Double Retrieval and Ranking for Accurate Question Answering
arxiv(2022)
摘要
Recent work has shown that an answer verification step introduced in Transformer-based answer selection models can significantly improve the state of the art in Question Answering. This step is performed by aggregating the embeddings of top $k$ answer candidates to support the verification of a target answer. Although the approach is intuitive and sound still shows two limitations: (i) the supporting candidates are ranked only according to the relevancy with the question and not with the answer, and (ii) the support provided by the other answer candidates is suboptimal as these are retrieved independently of the target answer. In this paper, we address both drawbacks by proposing (i) a double reranking model, which, for each target answer, selects the best support; and (ii) a second neural retrieval stage designed to encode question and answer pair as the query, which finds more specific verification information. The results on three well-known datasets for AS2 show consistent and significant improvement of the state of the art.
更多查看译文
关键词
accurate question answering,retrieval,ranking
AI 理解论文
溯源树
样例
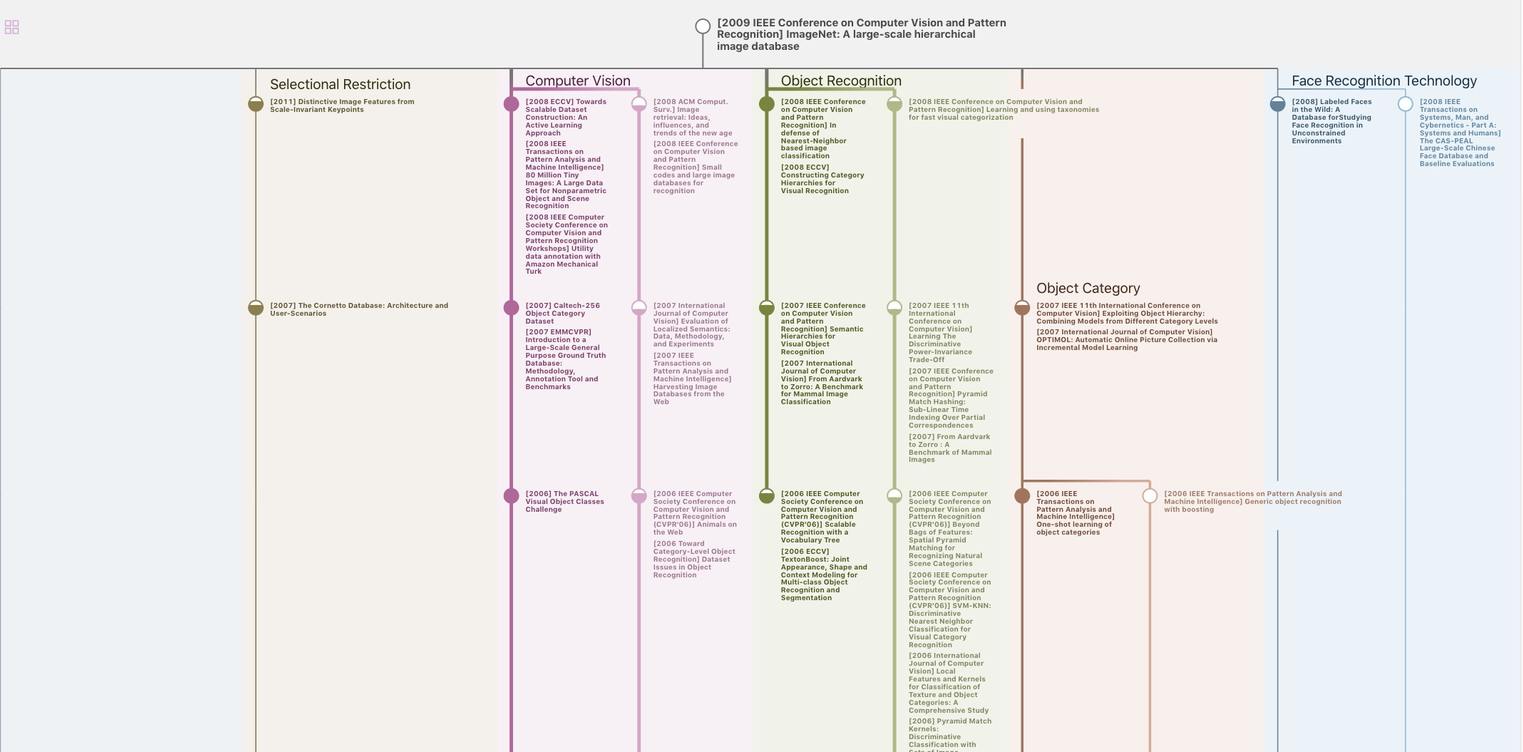
生成溯源树,研究论文发展脉络
Chat Paper
正在生成论文摘要