resource allocation. To provide mobile users with better quality of service, the scheme for base station selection and handover has been explored in several studies. However, network traffic is dynamic and uncertain in the future 6G network environment, which makes the handover scheme based on deterministic network traffic performance is poor. In this paper, we propose a hybrid model based Hidden Markov Model (HMM) and Long Short Term Memory (LSTM) to predict wireless network traffic to address this issue. First, we train HMM to obtain hidden state information; then, we introduce hidden state information to the training process of LSTM to predict wireless network traffic. Simulation results show the accuracy of the hybrid HMM-LSTM model compared to traditional time series prediction models, such as Autoregressive Integral Moving Average (ARIMA) and conventional LSTM.
更多查看译文
关键词
6G,Long Short Term Memory,Hidden Markov Model,Network Traffic Prediction
AI 理解论文
溯源树
样例
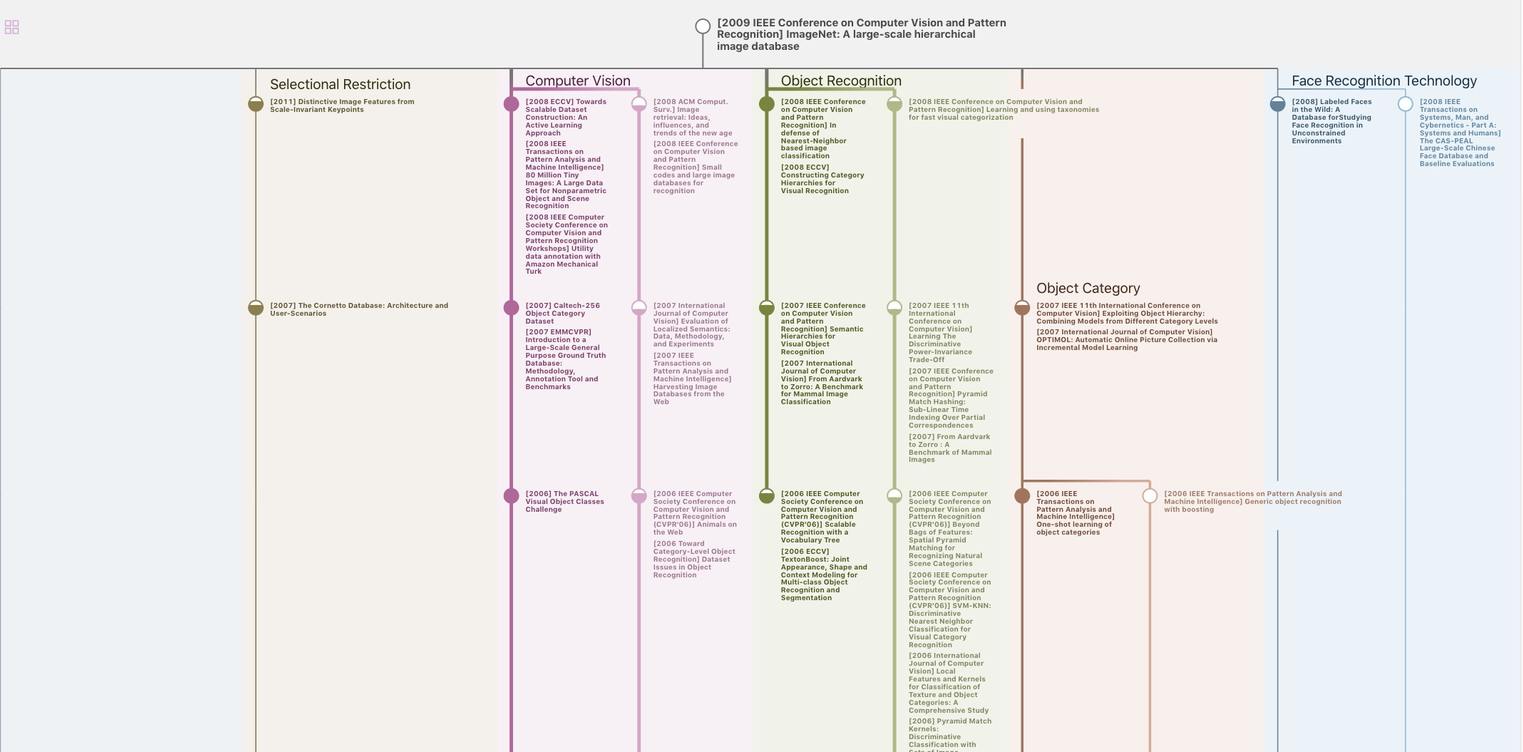
生成溯源树,研究论文发展脉络
Chat Paper
正在生成论文摘要