Mining of Weak Fault Information Adaptively Based on DNN Inversion Estimation for Fault Diagnosis of Rotating Machinery
IEEE ACCESS(2022)
摘要
Fault feature extraction plays a significant role in bearing fault diagnosis, especially in incipient fault period. Recently, deep neural network has been favored by many researchers due to its excellent hierarchical feature extraction capabilities. The existing diagnosis methods based on deep neural network mostly take original condition monitoring data as input and further convert fault diagnosis into pattern recognition issues. Although it improves the level of intelligent diagnosis, it is still confronted with practical problems. Since deep neural network includes many non-linear mapping layers, the extracted fault-related features show a high degree of abstraction, which reduces the practical level of this technology. Aimed at this problem, a mining method of understandably weak fault information is proposed based on deep neural network inversion estimation. From perspective of neuron response degree, this method mines the most sensitive input pattern that maximizes the neuron's activation value of network output layer in the original input feature space. It can intuitively mine the weak fault information from the original signal. Two bearing experiments verify the effectiveness and reliability of the proposed method.
更多查看译文
关键词
Feature extraction, Mathematical models, Fault diagnosis, Data mining, Deep learning, Neurons, Condition monitoring, Deep neural network inversion estimation, fault feature extraction, mining of weak fault information
AI 理解论文
溯源树
样例
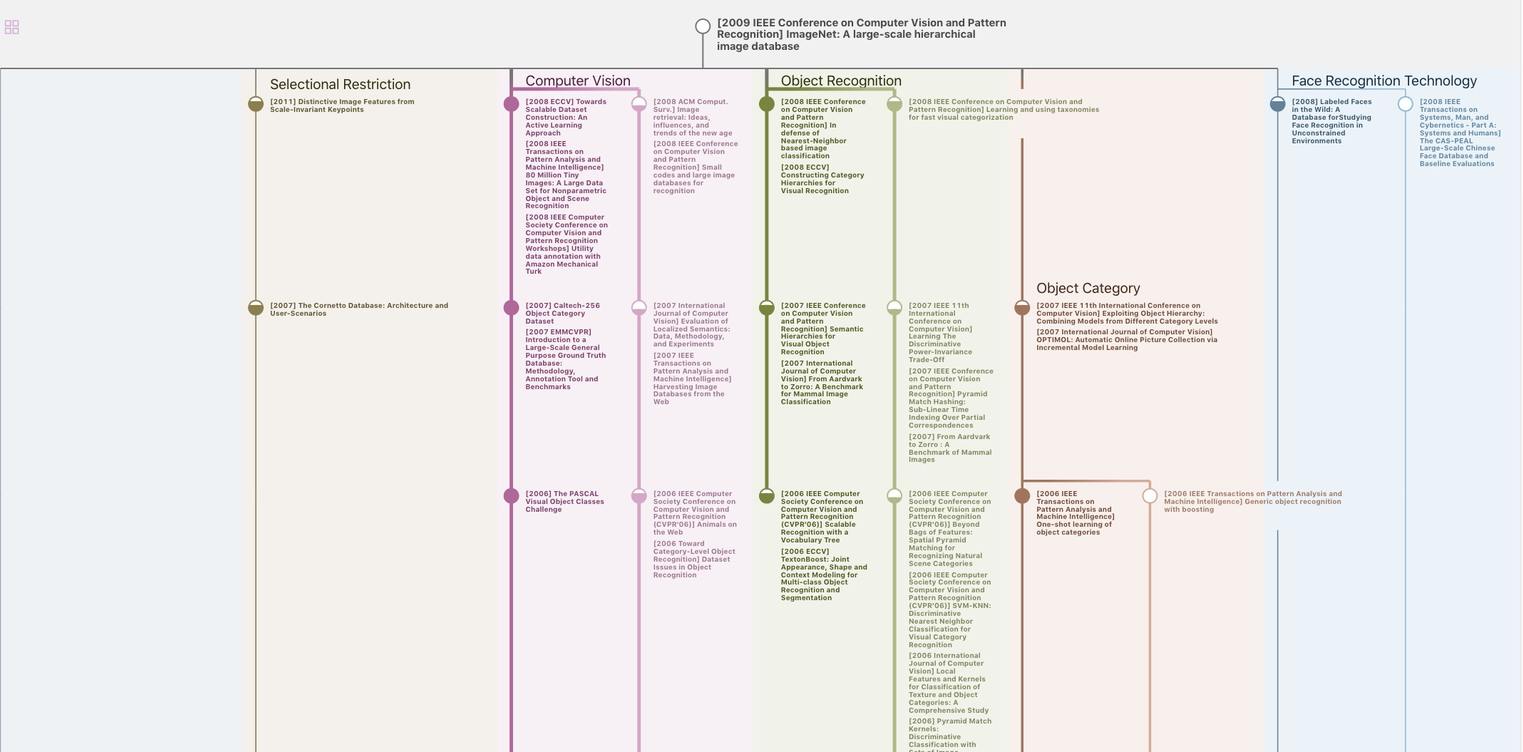
生成溯源树,研究论文发展脉络
Chat Paper
正在生成论文摘要