Learning Pixel Trajectories with Multiscale Contrastive Random Walks
IEEE Conference on Computer Vision and Pattern Recognition(2022)
摘要
A range of video modeling tasks, from optical flow to multiple object tracking, share the same fundamental challenge: establishing space-time correspondence. Yet, approaches that dominate each space differ. We take a step to-wards bridging this gap by extending the recent contrastive random walk formulation to much denser, pixel-level spacetime graphs. The main contribution is introducing hierarchy into the search problem by computing the transition matrix between two frames in a coarse-to-fine manner, forming a multiscale contrastive random walk when ex-tended in time. This establishes a unified technique for self-supervised learning of optical flow, keypoint tracking, and video object segmentation. Experiments demonstrate that, for each of these tasks, the unified model achieves performance competitive with strong self-supervised approaches specific to that task. 1 1 Project page at https://jasonbian97.github.io/flowwalk
更多查看译文
关键词
Motion and tracking
AI 理解论文
溯源树
样例
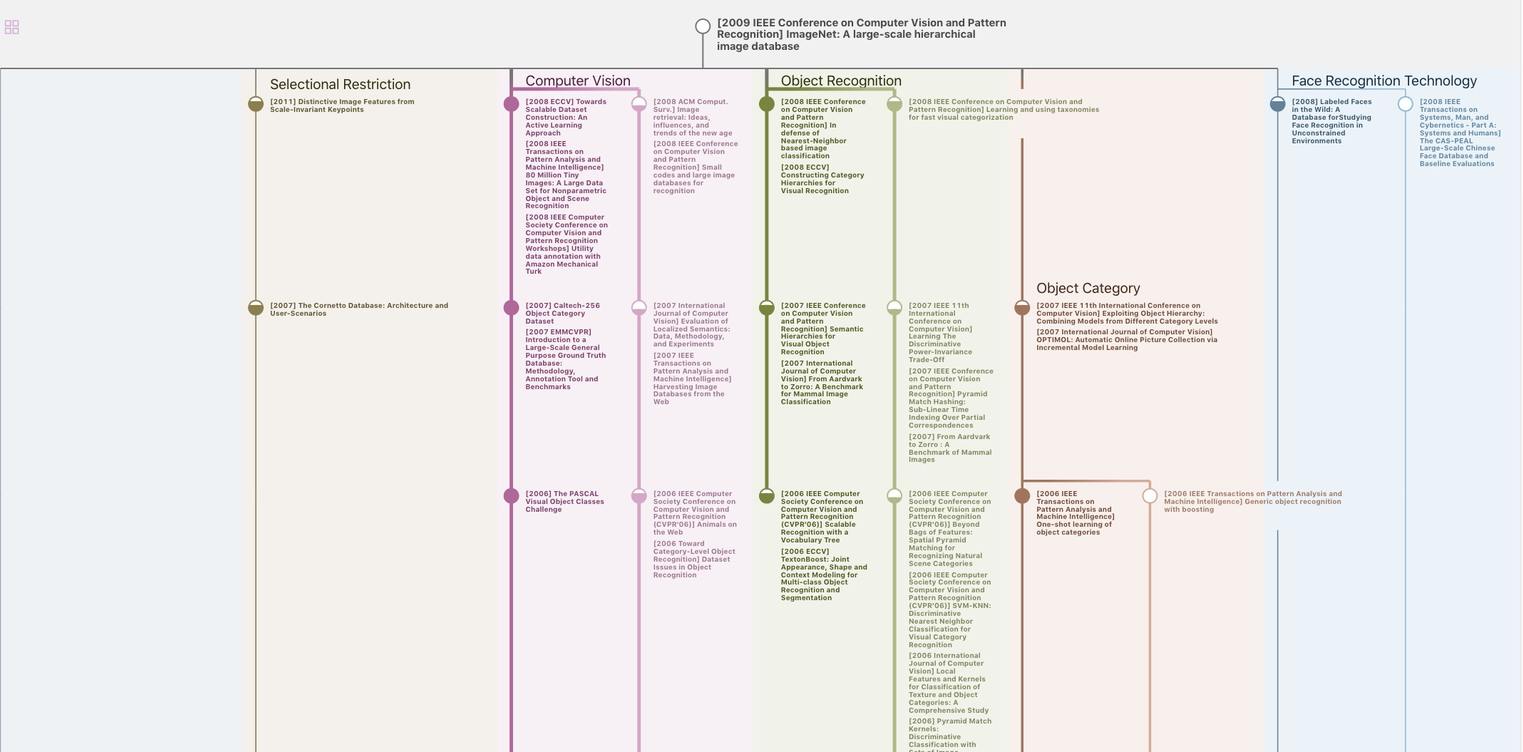
生成溯源树,研究论文发展脉络
Chat Paper
正在生成论文摘要