Structural Knowledge Organization and Transfer for Class-Incremental Learning
International Multimedia Conference(2021)
摘要
ABSTRACT Deep models are vulnerable to catastrophic forgetting when fine-tuned on new data. Popular distillation-based methods usually neglect the relations between data samples and may eventually forget essential structural knowledge. To solve these shortcomings, we propose a structural graph knowledge distillation based incremental learning framework to preserve both the positions of samples and their relations. Firstly, a memory knowledge graph (MKG) is generated to fully characterize the structural knowledge of historical tasks. Secondly, we develop a graph interpolation mechanism to enrich the domain of knowledge and alleviate the inter-class sample imbalance issue. Thirdly, we introduce structural graph knowledge distillation to transfer the knowledge of historical tasks. Comprehensive experiments on three datasets validate the proposed method.
更多查看译文
AI 理解论文
溯源树
样例
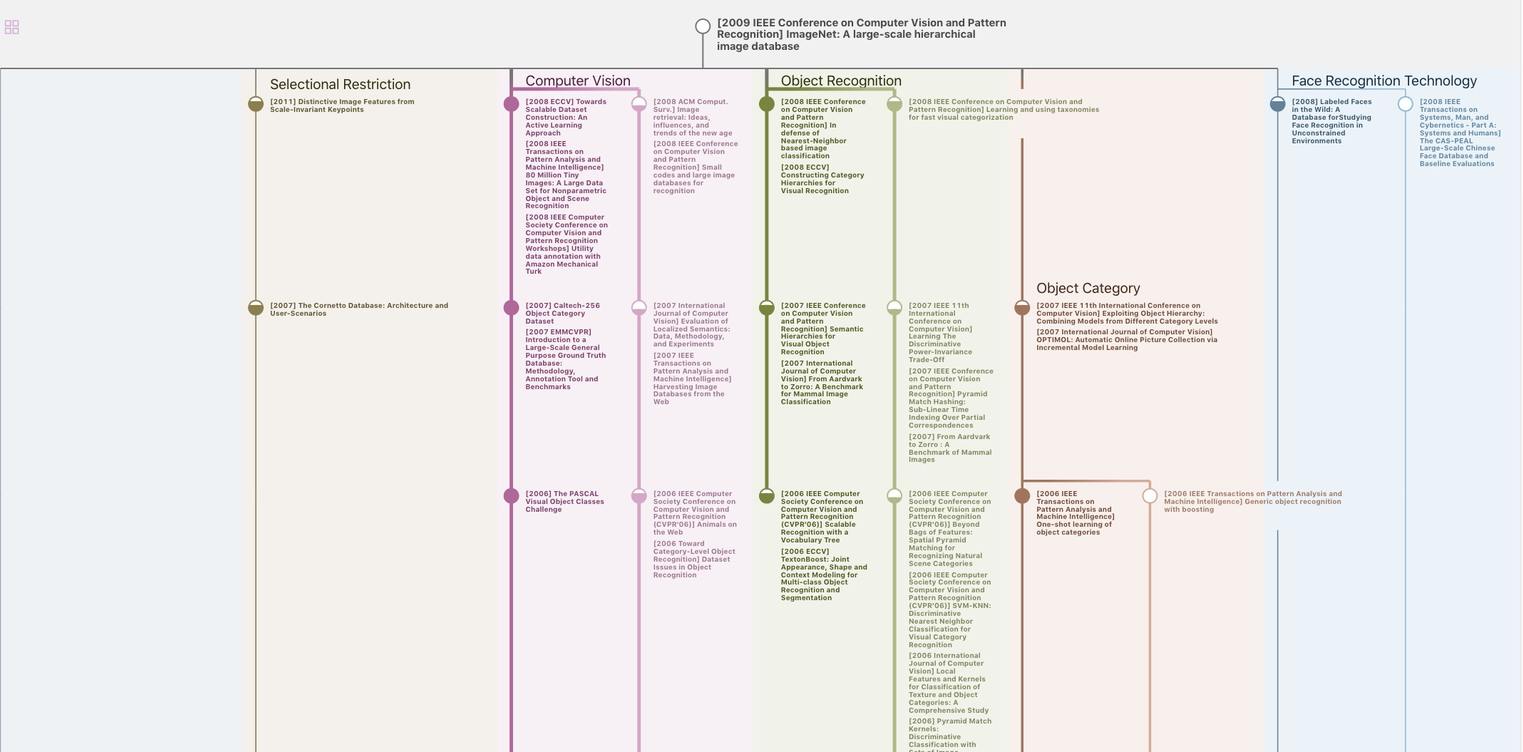
生成溯源树,研究论文发展脉络
Chat Paper
正在生成论文摘要