Experimental Design Networks: A Paradigm for Serving Heterogeneous Learners Under Networking Constraints
IEEE Conference on Computer Communications (INFOCOM)(2023)
摘要
Significant advances in edge computing capabilities enable learning to occur at geographically diverse locations. In general, the training data needed in those learning tasks are not only heterogeneous but also not fully generated locally. In this paper, we propose an experimental design network paradigm, wherein learner nodes train possibly different Bayesian linear regression models via consuming data streams generated by data source nodes over a network. We formulate this problem as a social welfare optimization problem in which the global objective is defined as the sum of experimental design objectives of individual learners, and the decision variables are the data transmission strategies subject to network constraints. We first show that, assuming Poisson data streams in steady state, the global objective is a continuous DR-submodular function. We then propose a Frank-Wolfe type algorithm that outputs a solution within a
$1-1/e$
factor from the optimal. Our algorithm contains a novel gradient estimation component which is carefully designed based on Poisson tail bounds and sampling. Finally, we complement our theoretical findings through extensive experiments. Our numerical evaluation shows that the proposed algorithm outperforms several baseline algorithms both in maximizing the global objective and in the quality of the trained models.
更多查看译文
关键词
Linear regression,Task analysis,Optimization,Bayes methods,Data models,Training,Network topology,Experimental design,DR-submodularity,Bayesian linear regression
AI 理解论文
溯源树
样例
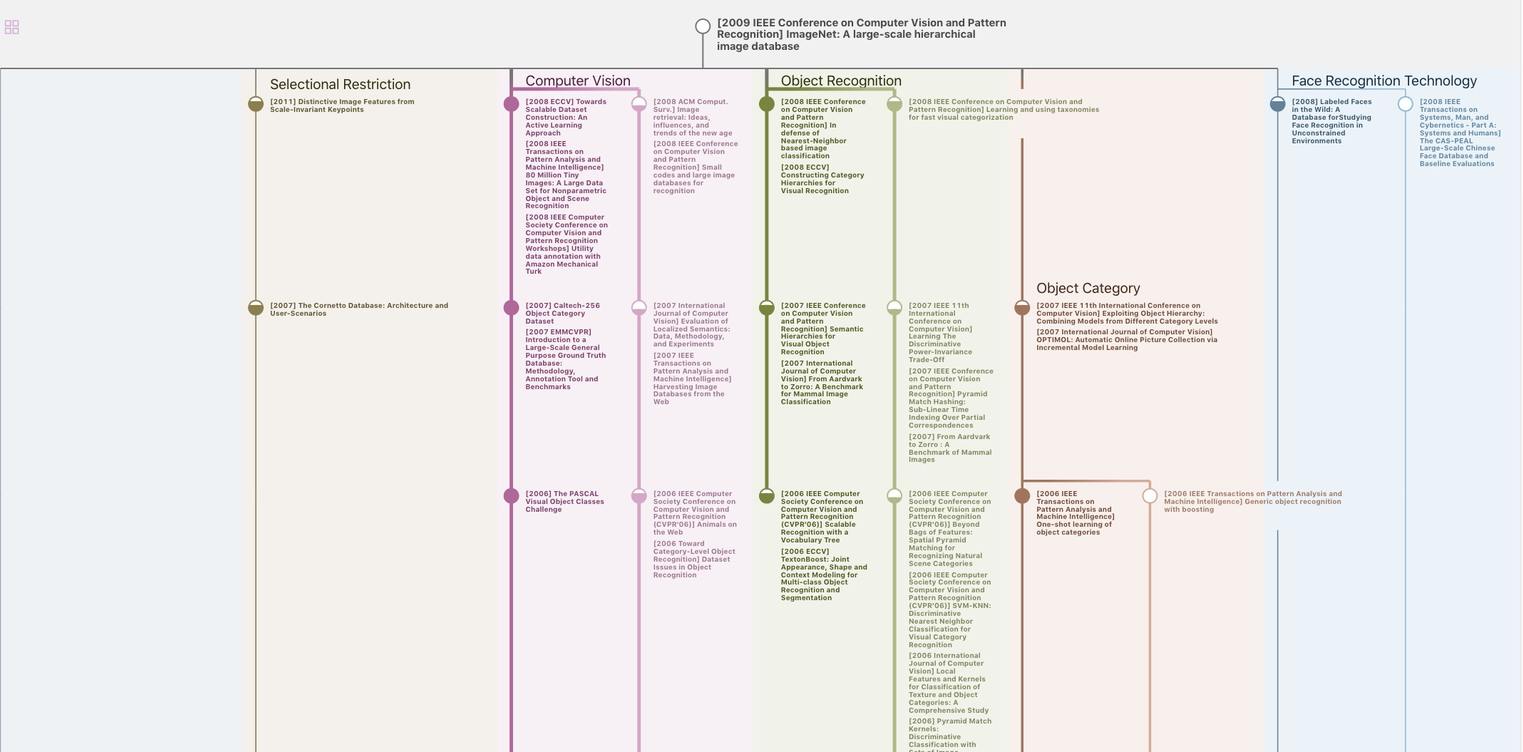
生成溯源树,研究论文发展脉络
Chat Paper
正在生成论文摘要