Deep Learning in Prediction of Late Major Bleeding After Transcatheter Aortic Valve Replacement
CLINICAL EPIDEMIOLOGY(2022)
摘要
Purpose: Late major bleeding is one of the main complications after transcatheter aortic valve replacement (TAVR). We aimed to develop a risk prediction model based on deep learning to predict major or life-threatening bleeding complications (MLBCs) after TAVR. Patients and Methods: This was a retrospective study including TAVR patients from West China Hospital of Sichuan University Transcatheter Aortic Valve Replacement Registry (ChiCTR2000033419) between April 17, 2012 and May 27, 2020. A deep learning-based model named BLeNet was developed with 56 features covering baseline, procedural, and post-procedural characteristics. The model was validated with the bootstrap method and evaluated using Harrell's concordance index (c-index), receiver operating characteristics (ROC) curve, calibration curve, and Kaplan-Meier estimate. Captum interpretation library was applied to identify feature importance. The BLeNet model was compared with the traditional Cox proportional hazard (Cox-PH) model and the random survival forest model in the metrics mentioned above. Results: The BLeNet model outperformed the Cox-PH and random survival forest models significantly in discrimination [optimism-corrected c-index of BLeNet vs Cox-PH vs random survival forest: 0.81 (95% CI: 0.79-0.92) vs 0.72 (95% CI: 0.63-0.77) vs 0.70 (95% CI: 0.61-0.74)] and calibration (integrated calibration index of BLeNet vs Cox-PH vs random survival forest: 0.007 vs 0.015 vs 0.019). In Kaplan-Meier analysis, BLeNet model had great performance in stratifying high-and low-bleeding risk patients (p < 0.0001). Conclusion: Deep learning is a feasible way to build prediction models concerning TAVR prognosis. A dedicated bleeding risk prediction model was developed for TAVR patients to facilitate well-informed clinical decisions.
更多查看译文
关键词
deep learning, transcatheter aortic valve replacement, major or life-threatening bleeding complications, prediction model
AI 理解论文
溯源树
样例
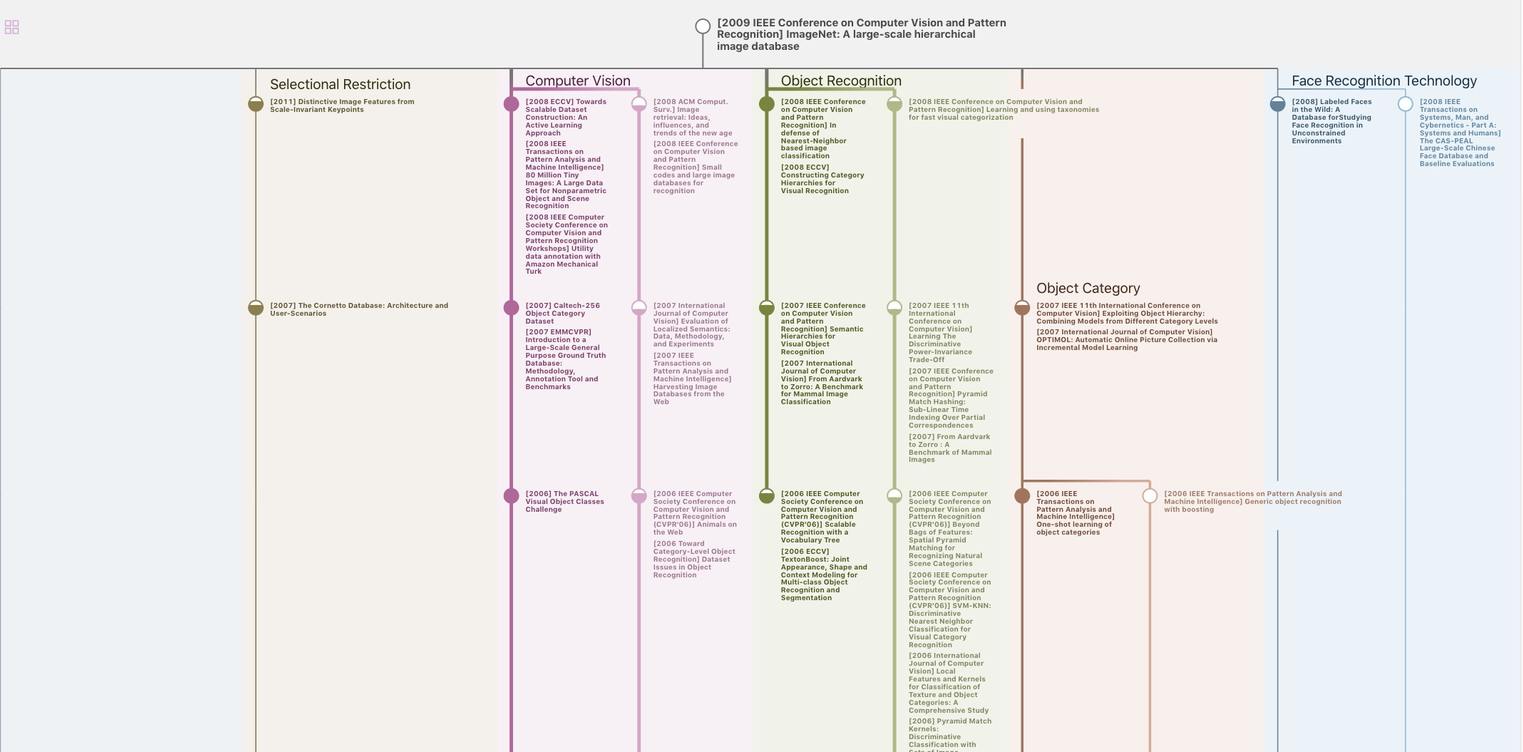
生成溯源树,研究论文发展脉络
Chat Paper
正在生成论文摘要