ComParE - A User Behavior Centric Framework for Personalized Recommendations in Skill Gaming Platforms.
COMAD/CODS(2022)
摘要
Recommendation in outcome based platforms (eg. skill gaming, financial trading) where users get rewards based on their choices and the subsequent non-deterministic outcomes from such choices presents a unique set of challenges. Unlike other online services (e.g., e-commerce, social media), these platforms often see: (a) distinctive longitudinal behavior patterns in users’ transactions, (b) enormous content generated by the user interactions, (c) a dynamic interplay between the users’ transactional history and outcomes towards their future behavior, (d) ordinal nature of transaction elements. Motivated by these observations, we propose ComParE (Competing Parallel Networks with Expert Network), a novel personalized recommendation framework that: (i) exploits the distinct behavioral trends in the data, by training competing parallel networks as local experts, (ii) trains a global expert network to get the overall picture for the final prediction, and (iii) introduces custom loss functions to learn inherent ordering and interpretations of the classes being predicted. With the example of personalizing entry fee choices for the game of Rummy on the RummyCircle platform we show: (i) distinct and robust user Personas found based on historical entry fee selections; (ii) significant boosts in the entry fee predictions through ComParE with both neural networks and classical ML algorithms for local and global experts; (iii) substantial lifts over platform default as shown through offline analysis. ComParE significantly outperforms other baselines including well known deep learning models, as shown through offline experiments.
更多查看译文
AI 理解论文
溯源树
样例
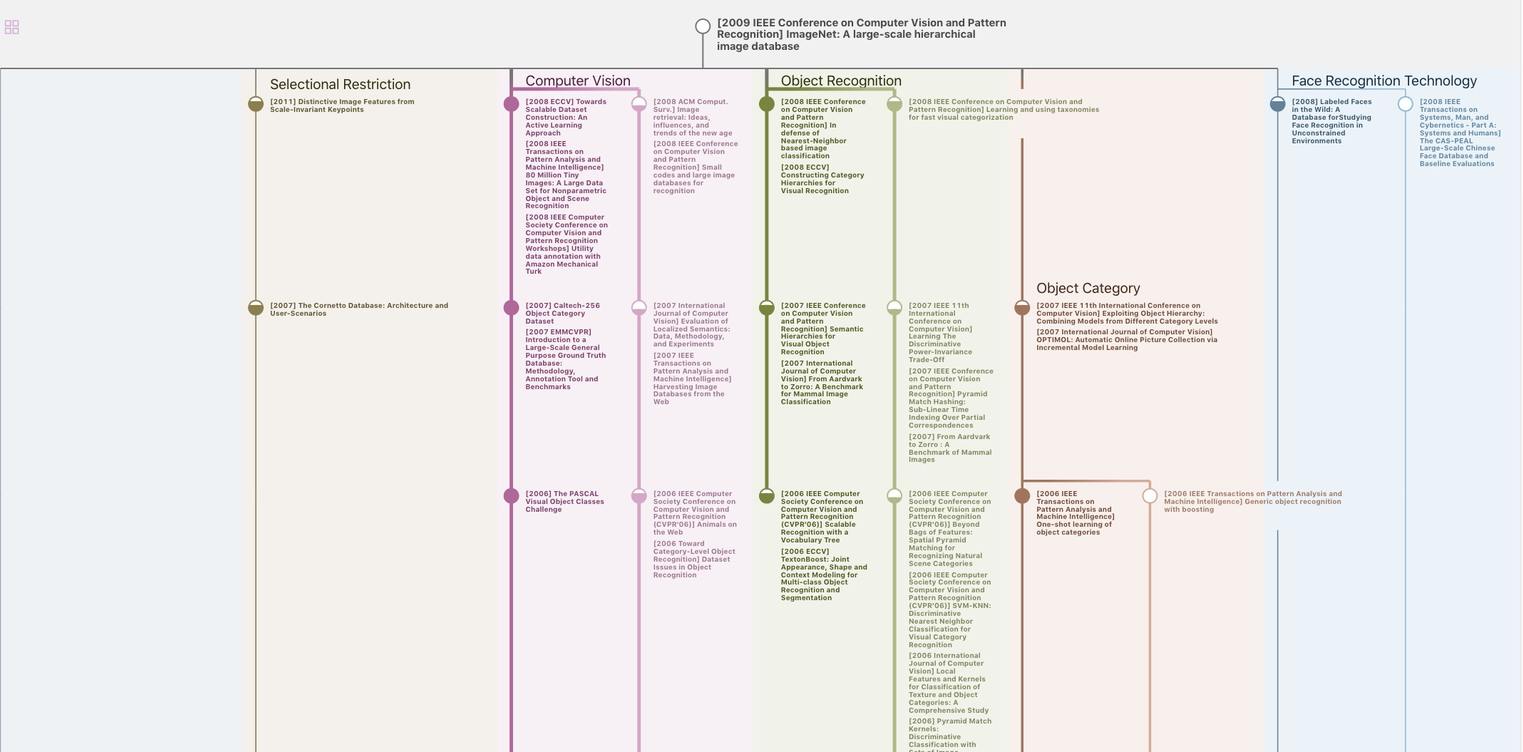
生成溯源树,研究论文发展脉络
Chat Paper
正在生成论文摘要