Replacing Data Augmentation with Rotation-Equivariant CNNs in Image-Based Classification of Oral Cancer.
CIARP(2021)
摘要
We present how replacing convolutional neural networks with a rotation-equivariant counterpart can be used to reduce the amount of training images needed for classification of whether a cell is cancerous or not. Our hypothesis is that data augmentation schemes by rotation can be replaced, thereby increasing weight sharing and reducing overfitting. The dataset at hand consists of single cell images. We have balanced a subset of almost 9.000 images from healthy patients and patients diagnosed with cancer. Results show that classification accuracy is improved and overfitting reduced if compared to an ordinary convolutional neural network. The results are encouraging and thereby an advancing step towards making screening of patients widely used for the application of oral cancer.
更多查看译文
关键词
data augmentation,oral,cancer,classification,rotation-equivariant,image-based
AI 理解论文
溯源树
样例
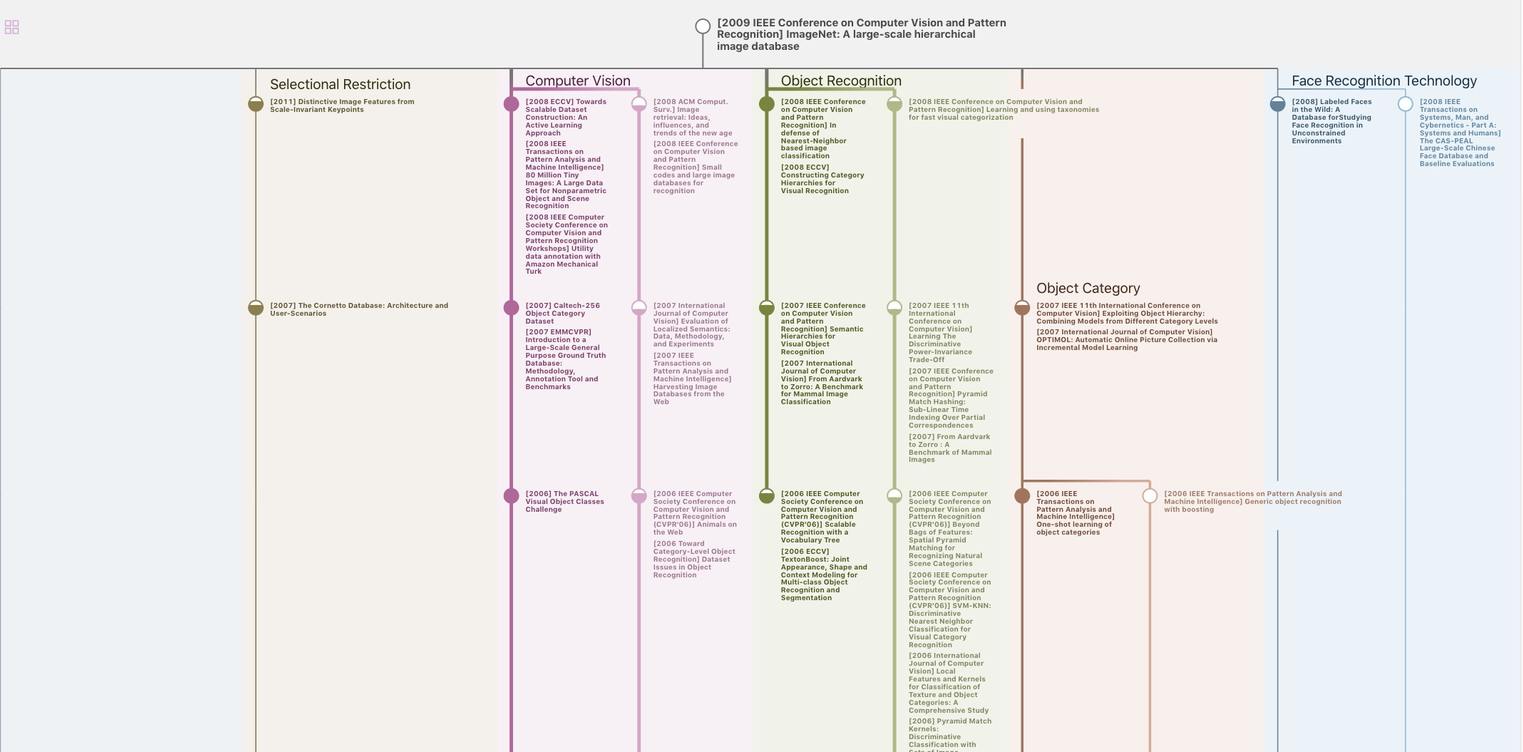
生成溯源树,研究论文发展脉络
Chat Paper
正在生成论文摘要