FoodNet - Simplifying Online Food Ordering with Contextual Food Combos.
COMAD/CODS(2022)
摘要
Bundling complementary dishes into easy-to-order food combos is vital to providing a seamless food ordering experience. Manually curating combos across several thousands of restaurants and millions of dishes is neither scalable nor can be personalized. We propose FoodNet, an attention-based deep learning architecture with a monotonically decreasing constraint of diversity, to recommend personalized two-item combos from across different restaurants. In a large-scale evaluation involving 200 million candidate combos, we show that FoodNet outperforms the Transformer based model by 1.3%, the Siamese network based model by 13.6%, and the traditional Apriori baseline by 18.8% in terms of NDCG, which are significant improvements at our scale. We also present qualitative results to show the importance of attention and lattice layers in the proposed architecture.
更多查看译文
AI 理解论文
溯源树
样例
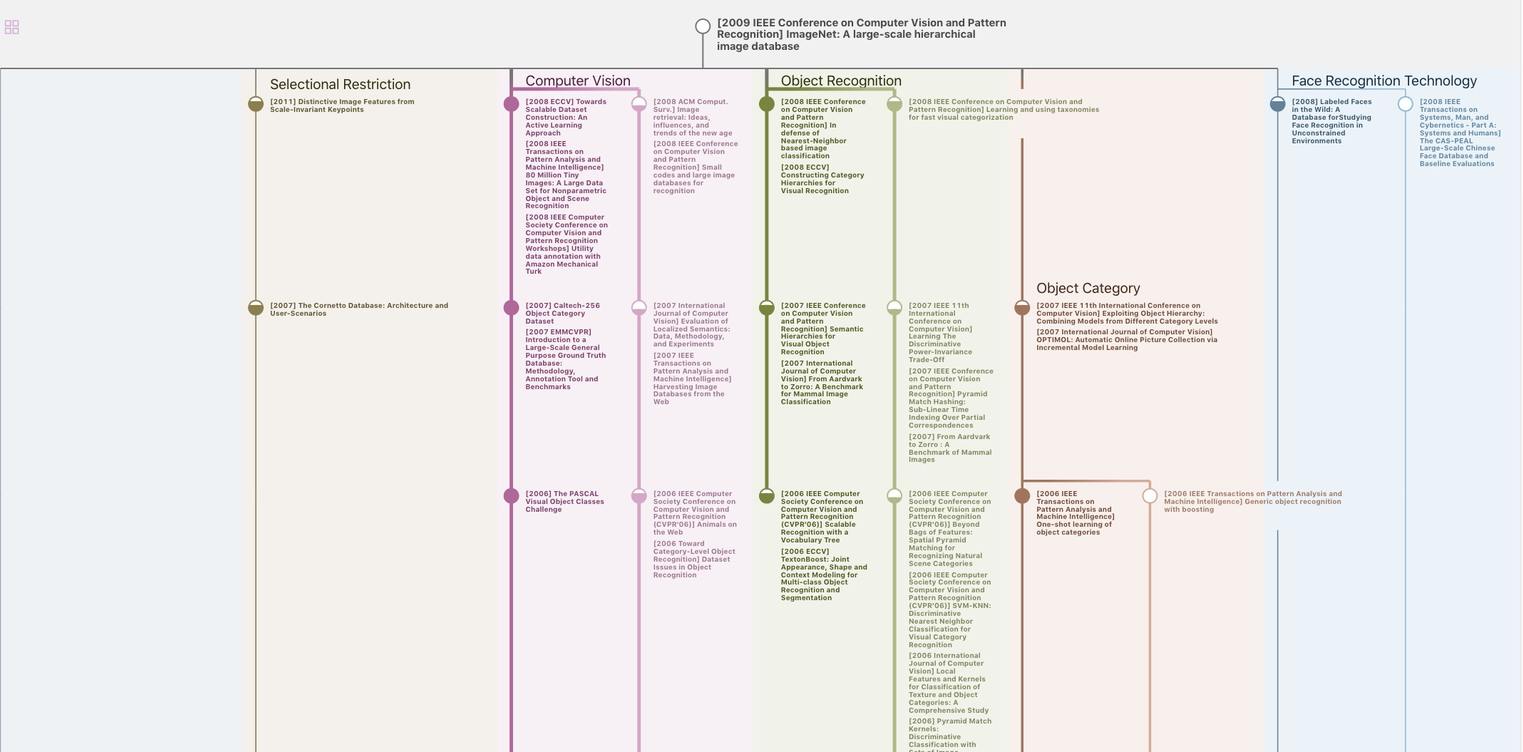
生成溯源树,研究论文发展脉络
Chat Paper
正在生成论文摘要