On the Linear Convergence of Extra-Gradient Methods for Nonconvex-Nonconcave Minimax Problems
arxiv(2023)
摘要
Recently, minimax optimization received renewed focus due to modern applications in machine learning, robust optimization, and reinforcement learning. The scale of these applications naturally leads to the use of first-order methods. However, the nonconvexities and nonconcavities present in these problems, prevents the application of typical Gradient Descent-Ascent, which is known to diverge even in bilinear problems. Recently, it was shown that the Proximal Point Method (PPM) converges linearly for a family of nonconvex-nonconcave problems. In this paper, we study the convergence of a damped version of Extra-Gradient Method (EGM) which avoids potentially costly proximal computations, only relying on gradient evaluation. We show that EGM converges linearly for smooth minimax optimization problem satisfying the same nonconvex-nonconcave condition needed by PPM.
更多查看译文
关键词
extragradient methods,nonconvex–nonconcave,linear convergence
AI 理解论文
溯源树
样例
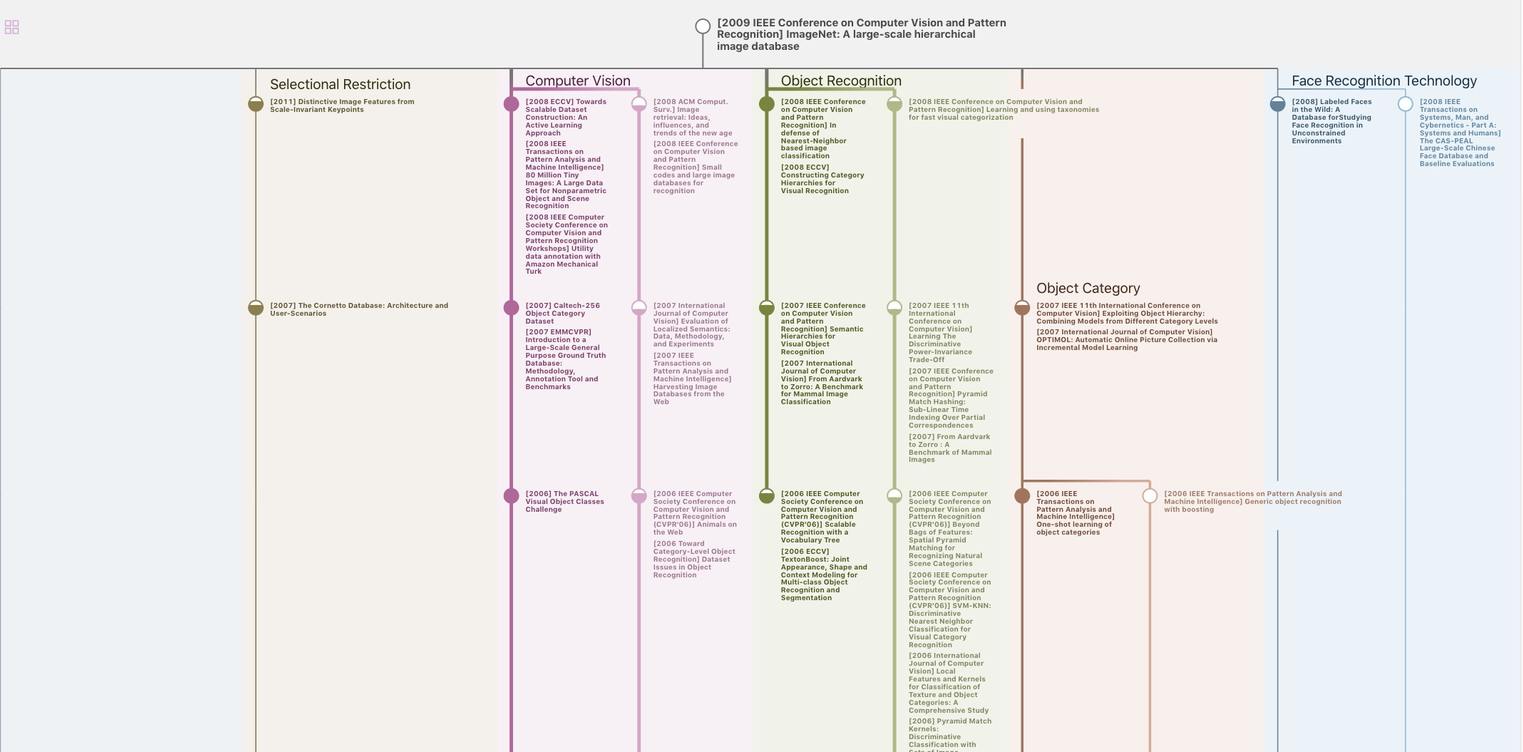
生成溯源树,研究论文发展脉络
Chat Paper
正在生成论文摘要