High-fidelity 3D Model Compression based on Key Spheres
2022 Data Compression Conference (DCC)(2022)
摘要
In recent years, neural signed distance function (SDF) has become one of the most effective representation methods for 3D models. By learning continuous SDFs in 3D space, neural networks can predict the distance from a given query space point to its closest object surface, whose positive and negative signs denote inside and outside of the object, respectively. Training a specific network for each 3D model, which individually embeds its shape, can realize compressed representation of objects by storing fewer network (and possibly latent) parameters. Consequently, reconstruction through network inference and surface recovery can be achieved. In this paper, we propose an SDF prediction network using explicit key spheres as input. Key spheres are extracted from the internal space of objects, whose centers either have relatively larger SDF values (sphere radii), or are located at essential positions. By inputting the spatial information of multiple spheres which imply different local shapes, the proposed method can significantly improve the reconstruction accuracy with a negligible storage cost. Compared to previous works, our method achieves the high-fidelity and high-compression 3D object coding and reconstruction. Experiments conducted on three datasets verify the superior performance of our method.
更多查看译文
关键词
high-fidelity 3D model compression,distance function,effective representation methods,continuous SDFs,neural networks,given query space point,closest object surface,positive signs,negative signs,specific network,fewer network,and possibly latent,network inference,surface recovery,SDF prediction network,explicit key spheres,relatively larger SDF values,sphere radii,essential positions,multiple spheres,different local shapes,high-compression
AI 理解论文
溯源树
样例
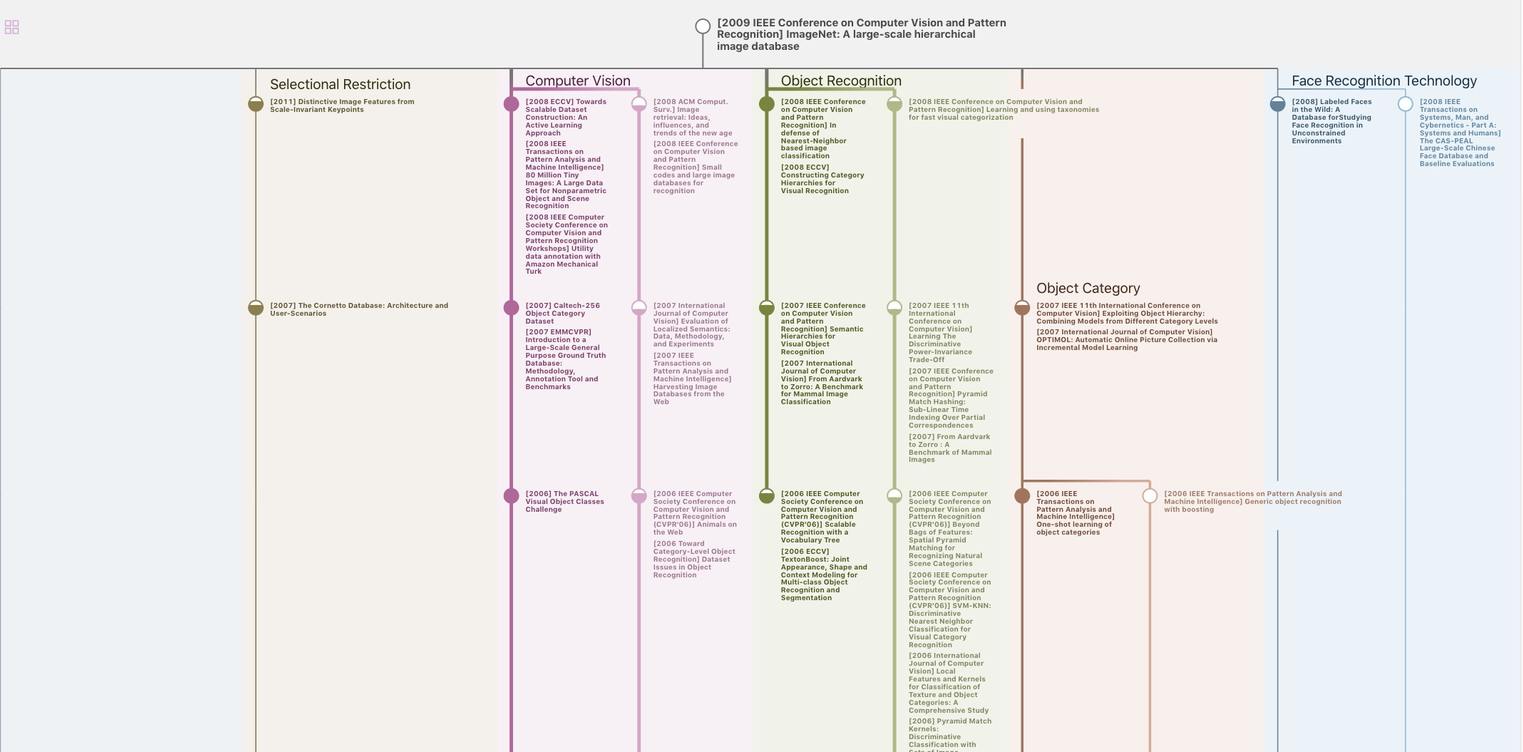
生成溯源树,研究论文发展脉络
Chat Paper
正在生成论文摘要