Generalization in Cardiac Image Segmentation.
IEEE BigData(2021)
摘要
Deep learning methods have achieved great success in medical imaging applications. Although data is very crucial for deep learning models, the medical imaging domain is restricted by the limited size of datasets and differences between them. This makes it difficult for models to generalize across datasets and achieve robust performance in practical settings. Therefore, knowing how to combine different datasets together to take advantage of new data while retaining performance on previous data becomes an important problem. In this paper, we focus on the task of cardiac image semantic segmentation and synthesize five different real-world scenarios to find the optimal training approach for deep learning models to achieve good generalization across different datasets.
更多查看译文
关键词
cardiac image segmentation,medical imaging applications,deep learning models,medical imaging domain,robust performance,practical settings,cardiac image semantic segmentation,synthesize five different real-world scenarios,good generalization
AI 理解论文
溯源树
样例
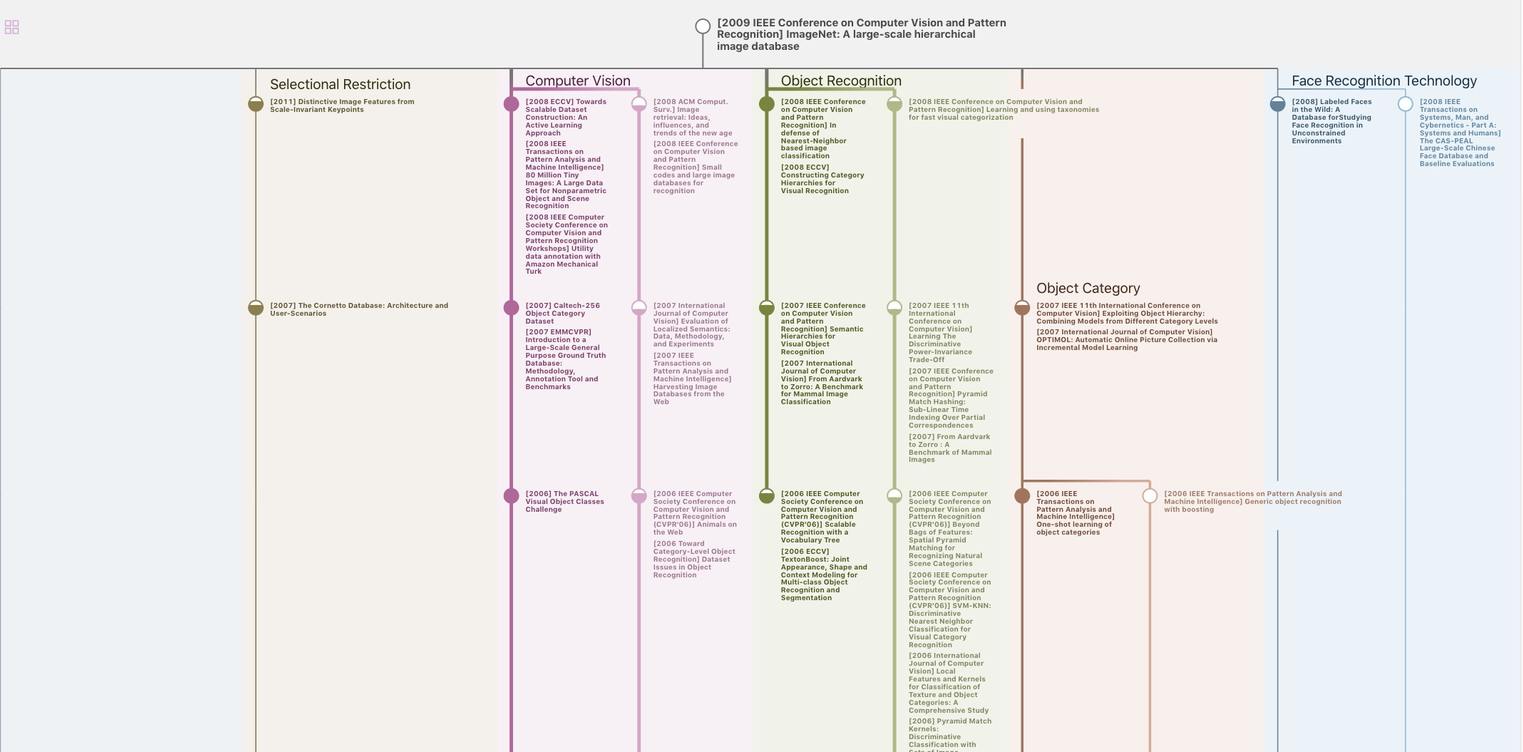
生成溯源树,研究论文发展脉络
Chat Paper
正在生成论文摘要