Shallow Node Representation Learning using Centrality Indices.
IEEE BigData(2021)
摘要
In recent years, learning embeddings for nodes of a graph has become one of the most efficient ways to solve different graph problems such as link prediction, clustering and classification. In this paper, we propose a novel method, called SECI, for learning embeddings of nodes, with application to link prediction. SECI samples from the network using breadth-first search and depth-first search, and interpolates between these two using centrality indices. The intuition behind SECI is that for nodes that have a low centrality score only a very small neighborhood is explored; and for dominant nodes that have a high centrality score a large neighborhood is explored. We evaluate the empirical performance of SECI over several real-world networks and show that it outperforms well-known existing algorithms.
更多查看译文
关键词
Graphs (networks),node embeddings,shallow representation learning,link prediction,centrality indices
AI 理解论文
溯源树
样例
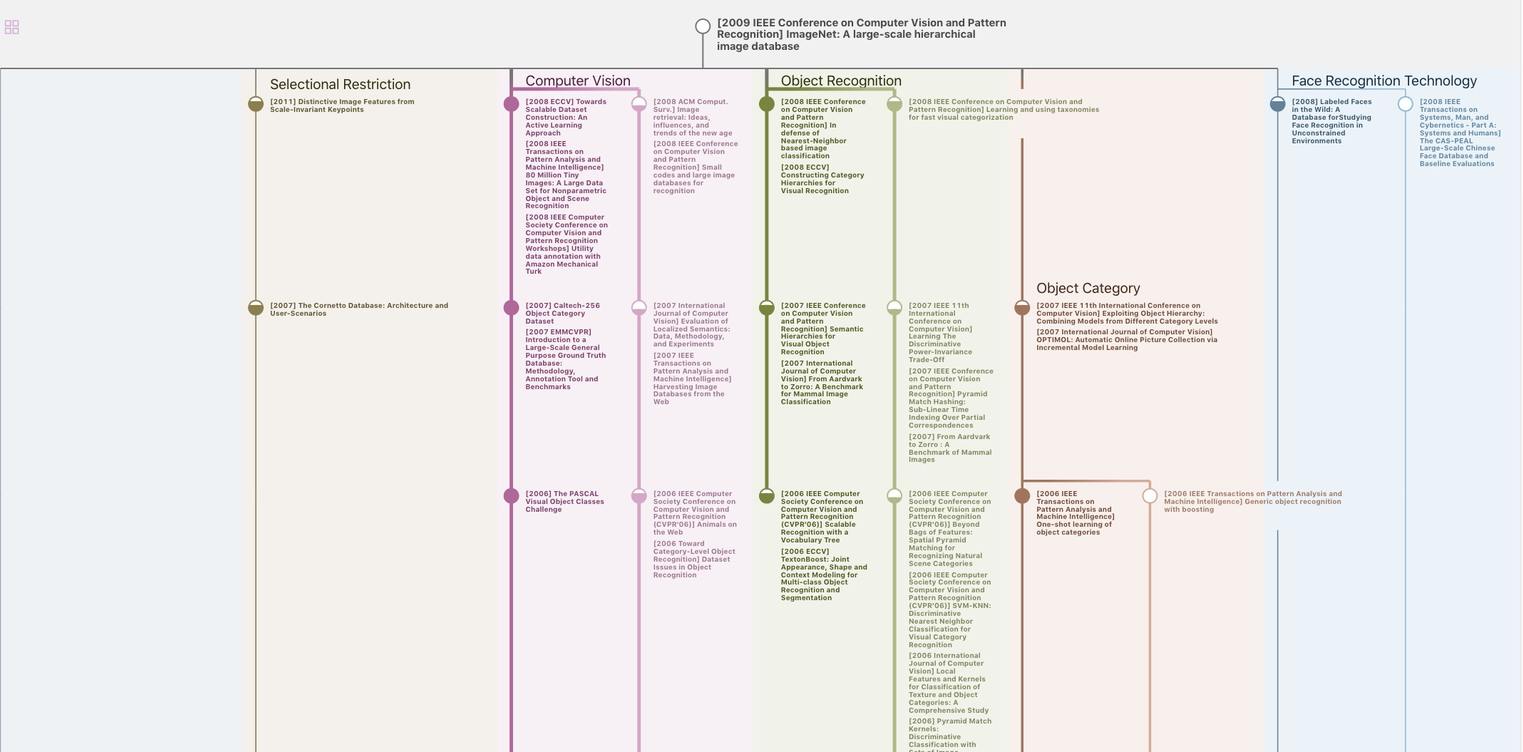
生成溯源树,研究论文发展脉络
Chat Paper
正在生成论文摘要