Learning Temporally and Semantically Consistent Unpaired Video-to-Video Translation through Pseudo-Supervision from Synthetic Optical Flow.
AAAI Conference on Artificial Intelligence(2022)
摘要
Unpaired video-to-video translation aims to translate videos between a source and a target domain without the need of paired training data, making it more feasible for real applications. Unfortunately, the translated videos generally suffer from temporal and semantic inconsistency. To address this, many existing works adopt spatiotemporal consistency constraints incorporating temporal information based on motion estimation. However, the inaccuracies in the estimation of motion deteriorate the quality of the guidance towards spatiotemporal consistency, which leads to unstable translation. In this work, we propose a novel paradigm that regularizes the spatiotemporal consistency by synthesizing motions in input videos with the generated optical flow instead of estimating them. Therefore, the synthetic motion can be applied in the regularization paradigm to keep motions consistent across domains without the risk of errors in motion estimation. Thereafter, we utilize our unsupervised recycle and unsupervised spatial loss, guided by the pseudo-supervision provided by the synthetic optical flow, to accurately enforce spatiotemporal consistency in both domains. Experiments show that our method is versatile in various scenarios and achieves state-of-the-art performance in generating temporally and semantically consistent videos. Code is available at: https://github.com/wangkaihong/Unsup_Recycle_GAN/.
更多查看译文
关键词
Computer Vision (CV)
AI 理解论文
溯源树
样例
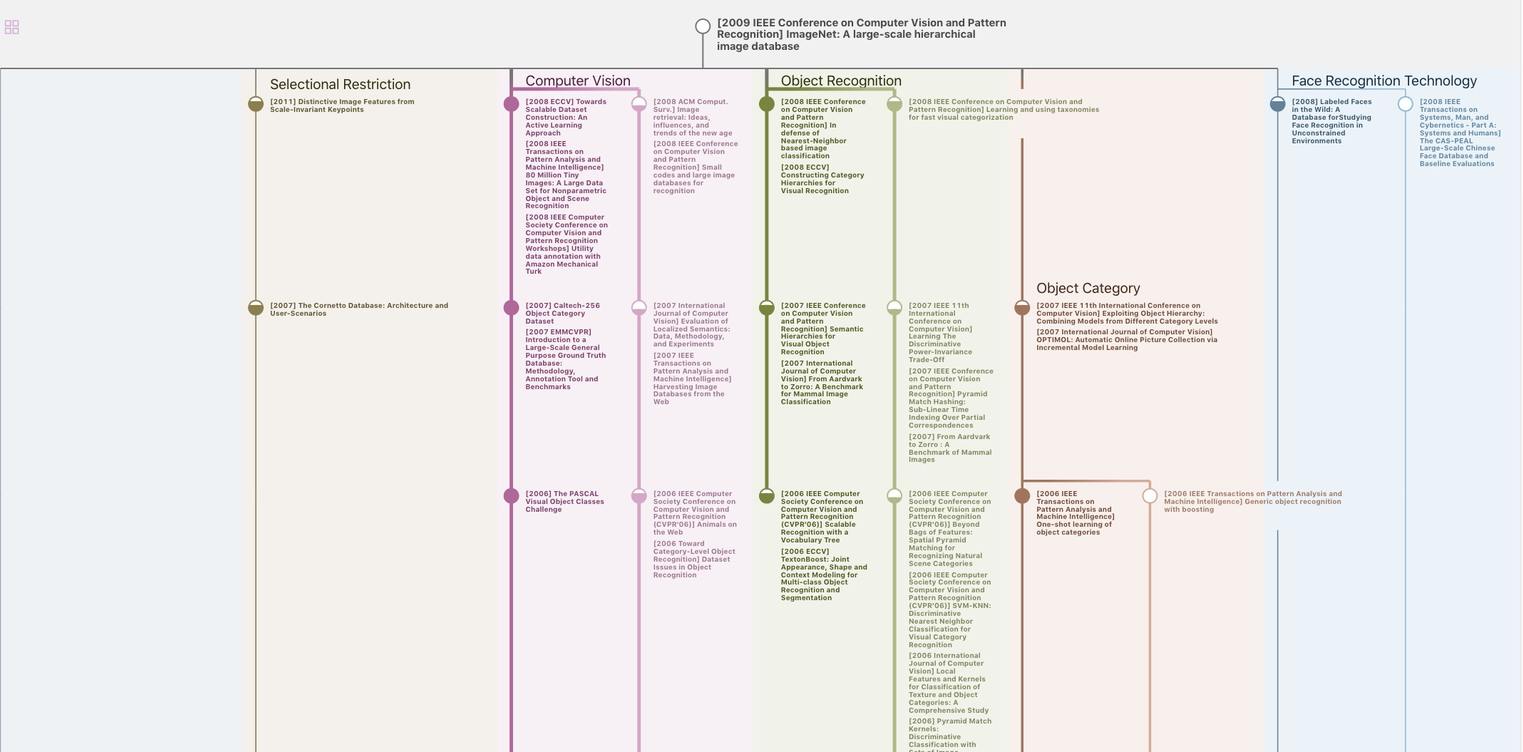
生成溯源树,研究论文发展脉络
Chat Paper
正在生成论文摘要