An Evaluation of Pretrained BERT Models for Comparing Semantic Similarity Across Unstructured Clinical Trial Texts.
ICIMTH(2021)
摘要
Processing unstructured clinical texts is often necessary to support certain tasks in biomedicine, such as matching patients to clinical trials. Among other methods, domain-specific language models have been built to utilize free-text information. This study evaluated the performance of Bidirectional Encoder Representations from Transformers (BERT) models in assessing the similarity between clinical trial texts. We compared an unstructured aggregated summary of clinical trials reviewed at the Johns Hopkins Molecular Tumor Board with the ClinicalTrials.gov records, focusing on the titles and eligibility criteria. Seven pretrained BERT-Based models were used in our analysis. Of the six biomedical-domain-specific models, only SciBERT outperformed the original BERT model by accurately assigning higher similarity scores to matched than mismatched trials. This finding is promising and shows that BERT and, likely, other language models may support patient-trial matching.
更多查看译文
关键词
Clinical trial,bidirectional coder representations,word embeddings
AI 理解论文
溯源树
样例
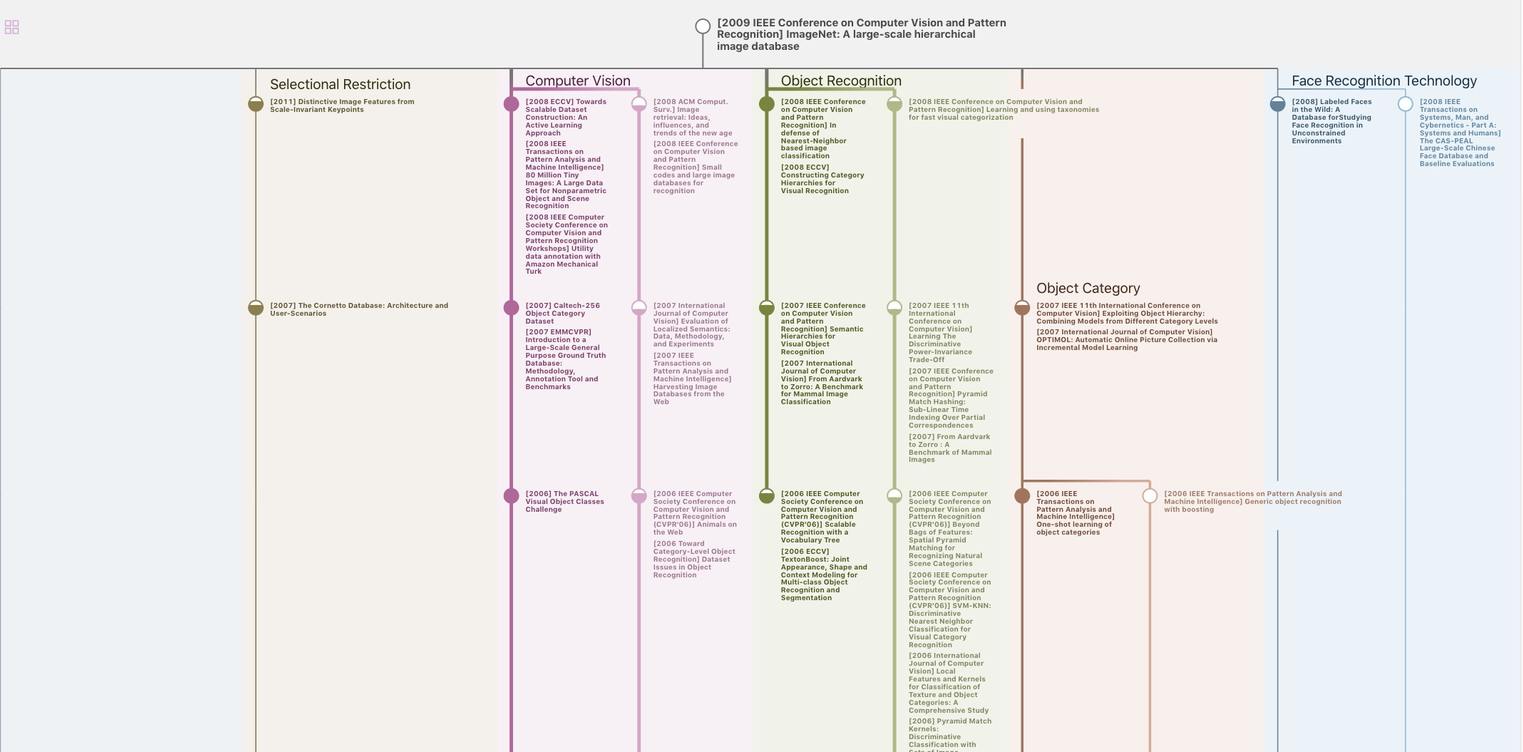
生成溯源树,研究论文发展脉络
Chat Paper
正在生成论文摘要