Towards a Mobile Robot Localization Benchmark with Challenging Sensordata in an Industrial Environment
2021 20TH INTERNATIONAL CONFERENCE ON ADVANCED ROBOTICS (ICAR)(2021)
摘要
To arrive at a realistic assessment of localization methods in terms of their performance in an industrial environment under various challenging conditions, we provide a benchmark to evaluate algorithms both for individual components as well as multi-sensor systems. For several sensor types, including wheel odometry, RGB cameras, RGB-D cameras, and LIDAR, potential issues were identified. The accuracy of wheel odometry, for example, when there are bumps on the track. For each sensor type, we explicitly chose a track for the benchmark dataset containing situations where the sensor fails to provide adequate measurements. Based on the acquired sensor data, localization can be achieved either using a single sensor information or sensor fusion. To help evaluate the output of associated localization algorithms, we provide a software to evaluate a set of metrics as part of the paper. An example application of the benchmark with state-of-the-art algorithms for each sensor is also provided.
更多查看译文
关键词
wheel odometry,sensor type,single sensor information,mobile robot localization benchmark,sensor data,industrial environment,multisensor systems,RGB-D cameras,LIDAR,sensor fusion
AI 理解论文
溯源树
样例
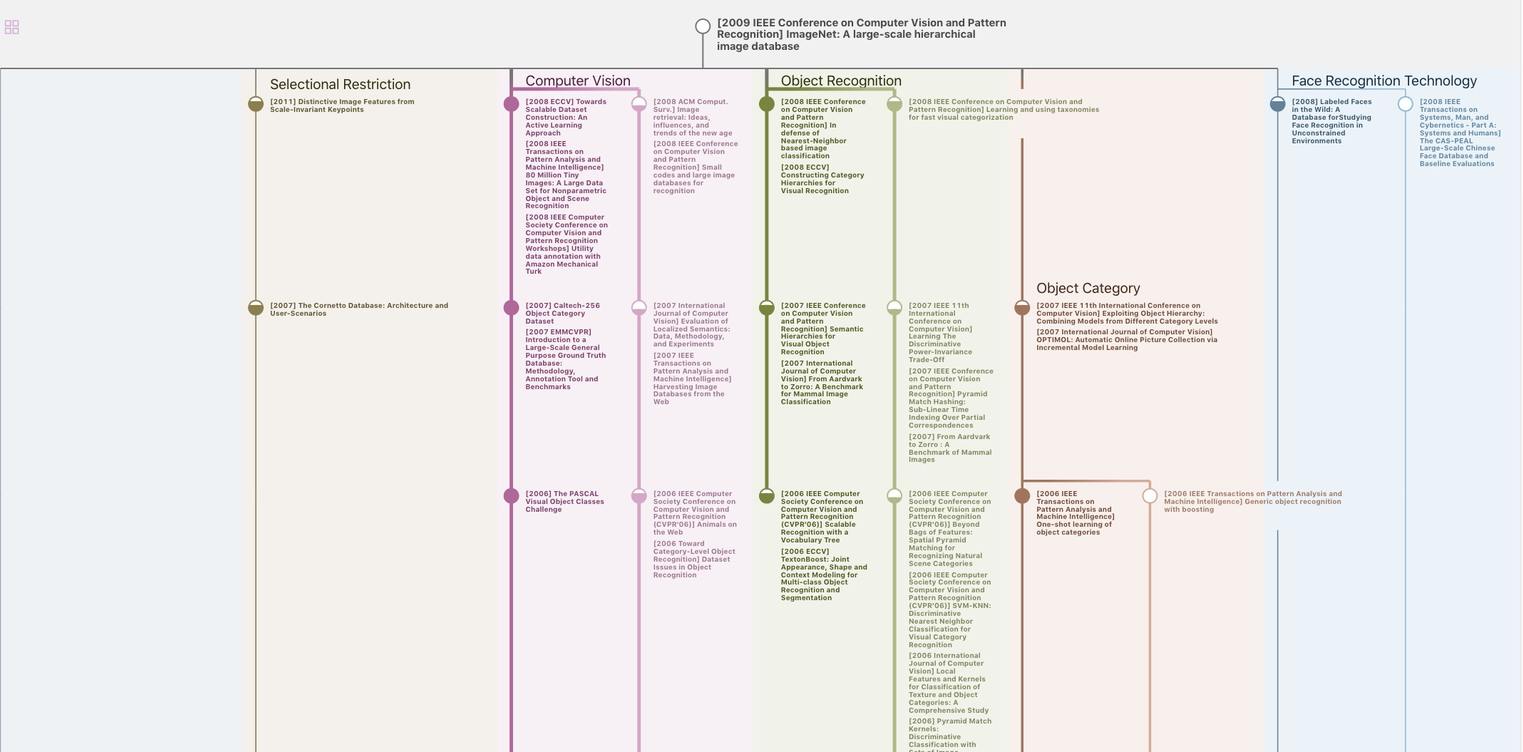
生成溯源树,研究论文发展脉络
Chat Paper
正在生成论文摘要