Unobserved Local Structures Make Compositional Generalization Hard
emnlp 2022(2022)
摘要
While recent work has convincingly showed that sequence-to-sequence models struggle to generalize to new compositions (termed compositional generalization), little is known on what makes compositional generalization hard on a particular test instance. In this work, we investigate what are the factors that make generalization to certain test instances challenging. We first substantiate that indeed some examples are more difficult than others by showing that different models consistently fail or succeed on the same test instances. Then, we propose a criterion for the difficulty of an example: a test instance is hard if it contains a local structure that was not observed at training time. We formulate a simple decision rule based on this criterion and empirically show it predicts instance-level generalization well across 5 different semantic parsing datasets, substantially better than alternative decision rules. Last, we show local structures can be leveraged for creating difficult adversarial compositional splits and also to improve compositional generalization under limited training budgets by strategically selecting examples for the training set.
更多查看译文
关键词
compositional generalization hard,structures
AI 理解论文
溯源树
样例
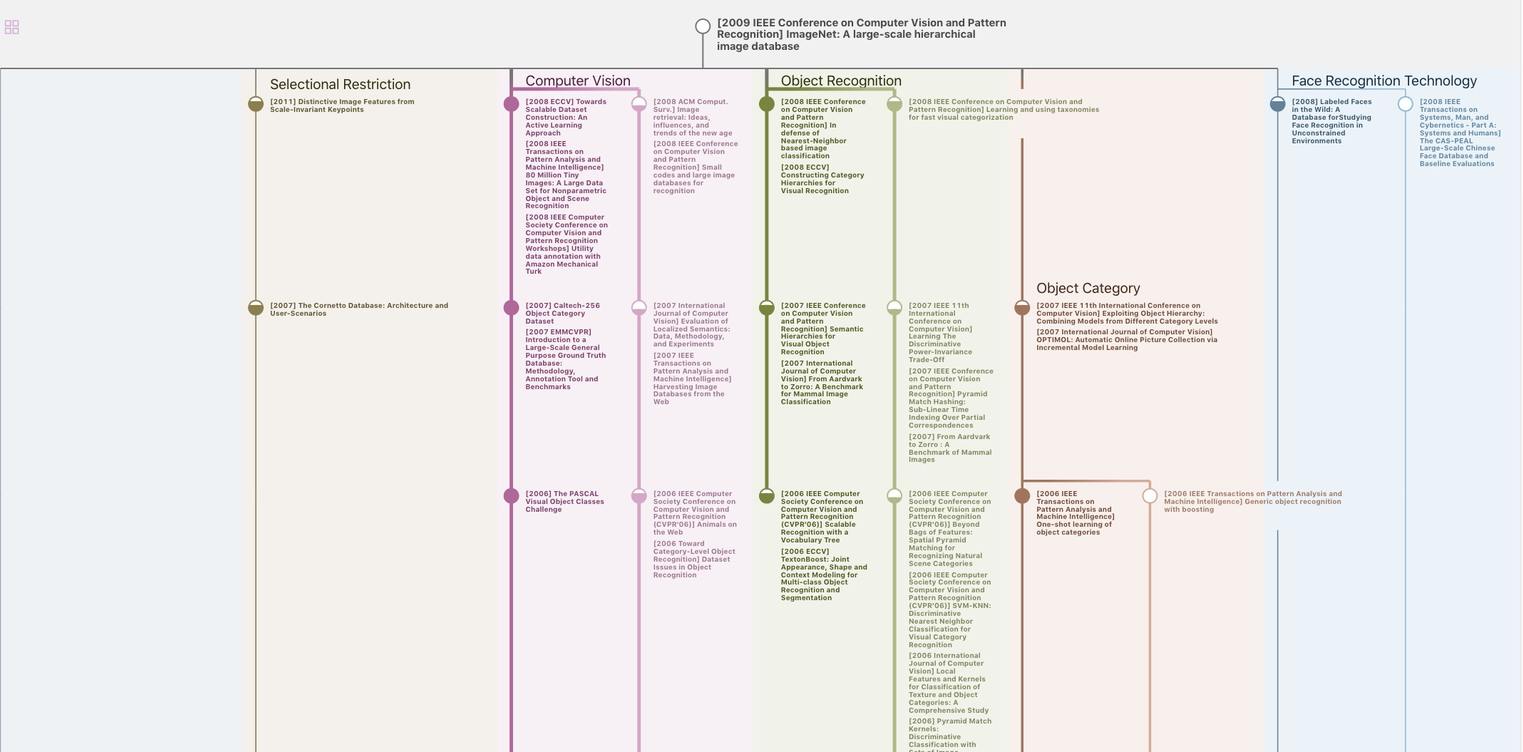
生成溯源树,研究论文发展脉络
Chat Paper
正在生成论文摘要