Improving VAE based molecular representations for compound property prediction
JOURNAL OF CHEMINFORMATICS(2022)
摘要
Collecting labeled data for many important tasks in chemoinformatics is time consuming and requires expensive experiments. In recent years, machine learning has been used to learn rich representations of molecules using large scale unlabeled molecular datasets and transfer the knowledge to solve the more challenging tasks with limited datasets. Variational autoencoders are one of the tools that have been proposed to perform the transfer for both chemical property prediction and molecular generation tasks. In this work we propose a simple method to improve chemical property prediction performance of machine learning models by incorporating additional information on correlated molecular descriptors in the representations learned by variational autoencoders. We verify the method on three property prediction tasks. We explore the impact of the number of incorporated descriptors, correlation between the descriptors and the target properties, sizes of the datasets etc. Finally, we show the relation between the performance of property prediction models and the distance between property prediction dataset and the larger unlabeled dataset in the representation space.
更多查看译文
关键词
Variational autoencoders, Vector representation, Transfer learning, Property prediction
AI 理解论文
溯源树
样例
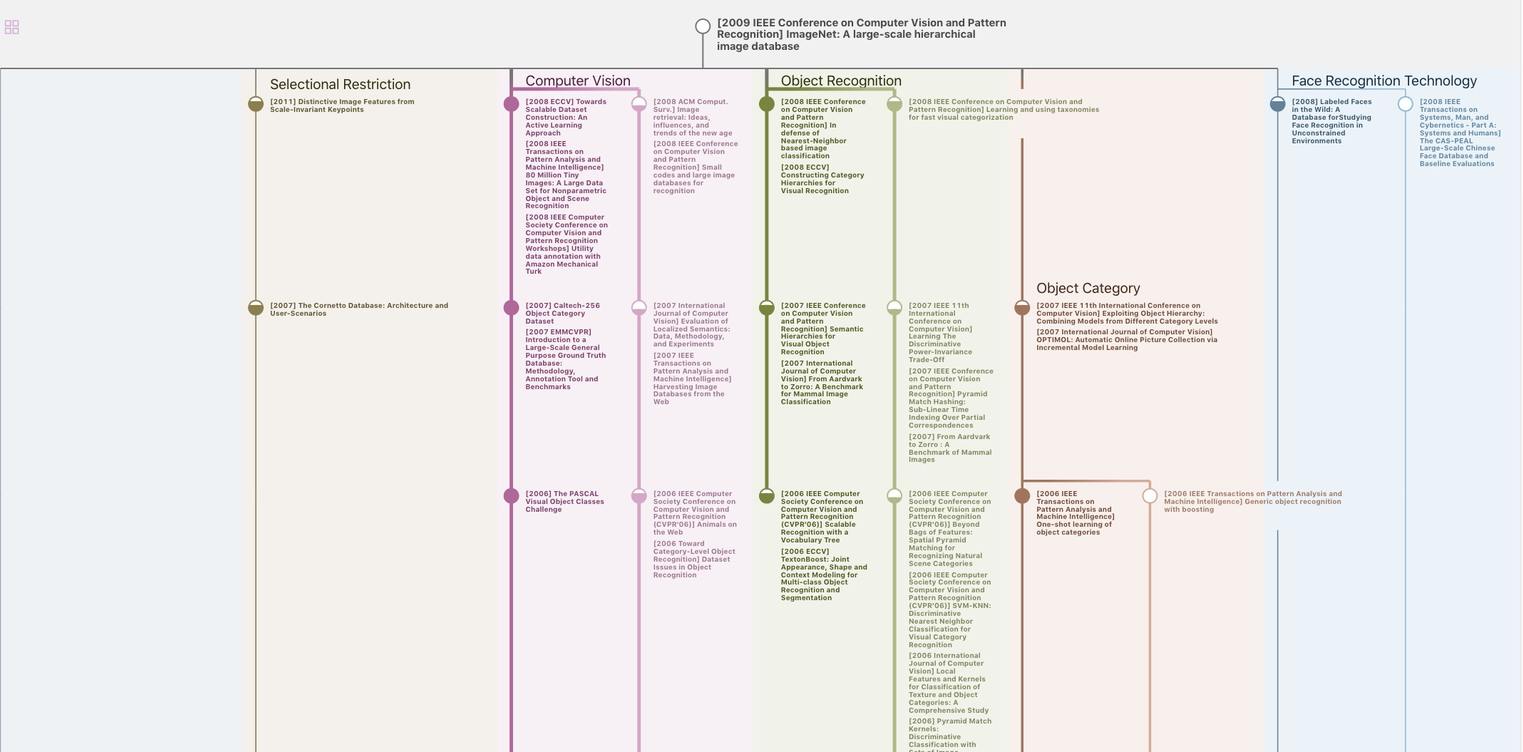
生成溯源树,研究论文发展脉络
Chat Paper
正在生成论文摘要