Machine Learning Applied to Omics Datasets Predicts Mortality in Patients with Alcoholic Hepatitis
METABOLITES(2022)
摘要
Alcoholic hepatitis is a major health care burden in the United States due to significant morbidity and mortality. Early identification of patients with alcoholic hepatitis at greatest risk of death is extremely important for proper treatments and interventions to be instituted. In this study, we used gradient boosting, random forest, support vector machine and logistic regression analysis of laboratory parameters, fecal bacterial microbiota, fecal mycobiota, fecal virome, serum metabolome and serum lipidome to predict mortality in patients with alcoholic hepatitis. Gradient boosting achieved the highest AUC of 0.87 for both 30-day mortality prediction using the bacteria and metabolic pathways dataset and 90-day mortality prediction using the fungi dataset, which showed better performance than the currently used model for end-stage liver disease (MELD) score.
更多查看译文
关键词
machine learning, mycobiome, virome, microbiota, metabolomics
AI 理解论文
溯源树
样例
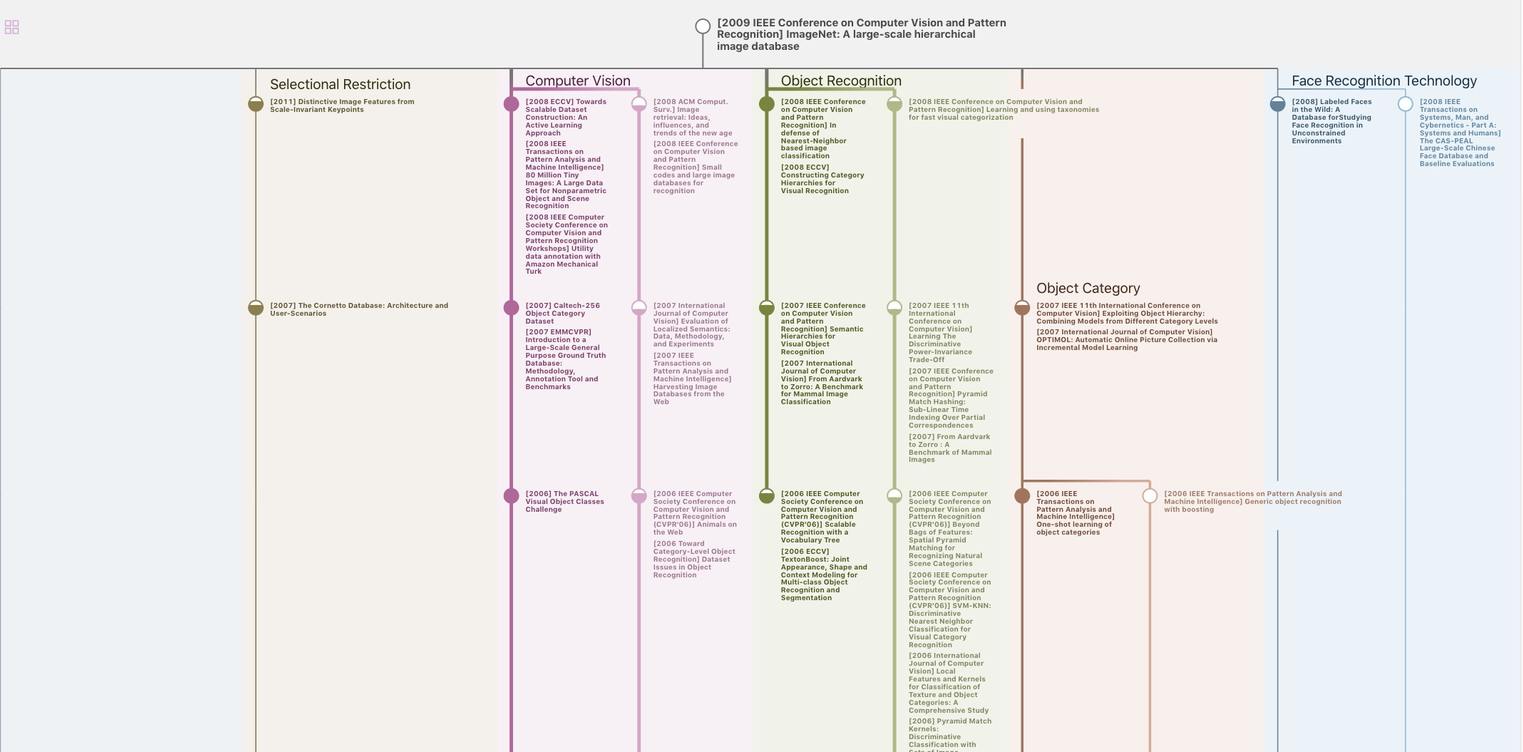
生成溯源树,研究论文发展脉络
Chat Paper
正在生成论文摘要