I-CNet: Leveraging Involution and Convolution for Image Classification
IEEE ACCESS(2022)
摘要
Convolution is widely adapted in deep learning models on image classification tasks for extracting hidden spatial-domain representations. However, as convolution is channel-specific, the potential cross-channel correlations in images are often neglected. This paper proposes a novel model, namely I-CNet, which leverages involution and convolution for improving the accuracy of image classification tasks, by extracting feature representations on both channel domain and spatial domain. The proposed I-CNet has been experimented on three image classification datasets. The experimental results show that involution component employed in I-CNet model can effectively represent cross-channel features in images and I-CNet is superior to other comparatives with higher classification accuracy achieved on all the three datasets.
更多查看译文
关键词
Convolution, Transfer learning, Kernel, Feature extraction, Training, Task analysis, Optimization, Image classification, involution, convolution, hybrid architecture
AI 理解论文
溯源树
样例
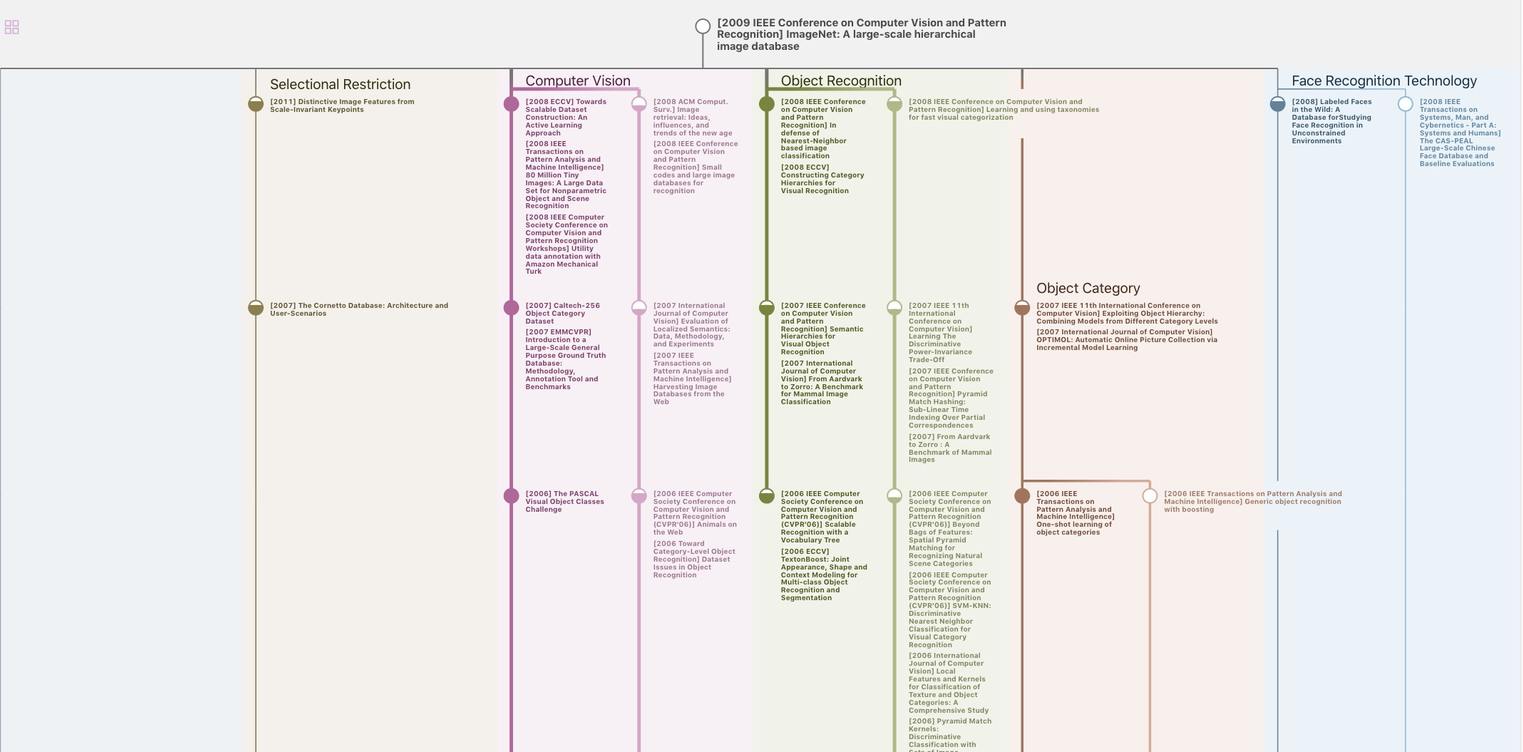
生成溯源树,研究论文发展脉络
Chat Paper
正在生成论文摘要