End-to-end diabetic retinopathy grading based on fundus fluorescein angiography images using deep learning
Graefe's Archive for Clinical and Experimental Ophthalmology(2022)
摘要
Purpose To develop and validate a deep learning system for diabetic retinopathy (DR) grading based on fundus fluorescein angiography (FFA) images. Methods A total of 11,214 FFA images from 705 patients were collected to form the internal dataset. Three convolutional neural networks, namely VGG16, RestNet50, and DenseNet, were trained using a nine-square grid input, and heat maps were generated. Subsequently, a comparison between human graders and the algorithm was performed. Lastly, the best model was tested on two external datasets (Xian dataset and Ningbo dataset). Results VGG16 performed the best, with a maximum accuracy of 94.17%, and had an AUC of 0.972, 0.922, and 0.994 for levels 1, 2, and 3, respectively. For Xian dataset, our model reached the accuracy of 82.47% and AUC of 0.910, 0.888, and 0.976 for levels 1, 2, and 3. As for Ningbo dataset, the network performed with the accuracy of 88.89% and AUC of 0.972, 0.756, and 0.945 for levels 1, 2, and 3. Conclusions A deep learning system for DR staging was trained based on FFA images and evaluated through human–machine comparisons as well as external dataset testing. The proposed system will help clinical practitioners to diagnose and treat DR patients, and lay a foundation for future applications of other ophthalmic or general diseases.
更多查看译文
关键词
Diabetic retinopathy, Fundus fluorescein angiography, Deep learning, Grading
AI 理解论文
溯源树
样例
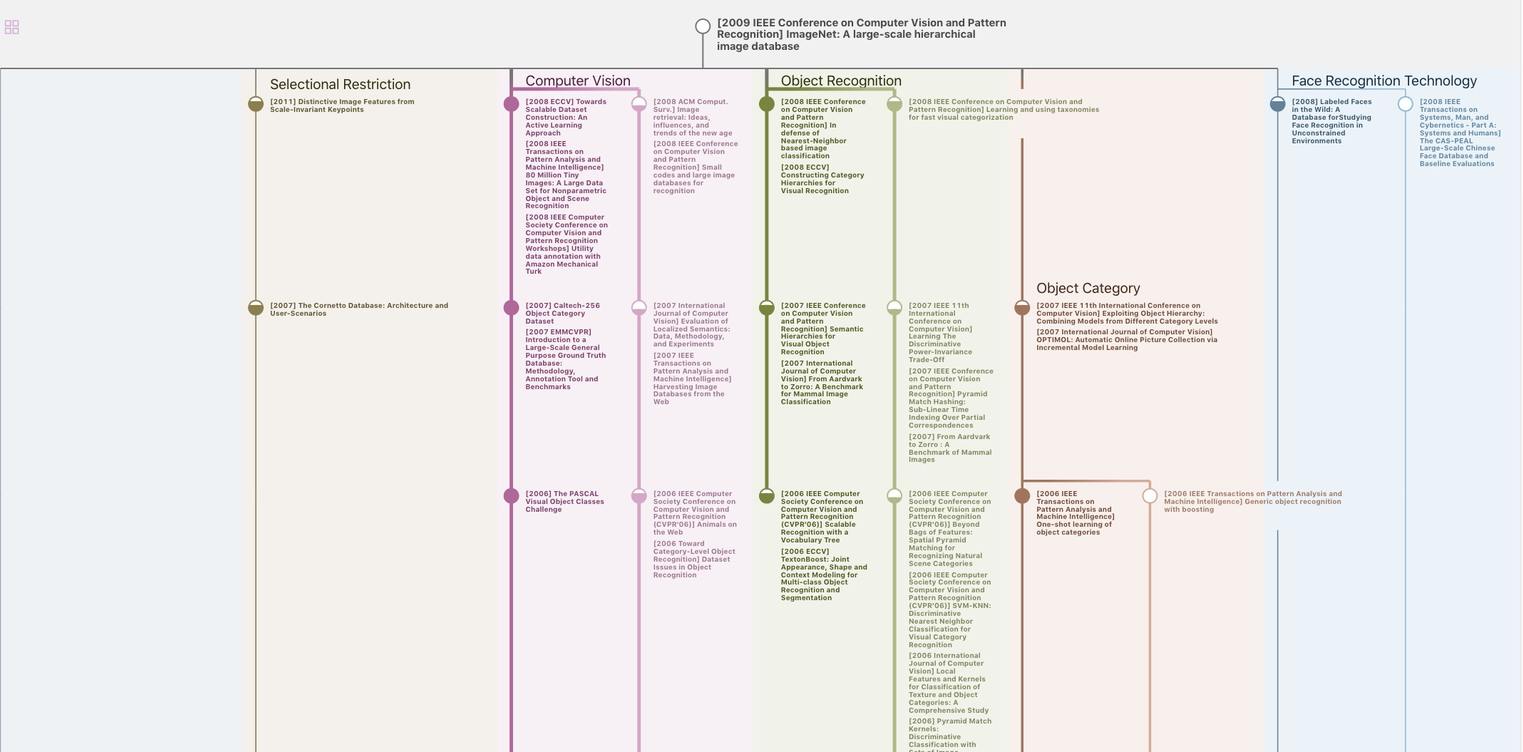
生成溯源树,研究论文发展脉络
Chat Paper
正在生成论文摘要