Online MCMC Thinning with Kernelized Stein Discrepancy
SIAM JOURNAL ON MATHEMATICS OF DATA SCIENCE(2024)
摘要
A fundamental challenge in Bayesian inference is efficient representation of a target distribution. Many nonparametric approaches do so by sampling a large number of points using variants of Markov chain Monte Carlo (MCMC). We propose an MCMC variant that retains only those posterior samples which exceed a kernelized Stein discrepancy (KSD) threshold, which we call KSD thinning. We establish the convergence and complexity trade-offs for several settings of KSD thinning as a function of the KSD threshold parameter, sample size, and other problem parameters. We provide experimental comparisons against other online nonparametric Bayesian methods that generate low -complexity posterior representations. We observe superior consistency/complexity trade-offs across a range of settings including MCMC sampling on two Bayesian inference problems from the biological sciences, and 10\times inference speedup and storage reduction for Bayesian neural networks with no loss of accuracy and no increase in training time. Our code is available at https://github.com/colehawkins/KSD-Thinning.
更多查看译文
关键词
Bayesian inference,online thinning,Stein discrepancy,Bayesian neural network
AI 理解论文
溯源树
样例
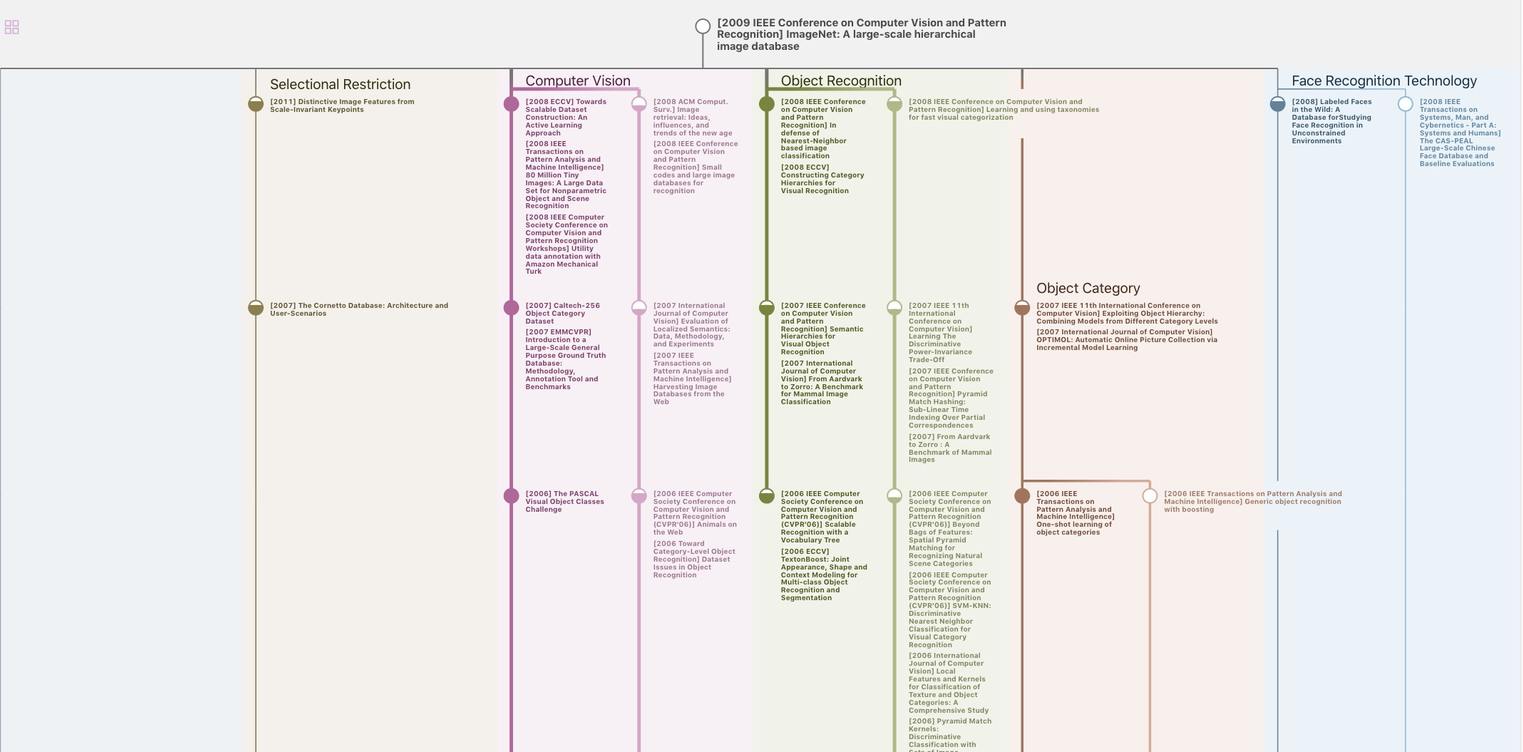
生成溯源树,研究论文发展脉络
Chat Paper
正在生成论文摘要