Spatiotemporal analysis using Riemannian composition of diffusion operators
APPLIED AND COMPUTATIONAL HARMONIC ANALYSIS(2024)
摘要
Multivariate time-series have become abundant in recent years, as many data acquisition systems record information through multiple sensors simultaneously. In this paper, we assume the variables pertain to some geometry and present an operator-based approach for spatiotemporal analysis. Our approach combines three components that are often considered separately: (i) manifold learning for building operators representing the geometry of the variables, (ii) Riemannian geometry of symmetric positive-definite matrices for multiscale composition of operators corresponding to different time samples, and (iii) spectral analysis of the composite operators for extracting different dynamic modes. We propose a method that is analogous to the classical wavelet analysis, which we term Riemannian multi resolution analysis (RMRA). We provide some theoretical results on the spectral analysis of the composite operators, and we demonstrate the proposed method on simulations and on real data.& COPY; 2023 The Author(s). Published by Elsevier Inc. This is an open access article under the CC BY-NC-ND license (http:// creativecommons .org /licenses /by -nc -nd /4 .0/).
更多查看译文
关键词
Manifold learning,Diffusion maps,Riemannian geometry,Symmetric positive-definite matrices
AI 理解论文
溯源树
样例
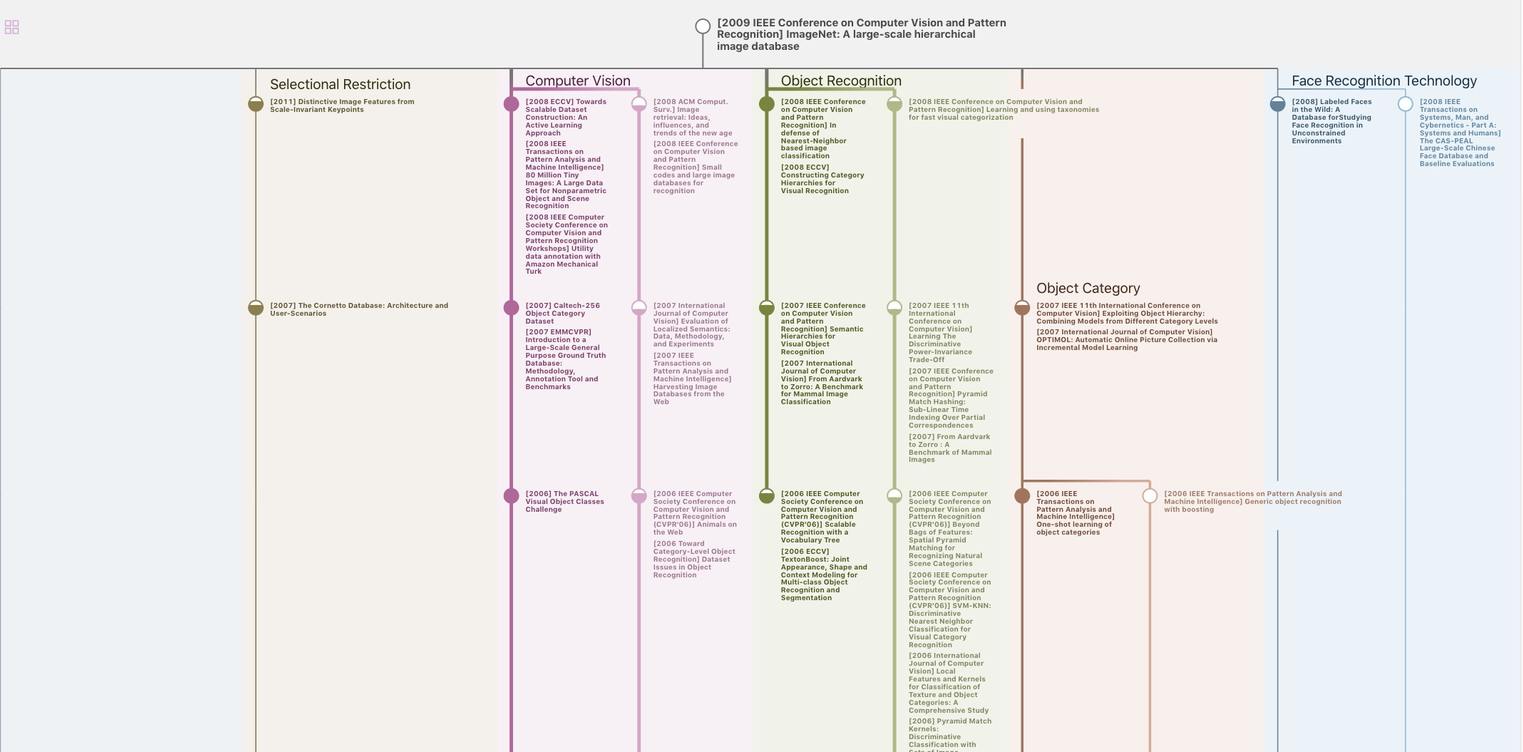
生成溯源树,研究论文发展脉络
Chat Paper
正在生成论文摘要