Improved Active Deep Learning for Semi-Supervised Classification of Hyperspectral Image
REMOTE SENSING(2022)
摘要
Hyperspectral image (HSI) data classification often faces the problem of the scarcity of labeled samples, which is considered to be one of the major challenges in the field of remote sensing. Although active deep networks have been successfully applied in semi-supervised classification tasks to address this problem, their performance inevitably meets the bottleneck due to the limitation of labeling cost. To address the aforementioned issue, this paper proposes a semi-supervised classification method for hyperspectral images that improves active deep learning. Specifically, the proposed model introduces the random multi-graph algorithm and replaces the expert mark in active learning with the anchor graph algorithm, which can label a considerable amount of unlabeled data precisely and automatically. In this way, a large number of pseudo-labeling samples would be added to the training subsets such that the model could be fine-tuned and the generalization performance could be improved without extra efforts for data manual labeling. Experiments based on three standard HSIs demonstrate that the proposed model can get better performance than other conventional methods, and they also outperform other studied algorithms in the case of a small training set.
更多查看译文
关键词
active deep learning, hyperspectral images, random multi-graph, small samples
AI 理解论文
溯源树
样例
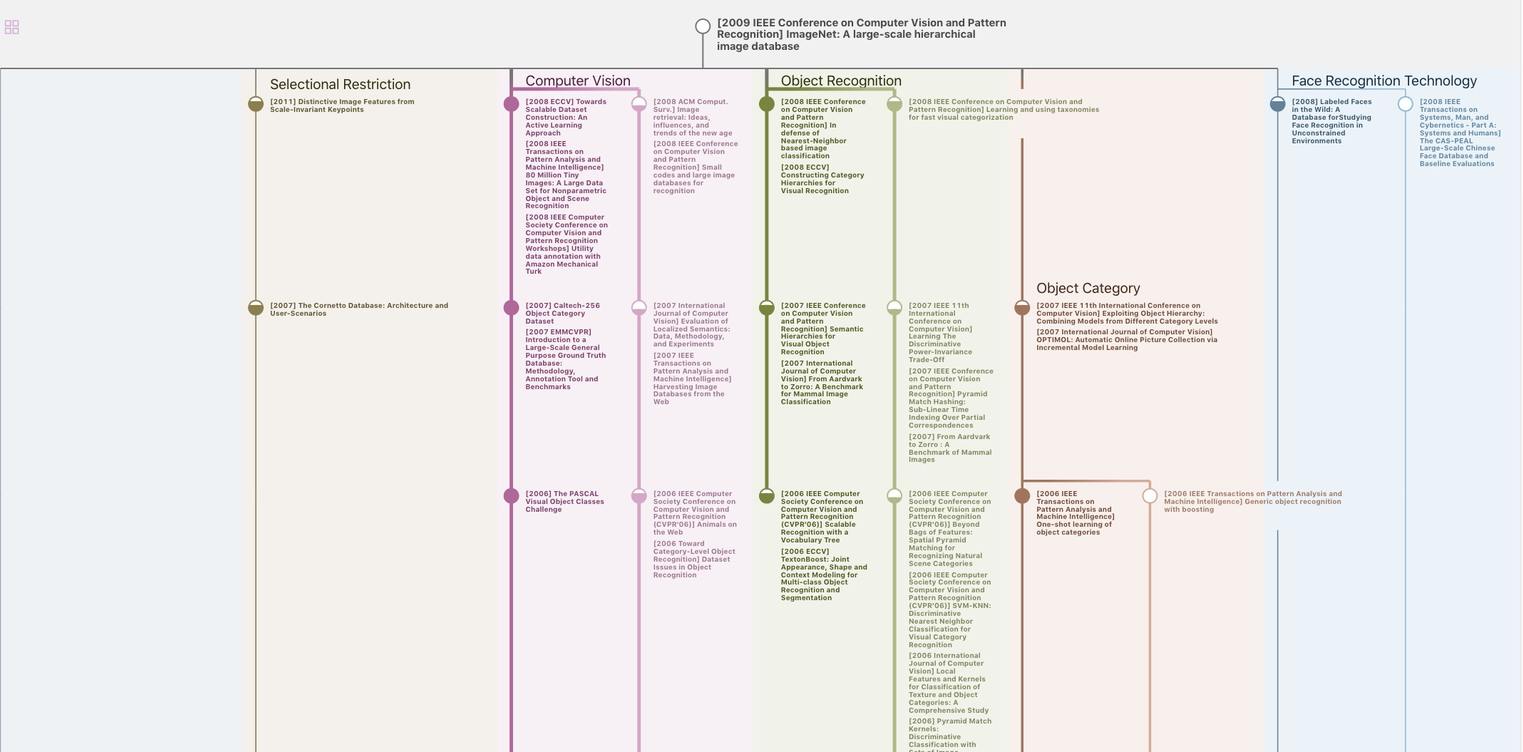
生成溯源树,研究论文发展脉络
Chat Paper
正在生成论文摘要