DRTCI: Learning Disentangled Representations for Temporal Causal Inference
arxiv(2022)
摘要
Medical professionals evaluating alternative treatment plans for a patient often encounter time varying confounders, or covariates that affect both the future treatment assignment and the patient outcome. The recently proposed Counterfactual Recurrent Network (CRN) accounts for time varying confounders by using adversarial training to balance recurrent historical representations of patient data. However, this work assumes that all time varying covariates are confounding and thus attempts to balance the full state representation. Given that the actual subset of covariates that may in fact be confounding is in general unknown, recent work on counterfactual evaluation in the static, non-temporal setting has suggested that disentangling the covariate representation into separate factors, where each either influence treatment selection, patient outcome or both can help isolate selection bias and restrict balancing efforts to factors that influence outcome, allowing the remaining factors which predict treatment without needlessly being balanced.
更多查看译文
关键词
temporal causal
AI 理解论文
溯源树
样例
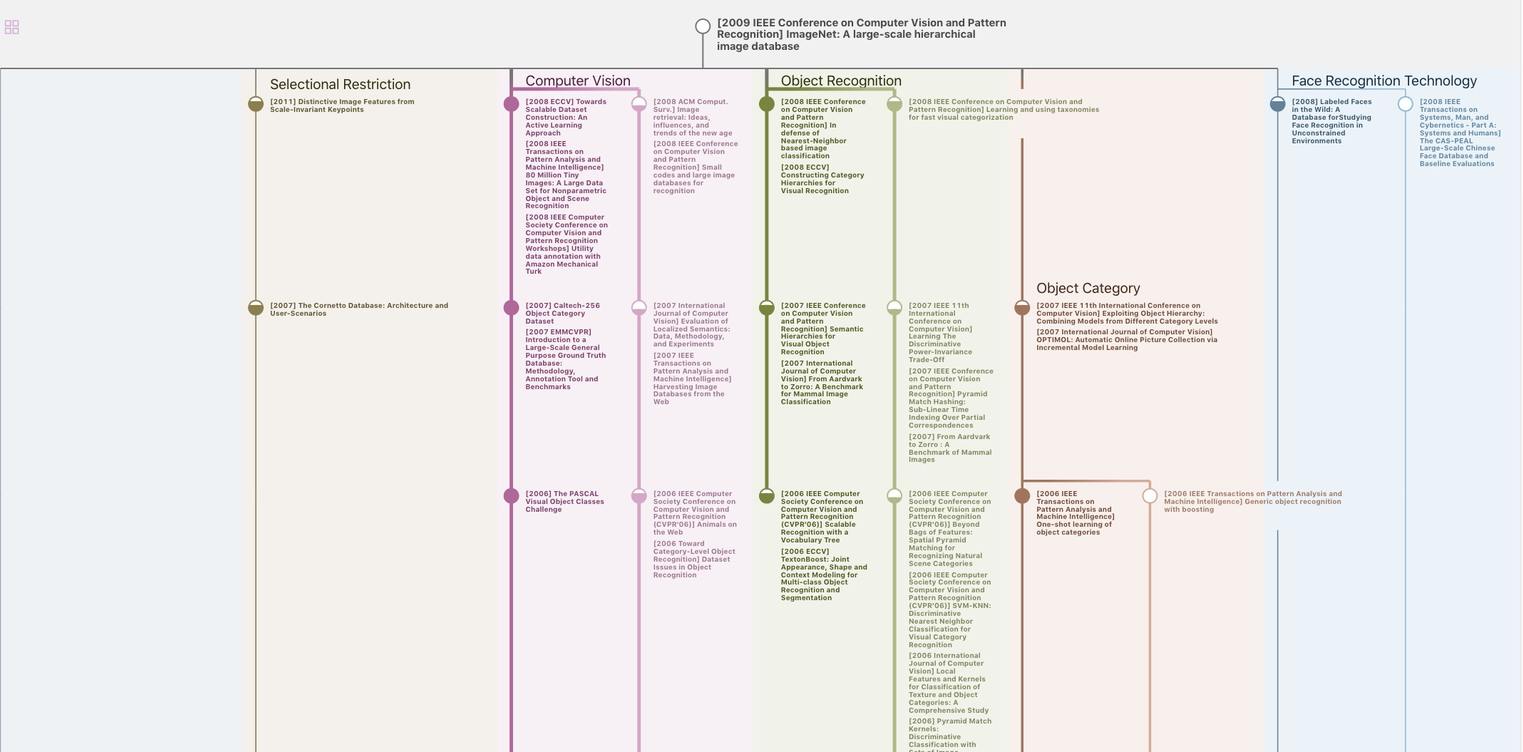
生成溯源树,研究论文发展脉络
Chat Paper
正在生成论文摘要