Radiomics-Based Detection of Radionecrosis Using Harmonized Multiparametric MRI
CANCERS(2022)
摘要
Simple Summary Within a multicentric clinical trial context for the treatment of recurrent high-grade brain tumors, the aim of our study was to assess the potential added value of multiparametric MRI harmonization to improve a classification problem based on radiomics analysis. We confirmed that harmonization reduced the so-called "scanner effect" related to the variability of multiparametric MRI protocol settings between the participating centers and improved the predictive performance of radiomics-based classification model. Radiomics features extracted from MRI perfusion gave the best accuracy for the classification between radionecrosis and tumor progression. Interestingly, our study revealed that radiomics features extracted from T1-weigthed MRI alone, before any injection of contrast product reached accuracies close to the perfusion model. In this study, a radiomics analysis was conducted to provide insights into the differentiation of radionecrosis and tumor progression in multiparametric MRI in the context of a multicentric clinical trial. First, the sensitivity of radiomic features to the unwanted variability caused by different protocol settings was assessed for each modality. Then, the ability of image normalization and ComBat-based harmonization to reduce the scanner-related variability was evaluated. Finally, the performances of several radiomic models dedicated to the classification of MRI examinations were measured. Our results showed that using radiomic models trained on harmonized data achieved better predictive performance for the investigated clinical outcome (balanced accuracy of 0.61 with the model based on raw data and 0.72 with ComBat harmonization). A comparison of several models based on information extracted from different MR modalities showed that the best classification accuracy was achieved with a model based on MR perfusion features in conjunction with clinical observation (balanced accuracy of 0.76 using LASSO feature selection and a Random Forest classifier). Although multimodality did not provide additional benefit in predictive power, the model based on T1-weighted MRI before injection provided an accuracy close to the performance achieved with perfusion.
更多查看译文
关键词
radiomics analysis, multicenter harmonization, multiparametric MRI, radiation-induced necrosis
AI 理解论文
溯源树
样例
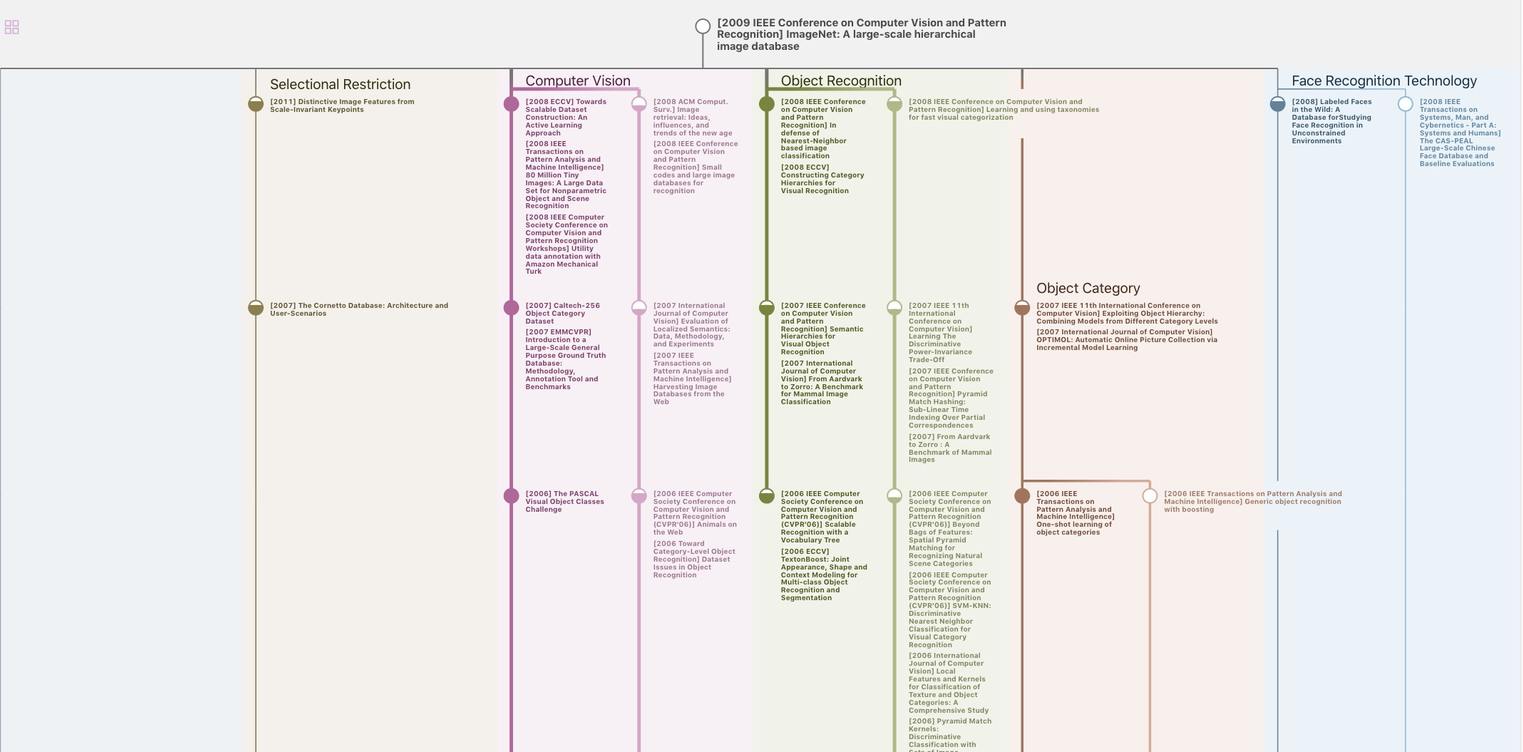
生成溯源树,研究论文发展脉络
Chat Paper
正在生成论文摘要