Will you go where you search? A deep learning framework for estimating user search-and-go behavior
Neurocomputing(2022)
摘要
•To the best of our knowledge, our work is the first attempt to model search-and-go human behavior as a CTR-like binary classification problem in recommender system.•We propose an effective end-to-end deep learning model with a wide&deep architecture by utilizing LSTM attention and factorization-machine, to learn the sophisticated interactions between mobility data and search data.•We evaluate the framework on real-world dataset collected from Yahoo! Japan portal application, and demonstrate satisfactory performances on multiple search-and-go scenarios at a nation-wide level.•Our propsed model DeepSTIN achieved better performances comparing with the baseline models such as GBDT, DNN, Factorization-supported Neural Network [48], Product-based Neural Network [26], Wide&Deep [2], Deep & Cross Network [31], DeepFM [10], and XDeepFM [19].•DeepSTIN improves the performance of DeepFM on four datasets by {6.4%, 7.9%, 11.8%, 9.3%} in AUC and {7.8%, 8.5%, 22.0%, 26.8%} in LogLoss.
更多查看译文
关键词
User behavior modeling,Recommender system,Location-based services,Ubiquitous and mobile computing,Deep learning
AI 理解论文
溯源树
样例
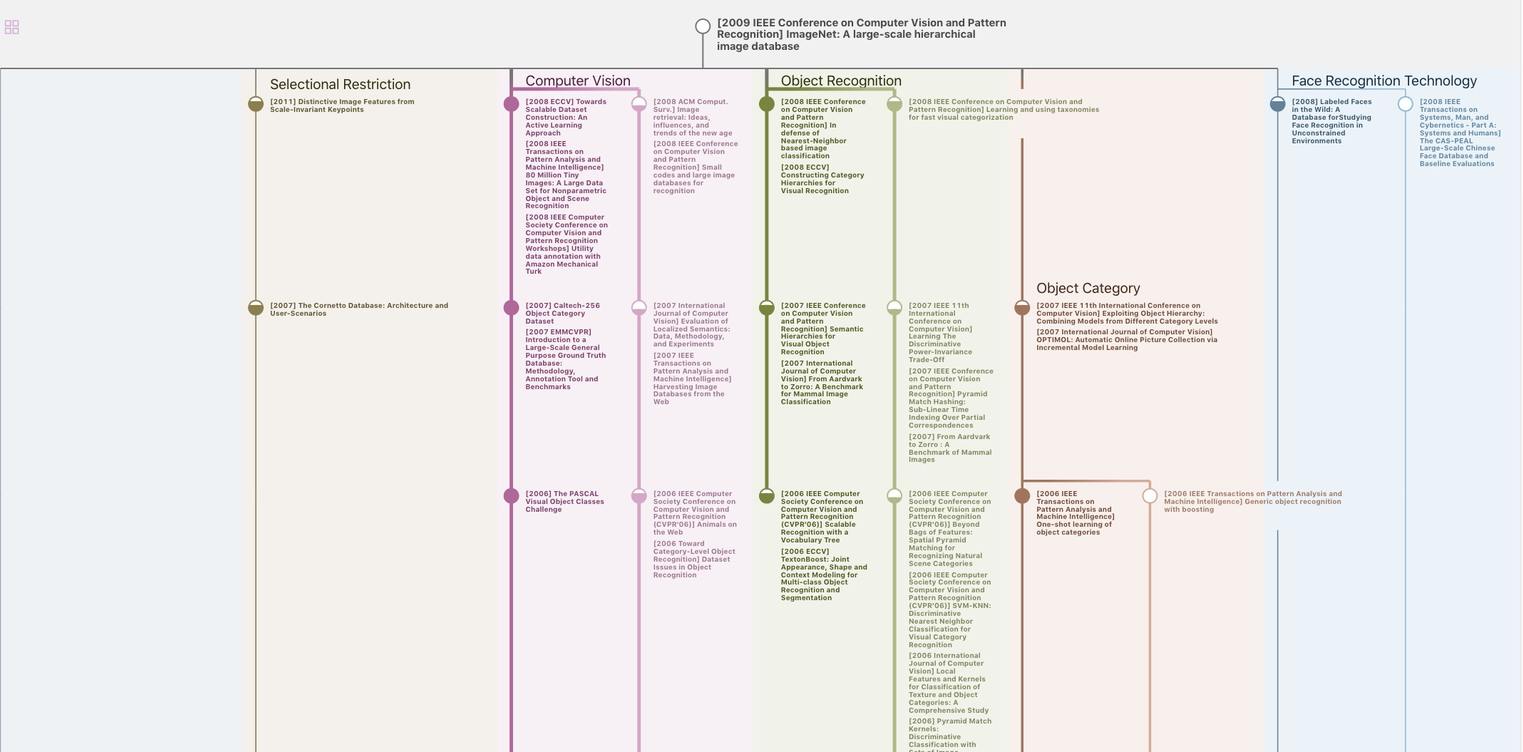
生成溯源树,研究论文发展脉络
Chat Paper
正在生成论文摘要