Learning Tensor Representations for Meta-Learning
INTERNATIONAL CONFERENCE ON ARTIFICIAL INTELLIGENCE AND STATISTICS, VOL 151(2022)
摘要
We introduce a tensor-based model of shared representation for meta-learning from a diverse set of tasks. Prior works on learning linear representations for meta-learning assume that there is a common shared representation across different tasks, and do not consider the additional task-specific observable side information. In this work, we model the meta-parameter through an order-3 tensor, which can adapt to the observed task features of the task. We propose two methods to estimate the underlying tensor. The first method solves a tensor regression problem and works under natural assumptions on the data generating process. The second method uses the method of moments under additional distributional assumptions and has an improved sample complexity in terms of the number of tasks. We also focus on the meta-test phase, and consider estimating task-specific parameters on a new task. Substituting the estimated tensor from the first step allows us estimating the task-specific parameters with very few samples of the new task, thereby showing the benefits of learning tensor representations for meta-learning. Finally, through simulation and several real-world datasets, we evaluate our methods and show that it improves over previous linear models of shared representations for meta-learning.
更多查看译文
关键词
tensor representations,meta-learning
AI 理解论文
溯源树
样例
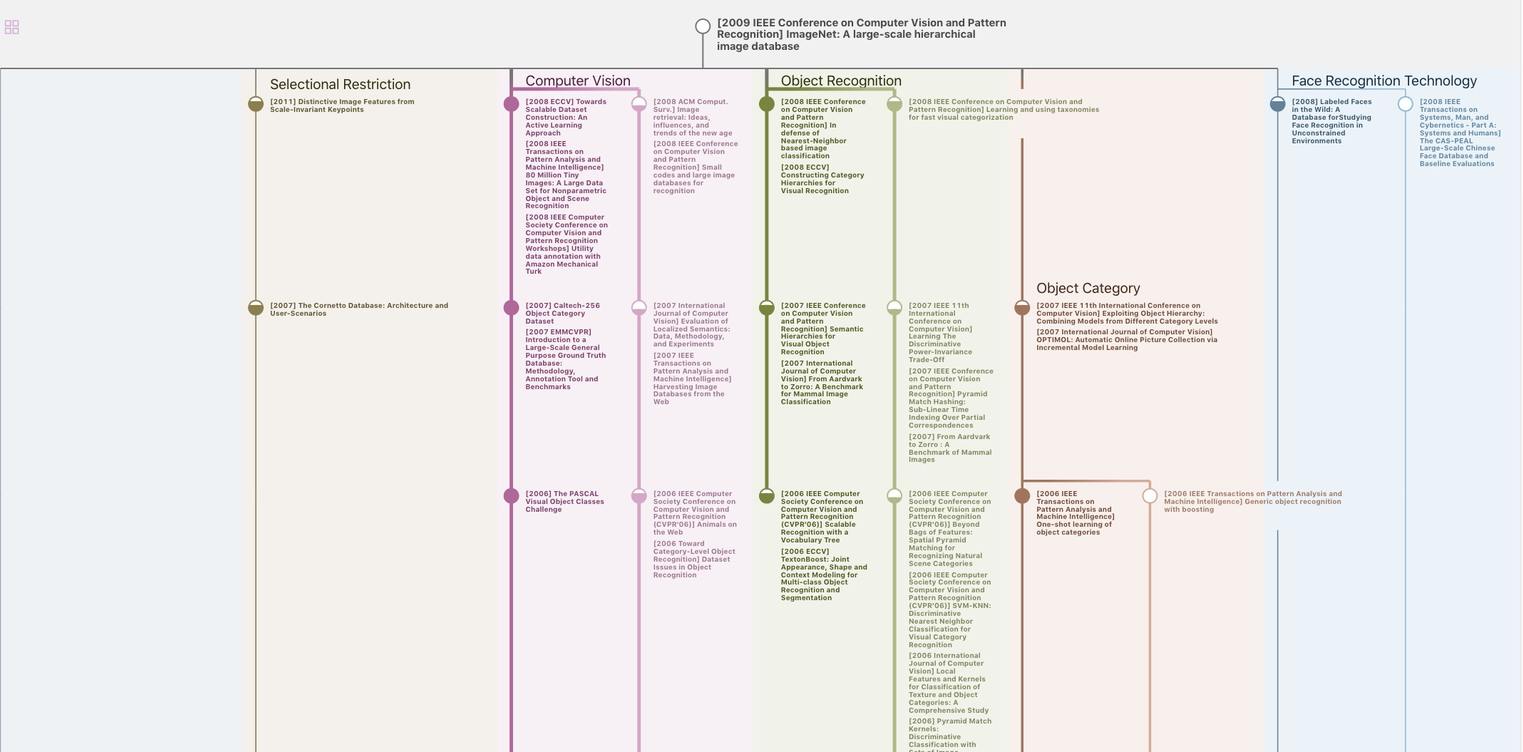
生成溯源树,研究论文发展脉络
Chat Paper
正在生成论文摘要