A nonlinear conjugate gradient method with complexity guarantees and its application to nonconvex regression
EURO Journal on Computational Optimization(2022)
摘要
Nonlinear conjugate gradients are among the most popular techniques for solving continuous optimization problems. Although these schemes have long been studied from a global convergence standpoint, their worst-case complexity properties have yet to be fully understood, especially in the nonconvex setting. In particular, it is unclear whether nonlinear conjugate gradient methods possess better guarantees than first-order methods such as gradient descent. Meanwhile, recent experiments have shown impressive performance of standard nonlinear conjugate gradient techniques on certain nonconvex problems, even when compared with methods endowed with the best known complexity guarantees.
更多查看译文
关键词
nonlinear conjugate,gradient method,regression,complexity guarantees
AI 理解论文
溯源树
样例
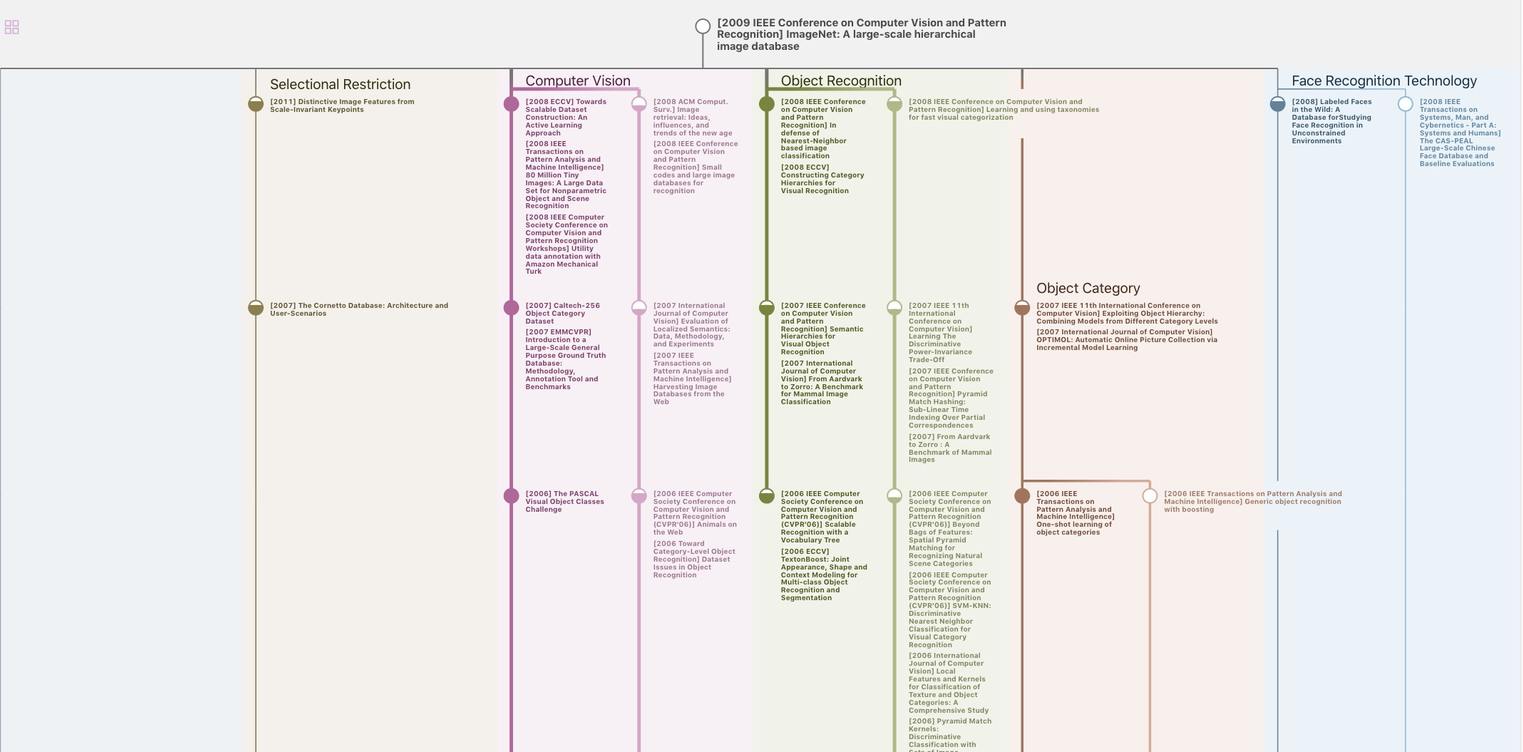
生成溯源树,研究论文发展脉络
Chat Paper
正在生成论文摘要