Learning to Drive (L2D) as a Low-Cost Benchmark for Real-World Reinforcement Learning
2021 20th International Conference on Advanced Robotics (ICAR)(2021)
摘要
We present Learning to Drive (L2D), a low-cost benchmark for real-world reinforcement learning (RL). L2D involves a simple and reproducible experimental setup where an RL agent has to learn to drive a Donkey car around three miniature tracks, given only monocular image observations and speed of the car. The agent has to learn to drive from disengagements, which occurs when it drives off the track. We present and open-source our training pipeline, which makes it straightforward to apply any existing RL algorithm to the task of autonomous driving with a Donkey car. We test imitation learning, state-of-the-art model-free, and model-based algorithms on the proposed L2D benchmark. Our results show that existing RL algorithms can learn to drive the car from scratch in less than five minutes of interaction. We demonstrate that RL algorithms can learn from sparse and noisy disengagement to drive even faster than imitation learning and a human operator.
更多查看译文
关键词
reinforcement learning,l2d,benchmark,low-cost,real-world
AI 理解论文
溯源树
样例
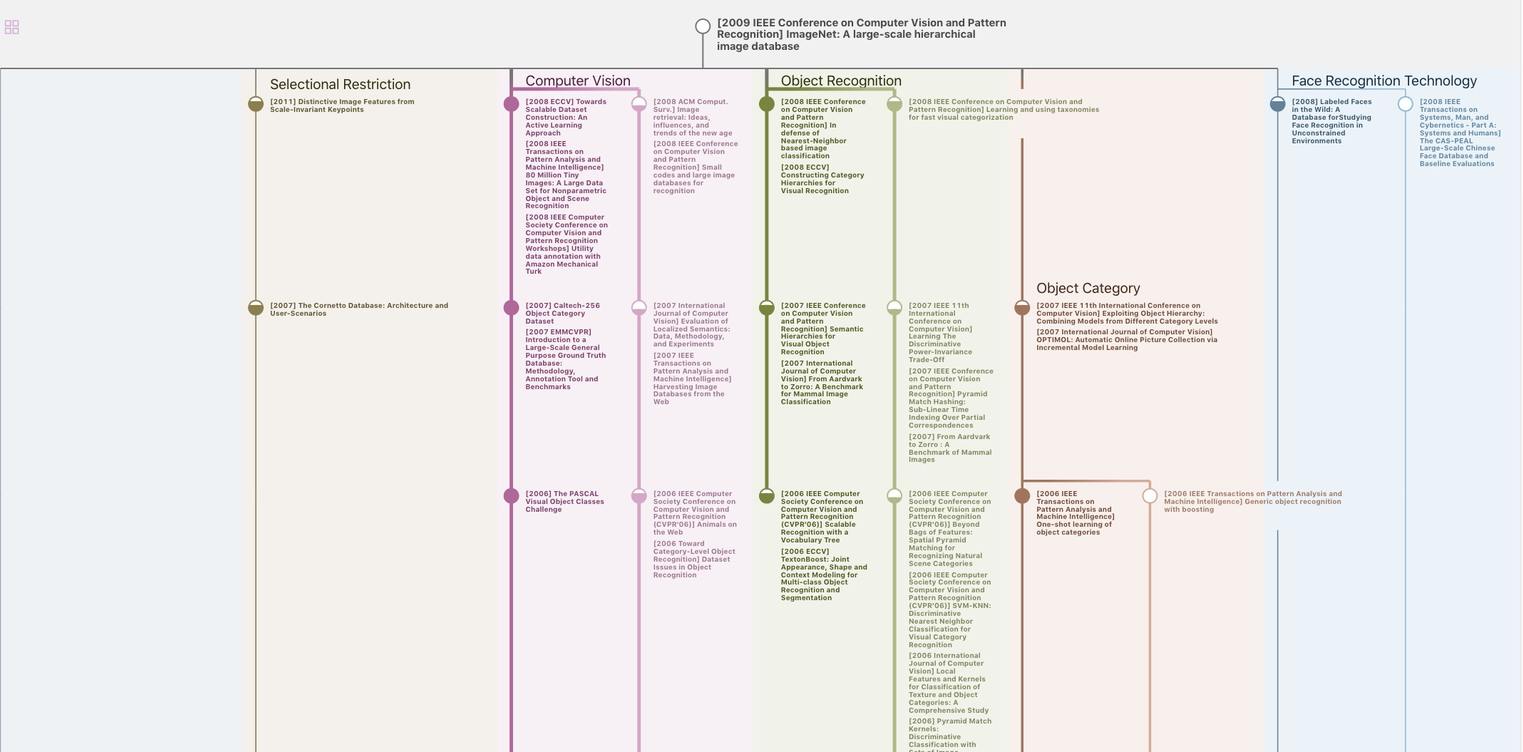
生成溯源树,研究论文发展脉络
Chat Paper
正在生成论文摘要