Identifying interactions in omics data for clinical biomarker discovery
biorxiv(2022)
摘要
The identification of predictive biomarker signatures from omics data for clinical applications is an active area of research. Recent developments in assay technologies and machine learning (ML) methods have led to significant improvements in predictive performance. However, most high-performing ML suffer from complex architectures and lack interpretability. Here, we present the application of a novel symbolic-regression-based method, the QLattice, on a selection of clinical omics data sets. This approach identifies putative regulatory interactions between biomolecules and generates parsimonious high-performing models that can both predict and explain the outcome of a given omics experiment. Due to their simplicity and explicit functional form, the models can be easily interpreted and have the potential to facilitate the discovery of new biomarker signatures.
### Competing Interest Statement
The authors are employed at Abzu, developers of the QLattice. The QLattice is freely available for non-commercial use.
更多查看译文
关键词
omics data
AI 理解论文
溯源树
样例
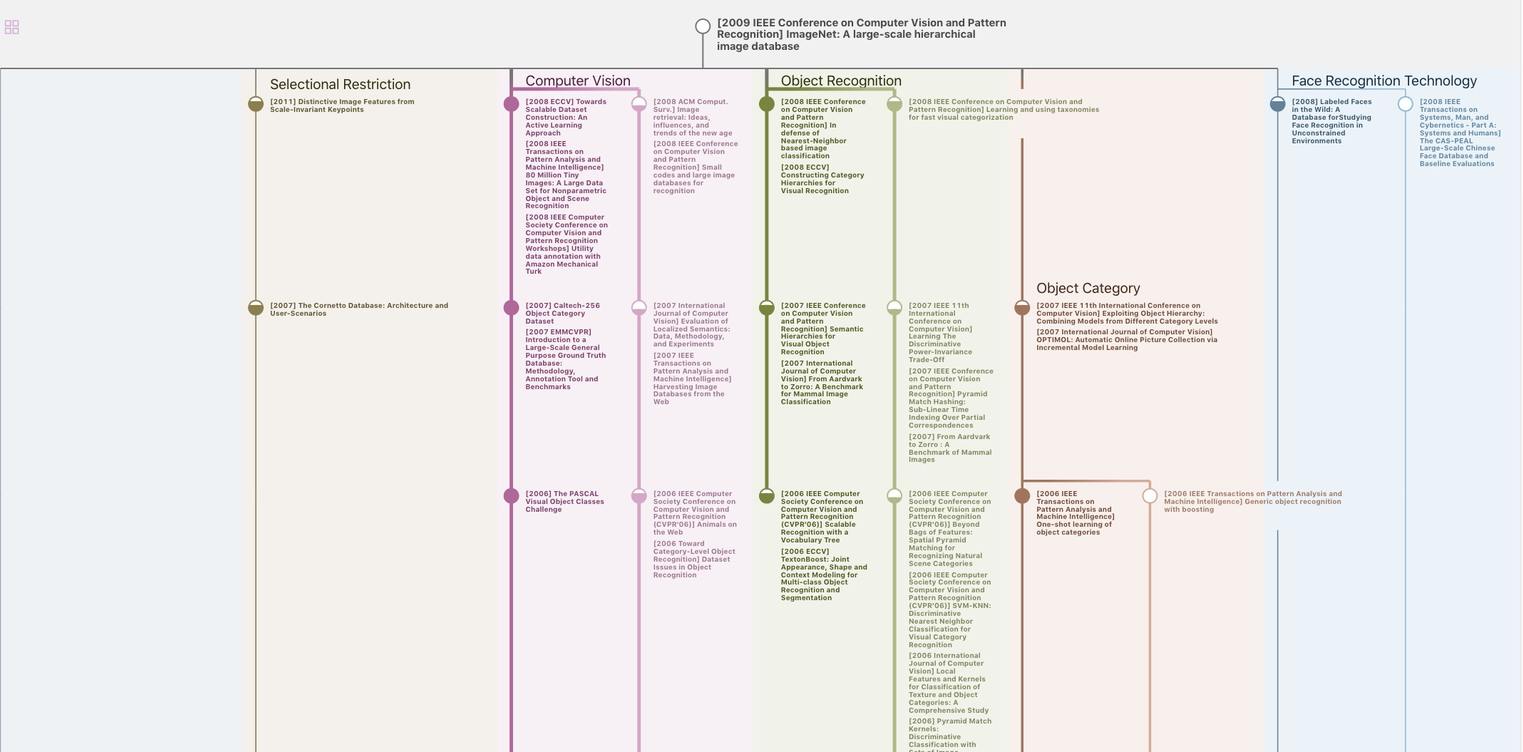
生成溯源树,研究论文发展脉络
Chat Paper
正在生成论文摘要