Improving Small Molecule pK(a) Prediction Using Transfer Learning With Graph Neural Networks
FRONTIERS IN CHEMISTRY(2022)
摘要
Enumerating protonation states and calculating microstate pK(a) values of small molecules is an important yet challenging task for lead optimization and molecular modeling. Commercial and non-commercial solutions have notable limitations such as restrictive and expensive licenses, high CPU/GPU hour requirements, or the need for expert knowledge to set up and use. We present a graph neural network model that is trained on 714,906 calculated microstate pK(a) predictions from molecules obtained from the ChEMBL database. The model is fine-tuned on a set of 5,994 experimental pK(a) values significantly improving its performance on two challenging test sets. Combining the graph neural network model with Dimorphite-DL, an open-source program for enumerating ionization states, we have developed the open-source Python package pkasolver, which is able to generate and enumerate protonation states and calculate pK(a) values with high accuracy.
更多查看译文
关键词
physical properties, PKA, Graph Neural Network (GNN), transfer learning, protonation states
AI 理解论文
溯源树
样例
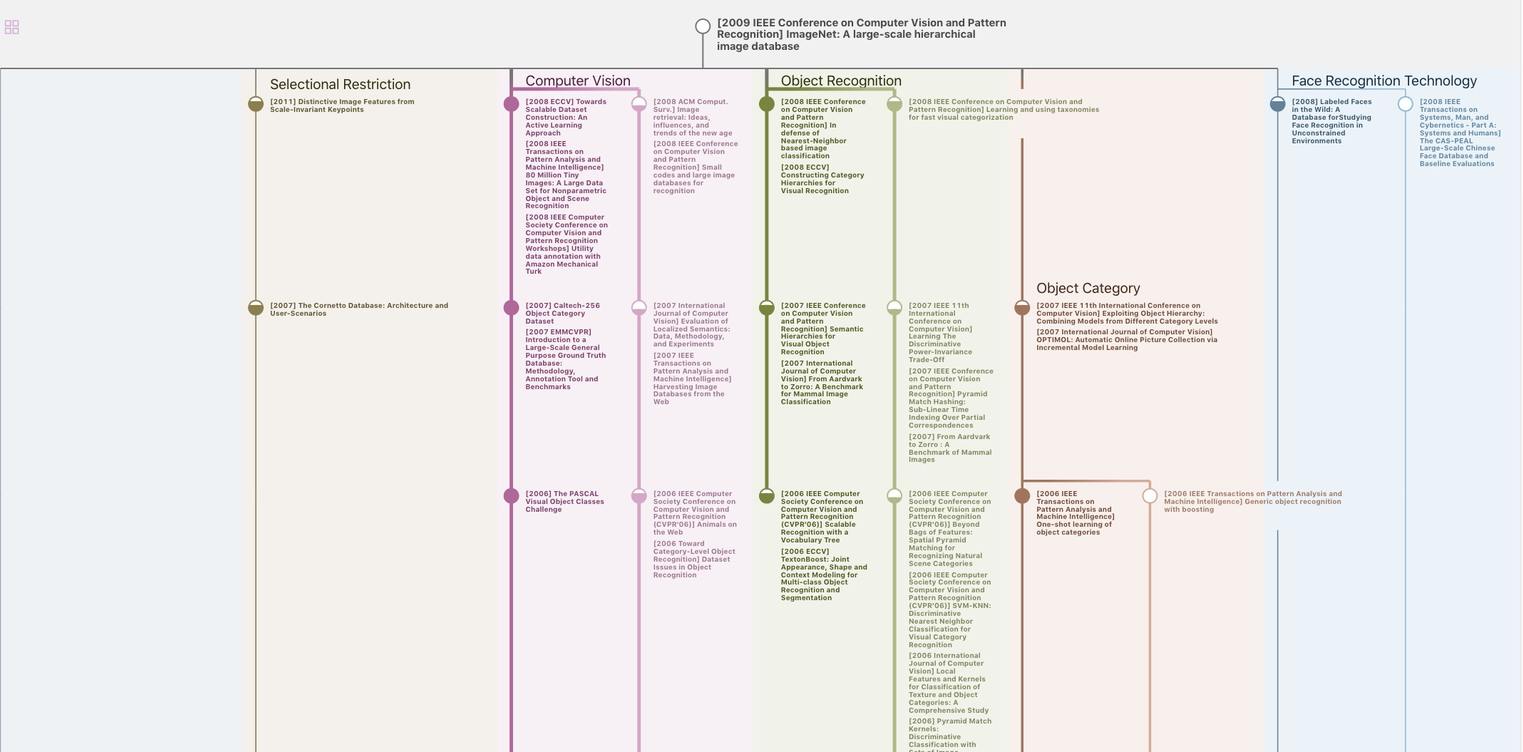
生成溯源树,研究论文发展脉络
Chat Paper
正在生成论文摘要