A Smoothed Version of the Lassosum Penalty for Fitting Integrated Risk Models Using Summary Statistics or Individual-Level Data
GENES(2022)
摘要
Polygenic risk scores are a popular means to predict the disease risk or disease susceptibility of an individual based on its genotype information. When adding other important epidemiological covariates such as age or sex, we speak of an integrated risk model. Methodological advances for fitting more accurate integrated risk models are of immediate importance to improve the precision of risk prediction, thereby potentially identifying patients at high risk early on when they are still able to benefit from preventive steps/interventions targeted at increasing their odds of survival, or at reducing their chance of getting a disease in the first place. This article proposes a smoothed version of the "Lassosum" penalty used to fit polygenic risk scores and integrated risk models using either summary statistics or raw data. The smoothing allows one to obtain explicit gradients everywhere for efficient minimization of the Lassosum objective function while guaranteeing bounds on the accuracy of the fit. An experimental section on both Alzheimer's disease and COPD (chronic obstructive pulmonary disease) demonstrates the increased accuracy of the proposed smoothed Lassosum penalty compared to the original Lassosum algorithm (for the datasets under consideration), allowing it to draw equal with state-of-the-art methodology such as LDpred2 when evaluated via the AUC (area under the ROC curve) metric.
更多查看译文
关键词
integrated risk model, lassosum, nesterov, polygenic risk scores, smoothing
AI 理解论文
溯源树
样例
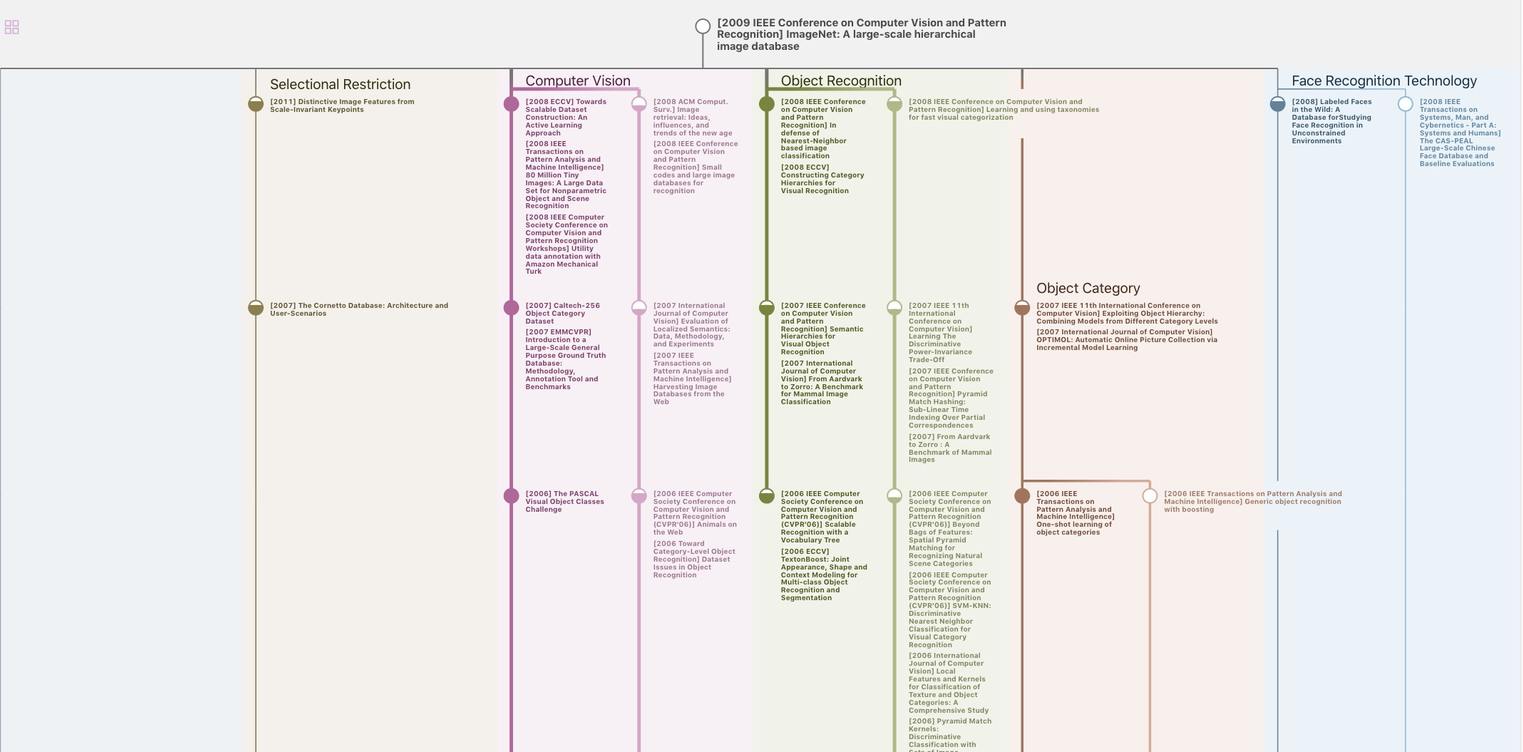
生成溯源树,研究论文发展脉络
Chat Paper
正在生成论文摘要