Interpretable Learned Emergent Communication for Human–Agent Teams
arxiv(2023)
摘要
Learning interpretable communication is essential for multiagent and human–agent teams (HATs). In multiagent reinforcement learning for partially observable environments, agents may convey information to others via learned communication, allowing the team to complete its task. Inspired by human languages, recent works study discrete (using only a finite set of tokens) and sparse (communicating only at some time-steps) communication. However, the utility of such communication in HAT experiments has not yet been investigated. In this work, we analyze the efficacy of sparse–discrete methods for producing emergent communication that enables high agent-only and HAT performance. We develop agent-only teams that communicate sparsely via our scheme of Enforcers that sufficiently constrain communication to any budget. Our results show no loss or minimal loss of performance in benchmark environments and tasks. In HATs tested in benchmark environments, where agents have been modeled using the Enforcers, we find that a prototype-based method produces meaningful discrete tokens that enable human partners to learn agent communication faster and better than a one-hot baseline. Additional HAT experiments show that an appropriate sparsity level lowers the cognitive load of humans when communicating with teams of agents and leads to superior team performance.
更多查看译文
关键词
Autonomous thinking behaviors through development,emergent communication,human–agent teams (HATs),multiagent reinforcement learning (MARL),neural networks for development
AI 理解论文
溯源树
样例
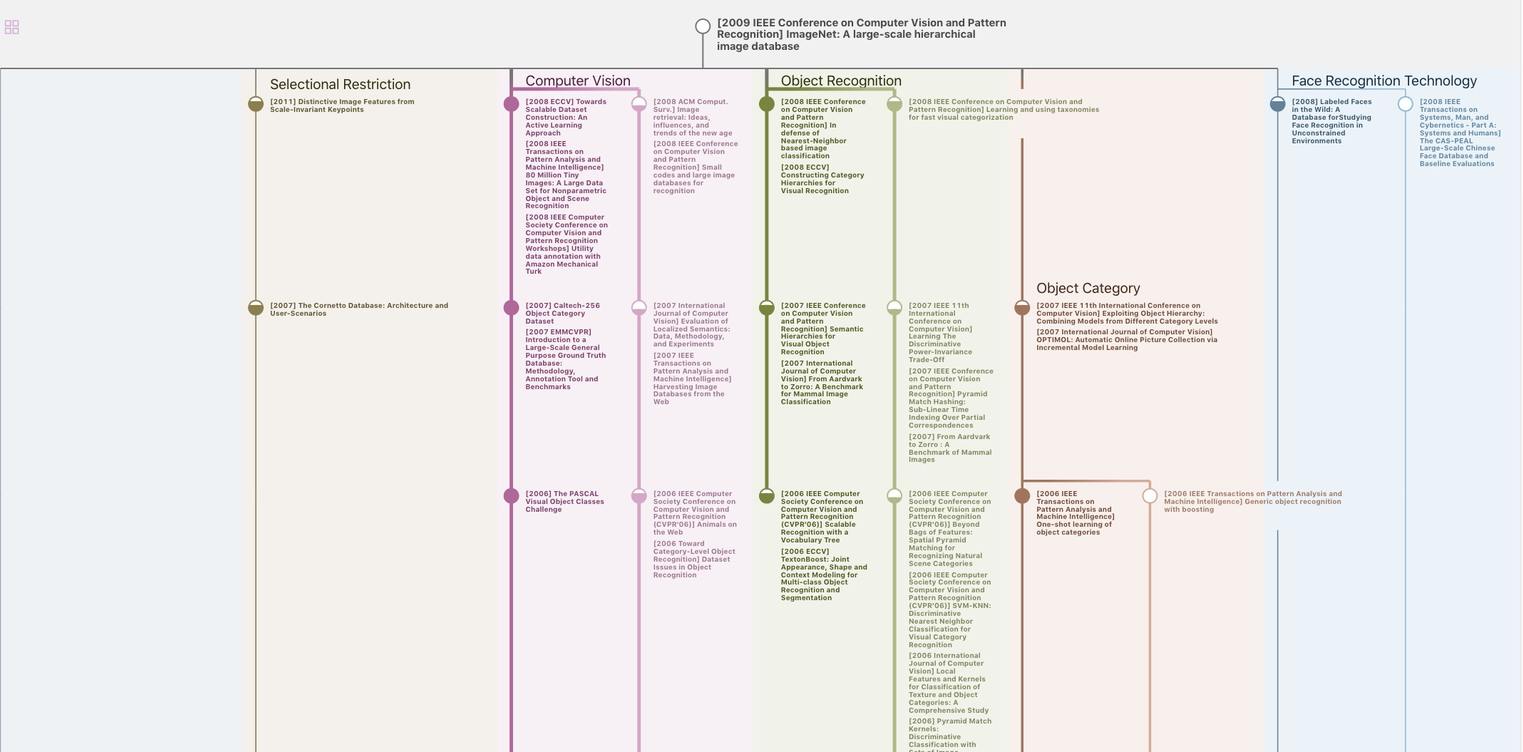
生成溯源树,研究论文发展脉络
Chat Paper
正在生成论文摘要