Trustworthy Knowledge Graph Completion Based on Multi-sourced Noisy Data
International World Wide Web Conference(2022)
摘要
ABSTRACT Knowledge graphs (KGs) have become a valuable asset for many AI applications. Although some KGs contain plenty of facts, they are widely acknowledged as incomplete. To address this issue, many KG completion methods are proposed. Among them, open KG completion methods leverage the Web to find missing facts. However, noisy data collected from diverse sources may damage the completion accuracy. In this paper, we propose a new trustworthy method that exploits facts for a KG based on multi-sourced noisy data and existing facts in the KG. Specifically, we introduce a graph neural network with a holistic scoring function to judge the plausibility of facts with various value types. We design value alignment networks to resolve the heterogeneity between values and map them to entities even outside the KG. Furthermore, we present a truth inference model that incorporates data source qualities into the fact scoring function, and design a semi-supervised learning way to infer the truths from heterogeneous values. We conduct extensive experiments to compare our method with the state-of-the-arts. The results show that our method achieves superior accuracy not only in completing missing facts but also in discovering new facts.
更多查看译文
关键词
Knowledge graph completion, truth inference, noisy data
AI 理解论文
溯源树
样例
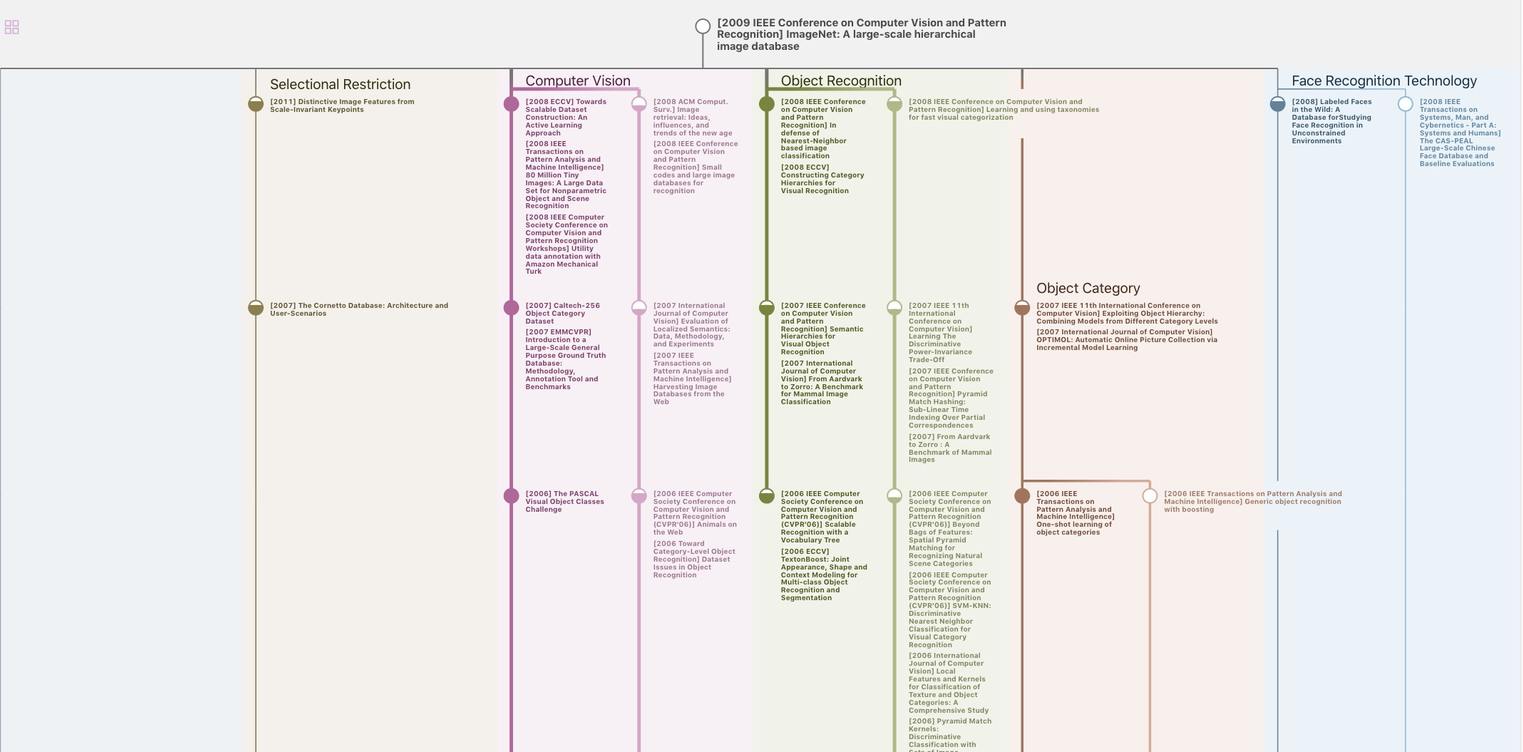
生成溯源树,研究论文发展脉络
Chat Paper
正在生成论文摘要