Comparison of Bayesian and particle swarm algorithms for hyperparameter optimisation in machine learning applications in high energy physics
COMPUTER PHYSICS COMMUNICATIONS(2024)
摘要
When using machine learning (ML) techniques, users typically need to choose a plethora of algorithm-specific parameters, referred to as hyperparameters. In this paper, we compare the performance of two algorithms, particle swarm optimisation (PSO) and Bayesian optimisation (BO), for the autonomous determination of these hyperparameters in applications to different ML tasks typical for the fiel d of high energ y physics (HEP). Ou r evaluation of the performance includes a comparison of the capability of the PSO and BO algorithms to make efficient use of the highly parallel computing resources that are characteristic of contemporar y H E P experiments.
更多查看译文
关键词
Hyperparameter optimization,High energy physics,Evolutionary algorith m s,Machine learning
AI 理解论文
溯源树
样例
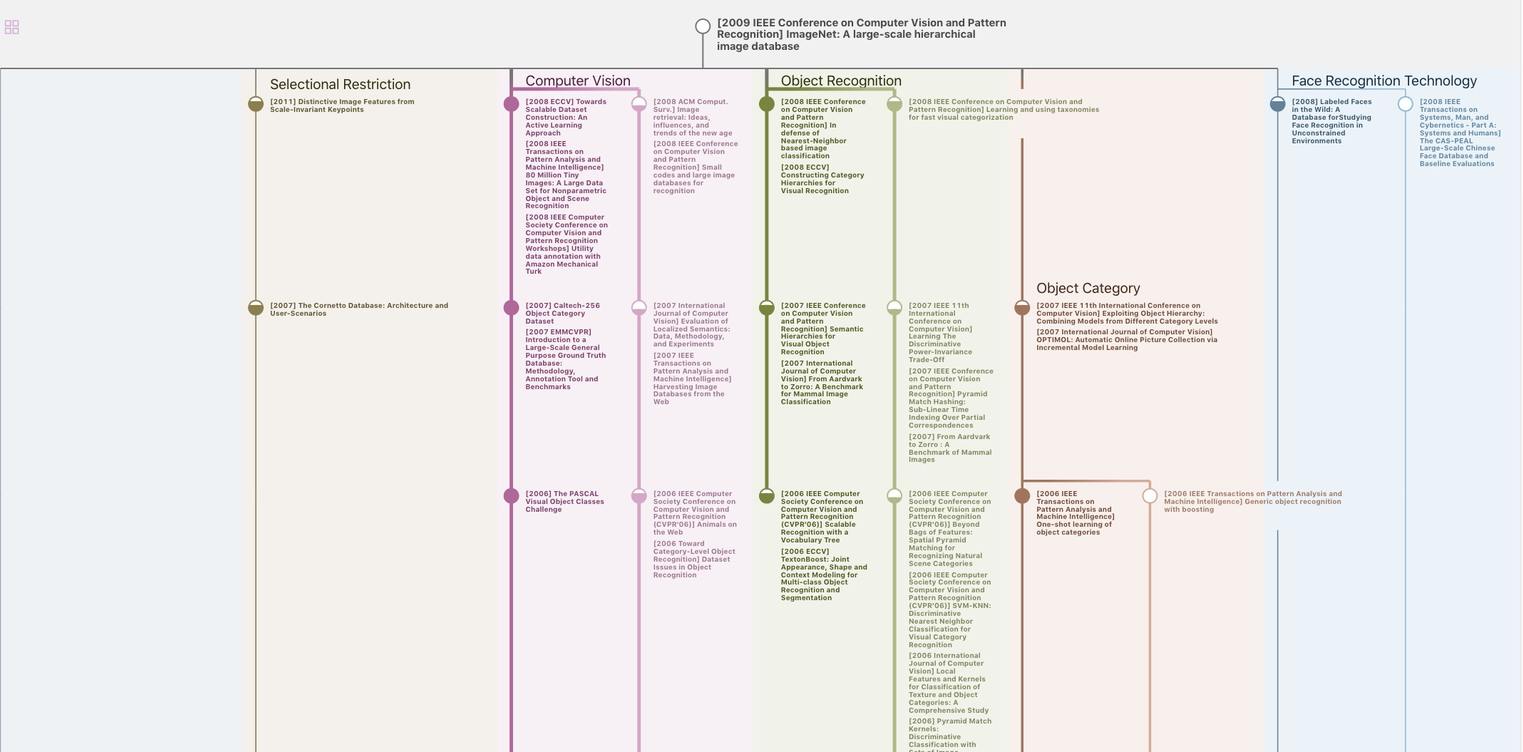
生成溯源树,研究论文发展脉络
Chat Paper
正在生成论文摘要