Domain Adaptation via Bidirectional Cross-Attention Transformer
arxiv(2022)
摘要
Domain Adaptation (DA) aims to leverage the knowledge learned from a source domain with ample labeled data to a target domain with unlabeled data only. Most existing studies on DA contribute to learning domain-invariant feature representations for both domains by minimizing the domain gap based on convolution-based neural networks. Recently, vision transformers significantly improved performance in multiple vision tasks. Built on vision transformers, in this paper we propose a Bidirectional Cross-Attention Transformer (BCAT) for DA with the aim to improve the performance. In the proposed BCAT, the attention mechanism can extract implicit source and target mixup feature representations to narrow the domain discrepancy. Specifically, in BCAT, we design a weight-sharing quadruple-branch transformer with a bidirectional cross-attention mechanism to learn domain-invariant feature representations. Extensive experiments demonstrate that the proposed BCAT model achieves superior performance on four benchmark datasets over existing state-of-the-art DA methods that are based on convolutions or transformers.
更多查看译文
关键词
domain adaptation,transformer,cross-attention
AI 理解论文
溯源树
样例
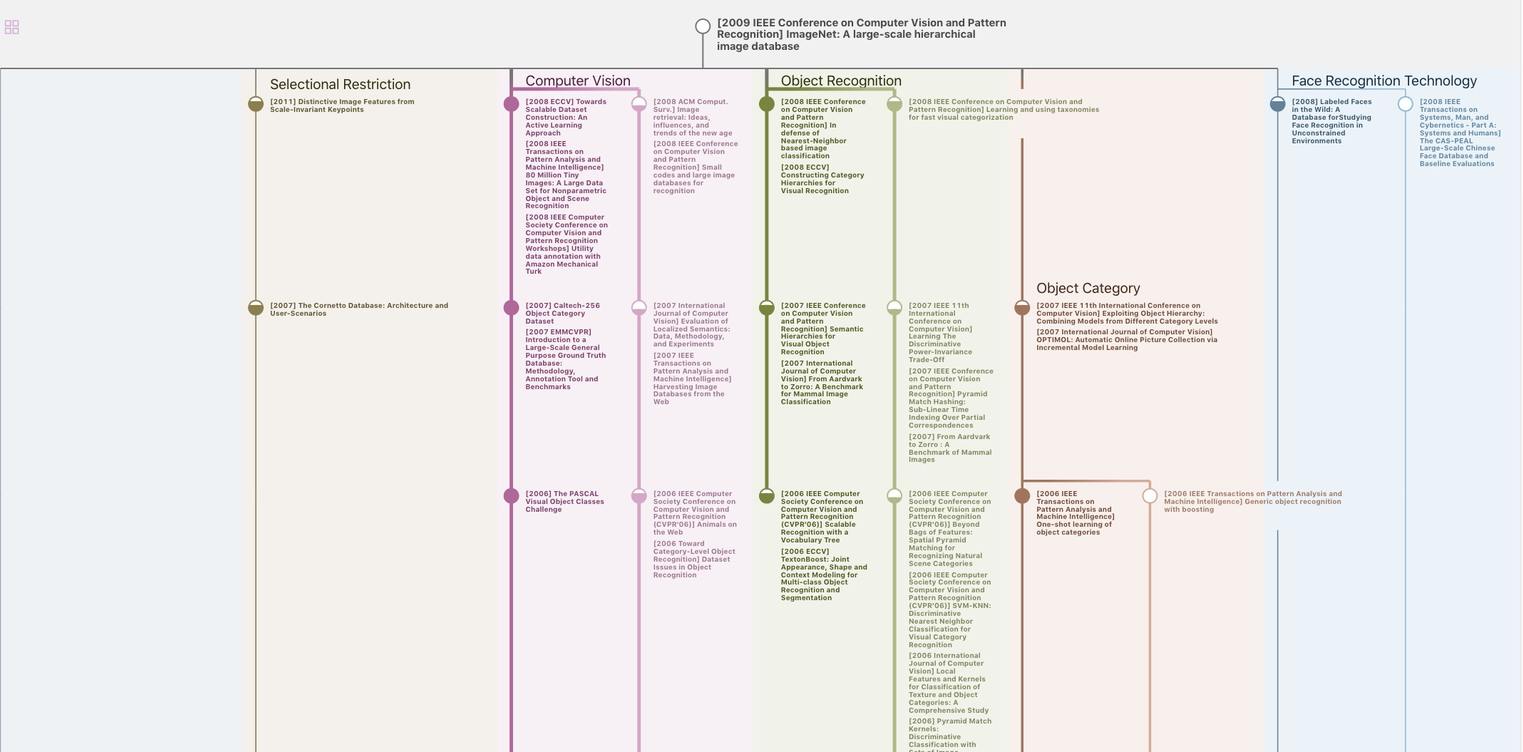
生成溯源树,研究论文发展脉络
Chat Paper
正在生成论文摘要