Accounting for the Effect of Inter-Task Similarity in Continual Learning Models.
SMC(2021)
摘要
Catastrophic forgetting has long been a major obstacle to continual learning. In this paper, we explore the effect of certain task characteristics, in particular inter-task similarity, on the extent of forgetting. Moreover, we experimentally study the effect of these characteristics on the effectiveness of recent state-of-the-art continual learning approaches. We show that the performance of some methods, for example, the recently proposed Learning without Forgetting (LwF) and elastic weight consolidation (EWC) models, is significantly dependent on these characteristics. We propose a rehearsal-based extension to continual learning models to address this vulnerability. We develop this extension first in the context of LwF and later demonstrate its effectiveness with other models such as EWC. We show that a memory budget of 1% of training data is sufficient to significantly improve on performance in cases of low inter-task similarity.
更多查看译文
关键词
learning,models*,inter-task
AI 理解论文
溯源树
样例
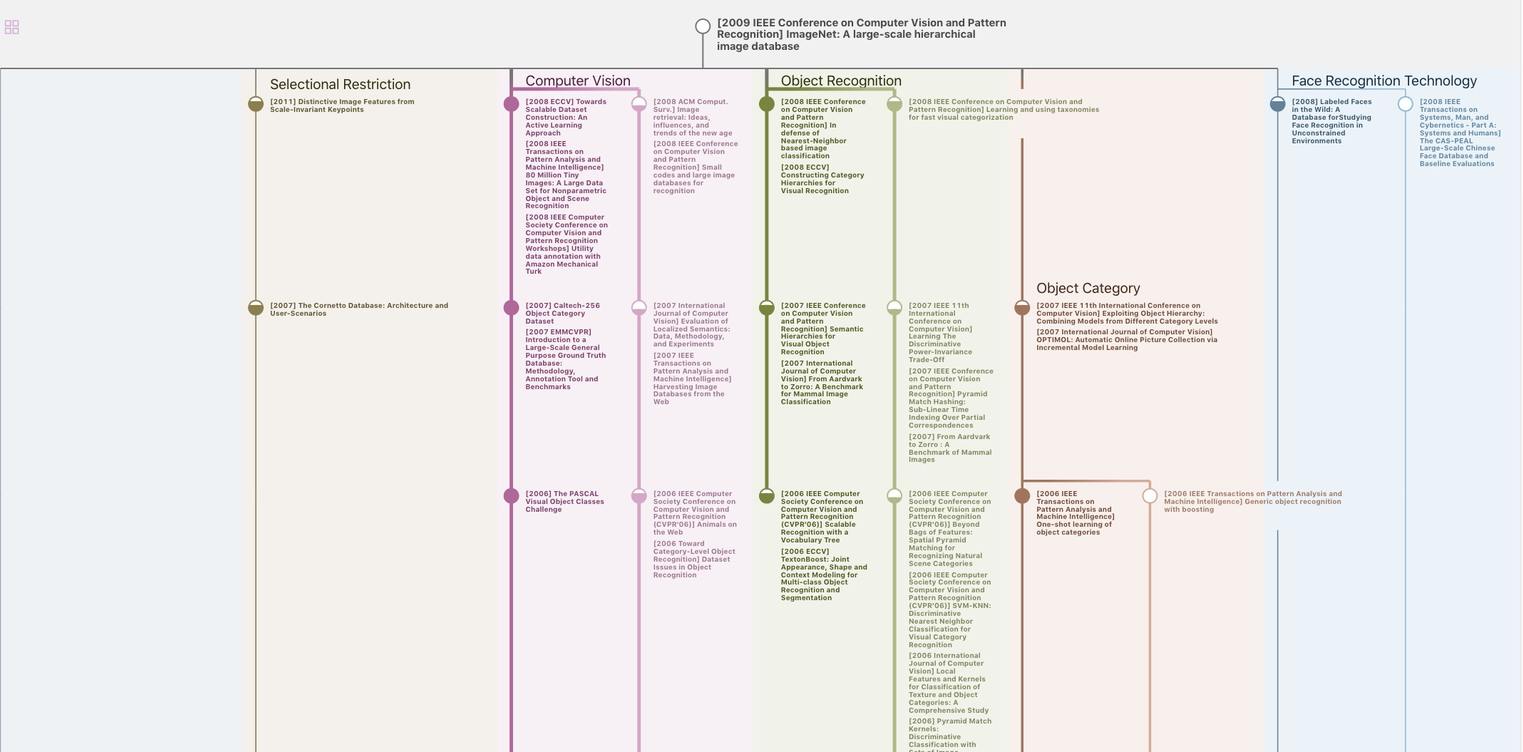
生成溯源树,研究论文发展脉络
Chat Paper
正在生成论文摘要