Multi-Task and Multi-Scene Unified Ranking Model for Online Advertising.
IEEE BigData(2021)
摘要
Online advertising and recommender systems often pose a multi-task problem, which tries to predict not only users' click-through rate (CTR) but also the post-click conversion rate (CVR). Meanwhile, multi-functional information systems commonly provide multiple service scenarios for users, such as news feed, search engine and product suggestions. Users may leave similar interest information across various service scenarios. Thus the prediction/ranking model should be conducted in a multiscene manner. This paper develops a unified ranking model for this multi-task and multi-scene problem. Compared to previous works, our model explores independent/non-shared embeddings for each task and scene, which reduces the coupling between tasks and scenes. New tasks or scenes could be added easily. Besides, a simplified network is chosen beyond the embedding layer, which largely improves the ranking efficiency for online services. Extensive offline and on line experiments demonstrated the superiority of the proposed unified ranking model.
更多查看译文
关键词
online advertising,learning to rank,multi-task learning,deep neural network,recommendation
AI 理解论文
溯源树
样例
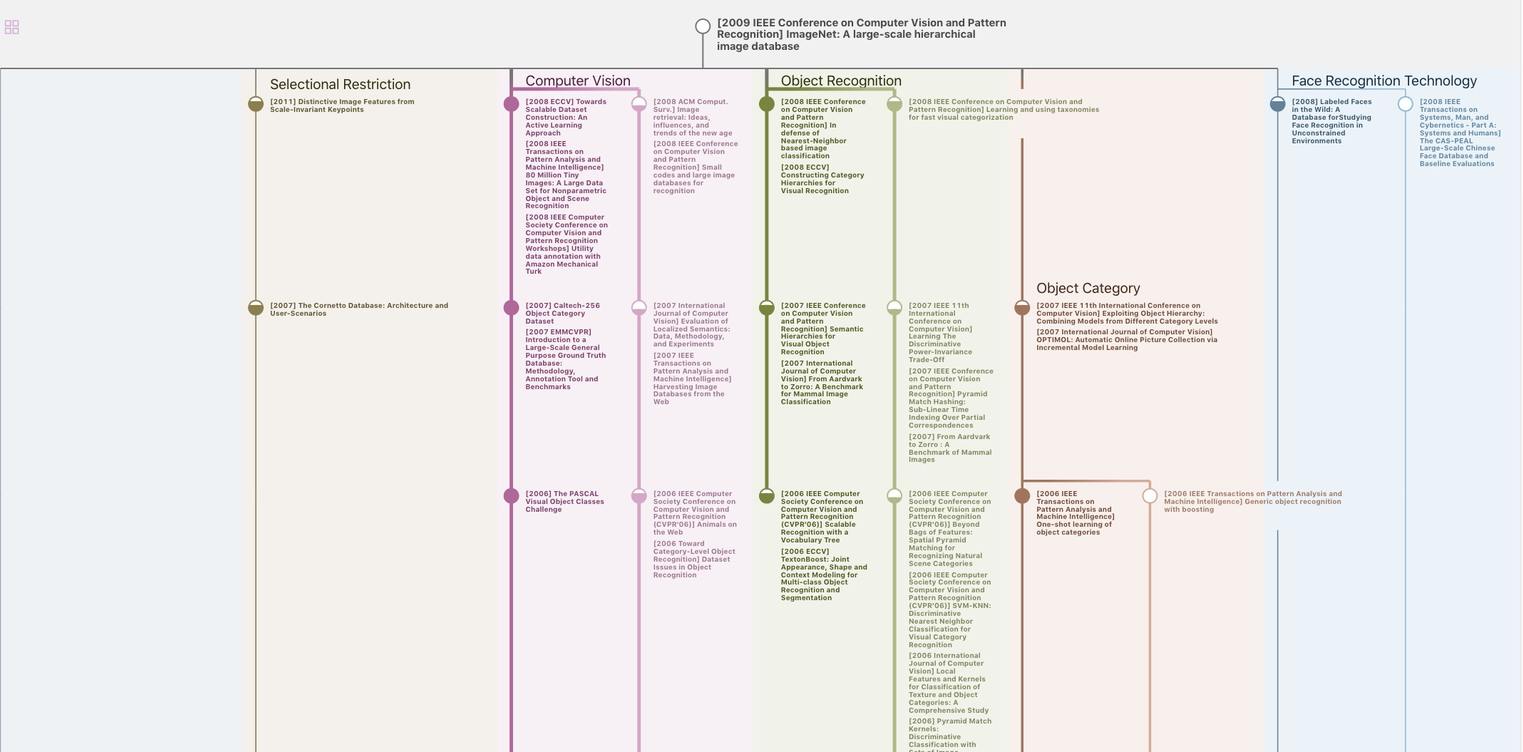
生成溯源树,研究论文发展脉络
Chat Paper
正在生成论文摘要